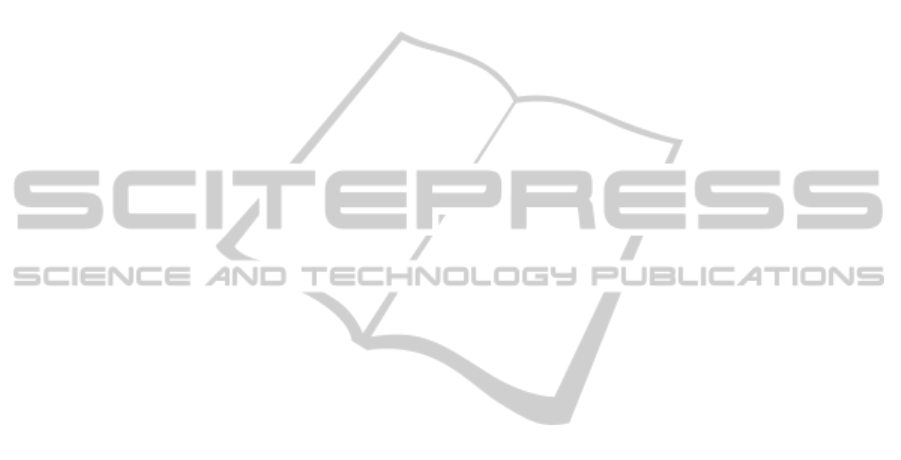
fitted model for European grain market from AD
1500 to 1800. The results are shown in Table 1.
Based on the modelling results, a cold phase
would raise grain price through lowering the supply
in the grain market, while the mild climate would be
favourable to agrarian economy.
In the meantime, the population is acting as role
of farmer in the study period. The simulation results
do not imply that population in pre-industrial
Western Europe did not act as consumer at all in the
past. However, the role of producer exceeds the role
of consumer in the long term.
4 DISUCUSSION
4.1 Annual Impact of Climate Change
First, the impact of climate on the grain market
virtually exists. The statistical model results are
consistent with the literature survey. The hypothesis
of study is not only theoretically sound, but also
quantitatively verified.
Second, the fluctuation rate in temperature will
be enlarged when it impacts on the grain market,
based on the modelling. The larger the temperature
changed, the larger price change would be,
according to the pattern of ARX modelling results.
Besides, based on the modelling results, the
interaction between climate change and grain market
is not a linear process, though it is due to the
modelling design in the research. However, as
pointed out, the process of climate change can be
non-linear (Schneider, 2004), and its corresponding
effect on the socioeconomic system can also reflect
non-linear patterns (Adger et al, 2009). Therefore,
following the current research on climate change
issues, price and temperature can be considered
exponential functions according to our statistical
results as well as to our studies.
4.2 Long Term Impact of Climate
Change
In order to examine the long term impact of
temperature parameter, the time series theory should
be reviewed. Generally, the ARX model can also be
written by the formula transformation, which is
listed in the Section of “Equations”.
Based on the result, the impact of climate change
from year t will be lasting long in the following year.
Furthermore, attenuation speeds of obvious climatic
impact last 10 years according to Figure 2. After 10
year, the impact is almost equal to zero. The
attenuation speeds of temperature impact show the
buffering capacity of human society to relieve the
climatic impact gradually though still exists for 10
years. This result justified again that the pre-
industrial Western Europe could try to make the
adaptation and relief to the climate change, while
with limited effectiveness. However, compared to
whole Europe of 25 years lasting effect (Pei et al,
2013), the higher population density makes Western
Europe is more vulnerable to climate change.
Due to the low speed of attenuation, cooling
impact could pile up, especially during the long term
cooling period. In the short-term (several years),
those social buffers are effective in stabilizing grain
prices. However, institutional and social buffering
mechanisms would be ultimately exhausted by the
recurrent subsistence crises caused by long term-
cooling (Orlove, 2005). Worldwide empirical studies
also have revealed that in the face of persistent
agricultural shortages induced by long-term cooling,
social buffers ultimately became ineffective and
were unable to prevent social-economic crisis (Lee
et al, 2008; Pei et al, 2014; Zhang et al, 2007).
Therefore, the long lasting climate change could be
more disastrous to society than short-term climate
variations.
Lastly, in addition to above theoretical
implications of a specific field, the simulation in the
study proves that ARX modelling is a feasible
choice in the field of historical research.
5 CONCLUSIONS
Climate change has played a very important role in
Western European agrarian economy in the pre-
industrial era. The current study first time adopts
ARX modelling to scrutinize climate-economy
association in pre-industrial Western Europe AD
1500-1800. This study fills the gap in previous
quantitative analyses about the short- and long-term
effect of climate change on past agrarian economies.
Through the statistical analysis, temperature is
important to economy of pre-industrial Western
Europe at a large spatial scale. In the short term,
cooling climate could cause high prices because of
poor production and scarcity in the grain market.
The larger the changes in temperature, the larger the
price changes are, which shows the non-linear
interaction between climate and economy in the
past. In the long term, the impact from climate
change could last around 10 year, which reflects the
social buffering capacity. The long term climatic
impact, especially 10 year or even longer could pile
PriceResponsesofGrainMarketunderClimateChangeinPre-industrialWesternEuropebyARXModelling
813