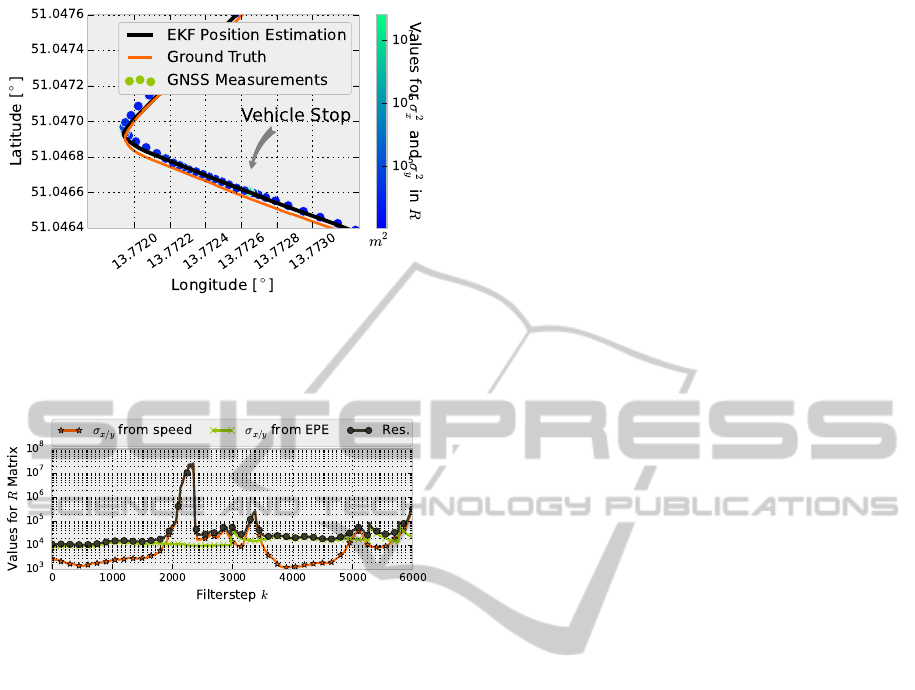
Figure 11: Detailed view of the stop within the performed
test drive with raised uncertainty for R. (GNSS measure-
ment dots are reduced to every 10th for better visualisation
in this figure).
Figure 12: Values for adaptive σ
v
, σ
EPE
and resulting σ for
x and y.
The values for the measurement uncertainties in R
are calculated like shown in figure 12.
7 CONCLUSIONS
In this paper we presented a novel approach to adap-
tively calculate the measurement uncertainty for an
improved position and attitude estimation, based on
the Estimated Position Error and the speed, deter-
mined by the low cost GNSS receiver. We explained
the basics and used some empirically chosen values
to parametrize the filter. With that, we showed with
simulated data, that the filter performes significantly
better than a standard Extended Kalman Filter. Based
on that, we conducted test drives and used the devel-
oped Adaptive EKF for a real world dataset, which
proved its ability to improve the position estimation
with partly bad signal quality. In addition to that, the
filter also performs pretty well on dynamic situations
and is not loosing the ability to follow dynamic vehi-
cle movements. The filter cannot be used to get rid
of bias in position estimation, because the EPE from
GNSS has, by definition, no information about static
drift of the position information. The presented fil-
ter can be used to get significantly better results while
standing still or driving slowly as well as keeping the
heading fixed while do so. Additionally, the attitude
of the vehicle is estimated, based on the filter pre-
sented in (Madgwick, 2010).
ACKNOWLEDGEMENTS
The authors would like to thank the Free State of Sax-
ony and the European Union, which funded this re-
search from the ESF fond.
REFERENCES
Barczyk, M. and Lynch, A. F. (2011). Invariant Extended
Kalman Filter design for a magnetometer-plus-GPS
aided inertial navigation system. IEEE Conference on
Decision and Control and European Control Confer-
ence, pages 5389–5394.
Bistrovs, V. and Kluga, A. (2012). Adaptive Extended
Kalman Filter for Aided Inertial Navigation System.
Electronics & Electrical Engineering, 6(6).
Buchholz, J. J. (2013). Vorlesungsmanuskript Regelung-
stechnik und Flugregler.
Effertz, J. (2009). Autonome Fahrzeugf¨uhrung in urbaner
Umgebung durch Kombination objekt- und karten-
basierter Umfeldmodelle. PhD thesis, Technische
Universit¨at Carolo-Wilhelmina zu Braunschweig.
Kalman, R. E. (1960). A New Approach to Linear Filtering
and Prediction Problems. 82(Series D):35–45.
Kelly, A. (1994). A 3D state space formulation of a naviga-
tion Kalman filter for autonomous vehicles. (May).
Kingston, D. and Beard, R. (2004). Real-Time Attitude and
Position Estimation for Small UAVs Using Low-Cost
Sensors. AIAA 3rd ”Unmanned Unlimited” Technical
Conference, Workshop and Exhibit, pages 1–9.
Madgwick, S. (2010). An efficient orientation filter for in-
ertial and inertial/magnetic sensor arrays. Report x-io
and University of Bristol.
Mourikis, A. and Roumeliotis, S. (2007). A Multi-State
Constraint Kalman Filter for Vision-aided Inertial
Navigation. Proceedings 2007 IEEE International
Conference on Robotics and Automation.
Penarrocha, I. and Sanchis, R. (2010). Adaptive extended
Kalman filter for recursive identification under miss-
ing data. 49th IEEE Conference on Decision and Con-
trol (CDC), pages 1165–1170.
Schubert, R., Adam, C., Obst, M., Mattern, N., Leonhardt,
V., and Wanielik, G. (2011). Empirical evaluation of
vehicular models for ego motion estimation. 2011
IEEE Intelligent Vehicles Symposium (IV), pages 534–
539.
Sharif, M. and Stein, A. (2004). Integrated ap-
proach to predict confidence of GPS measurement.
isprsserv.ifp.uni-stuttgart.de.
EPEandSpeedAdaptiveExtendedKalmanFilterforVehiclePositionandAttitudeEstimationwithLowCostGNSSand
IMUSensors
655