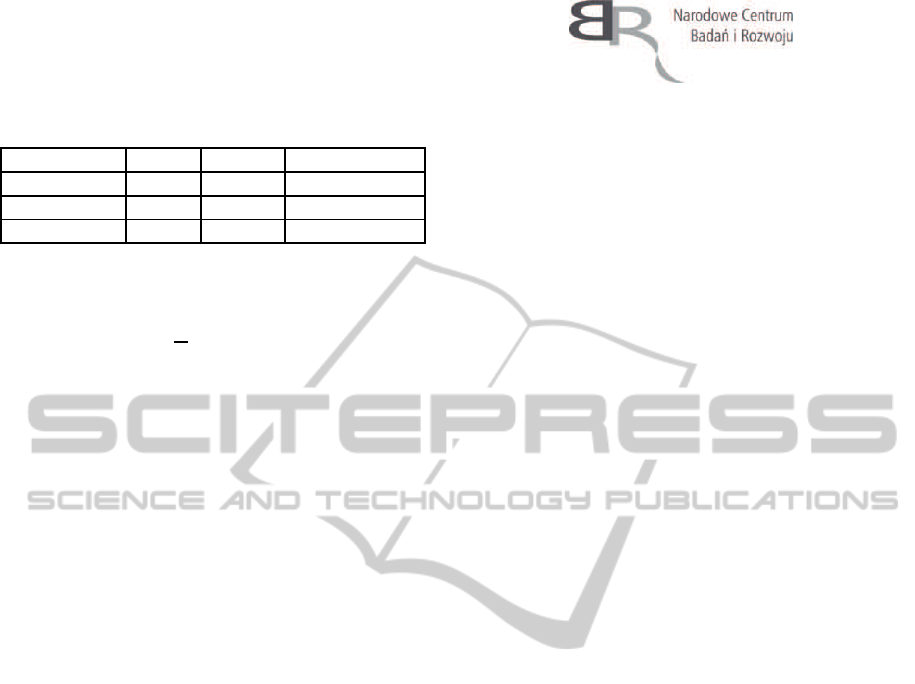
Table 4: Mean, standard deviation and 90% confidence in-
tervals of recognition rate for two system variants - system
with no modifications and system with disturbance mod-
els, arbitrary transition directions, no penalties and no si-
lence models. We also present statistics for differences be-
tween recognition rate in each bootstrap sample in the Im-
prov. row.
Rec. rate Mean St. dev. Conf. interv.
Unmod. sys. 80.3% 0.92% (78.7 - 81.8)%
Enh. sys. 81.1% 0.92% (79.5 - 82.6)%
Improv. 0.8% 0.52% (-0.1 - 1.7)%
improvement (poi) measure, defined by
poi =
1
B
B
∑
b=1
Θ(∆RR
b
) , (1)
where B is the number of bootstrap samples, Θ() is
the Heaviside function and ∆RR
b
is the recognition
rate difference between enhanced system and unmod-
ified system for b’th bootstrap sample. This measure
shows the percentage of bootstrap samples in which
recognition rate has been improved in the enhanced
system. In our case, poi amounted to 93.95%, leading
to conclusion that system is improved by introduction
of disturbance models. More details on this measure
may be found in (Bisani and Ney, 2004).
4 CONCLUSIONS
Our approach of handling acoustic disturbances, such
as breaths or filled pauses, improves the resuts of
spontaneous telephonic speech recognition. In or-
der to achieve the improvement, arbitrary transitions
between disturbance models are preferred and inser-
tion of silence models inside a phrase is discour-
aged. The method is useful in ASR systems, which
incorporate Hidden Markov Models in the recogni-
tion task, serving as an extension to the existing sys-
tem. Application of the method can be found in any
scenario, where ASR system has to deal with spon-
taneous speech, an example of such scenario being
recognition of user commands in IVR system menu.
ACKNOWLEDGEMENTS
This work was supported by LIDER/37/69/L-3/11/
NCBR/2012 grant. We thank Magdalena Igras for as-
sistance in gathering recordings and transcriptions of
filled pauses and breaths and Jakub Gałka for advices
regarding statistical analysis of our results.
REFERENCES
Audhkhasi, K., Kandhway, K., Deshmukh, O., and Verma,
A. (2009). Formant-based technique for automatic
filled-pause detection in spontaneous spoken english.
In Acoustics, Speech and Signal Processing, 2009.
ICASSP 2009. IEEE International Conference on,
pages 4857–4860.
Barczewska, K. and Igras, M. (2012). Detection of disfluen-
cies in speech signal. In Young scientists towards the
challenges of modern technology: 7th international
PhD students and young scientists conference in War-
saw.
Bisani, M. and Ney, H. (2004). Bootstrap estimates for
confidence intervals in asr performance evaluation. In
Acoustics, Speech, and Signal Processing, 2004. Pro-
ceedings. (ICASSP ’04). IEEE International Confer-
ence on, volume 1, pages I–409–12 vol.1.
Boakye, K. and Stolcke, A. (2006). Improved speech activ-
ity detection using cross-channel features for recog-
nition of multiparty meetings. In Proc. of INTER-
SPEECH, pages 1962–1965.
Gollan, C., Bisani, M., Kanthak, S., Schluter, R., and Ney,
H. (2005). Cross domain automatic transcription on
the tc-star epps corpus. In Acoustics, Speech, and
Signal Processing, 2005. Proceedings. (ICASSP ’05).
IEEE International Conference on, volume 1, pages
825–828.
Goto, M., Itou, K., and Hayamizu, S. (1999). A real-time
filled pause detection system for spontaneous speech
recognition. In Proc. of Eurospeech, pages 227–230.
Igras, M. and Zi´ołko, B. (2013a). Modelowanie i detekcja
oddechu w sygnale akustycznym. In Proc. of Mode-
lowanie i Pomiary w Medycynie.
Igras, M. and Zi´ołko, B. (2013b). Wavelet method for
breath detection in audio signals. In Multimedia and
Expo (ICME), 2013 IEEE International Conference
on, pages 1–6.
Konturek, S. (2007). Fizjologia człowieka. Podrecznik dla
student´ow medycyny. Elsevier Urban & Partner.
Marciniak, M., editor (2010). Anotowany korpus dialog´ow
telefonicznych. Akademicka Oficyna Wydawnicza
EXIT, Warsaw.
Ratan, V. (1993). Handbook of Human Physiology. Jaypee.
Stouten, F. and Martens, J. (2003). A feature-based filled
pause detection technique for dutch. In IEEE Intl
Workshop on ASRU, pages 309–314.
Zi´ołko, M., Gałka, J., Zi´ołko, B., Jadczyk, T., Skurzok, D.,
and Ma¸sior, M. (2011). Automatic speech recogni-
tion system dedicated for Polish. Proceedings of In-
terspeech, Florence.
SIGMAP2014-InternationalConferenceonSignalProcessingandMultimediaApplications
260