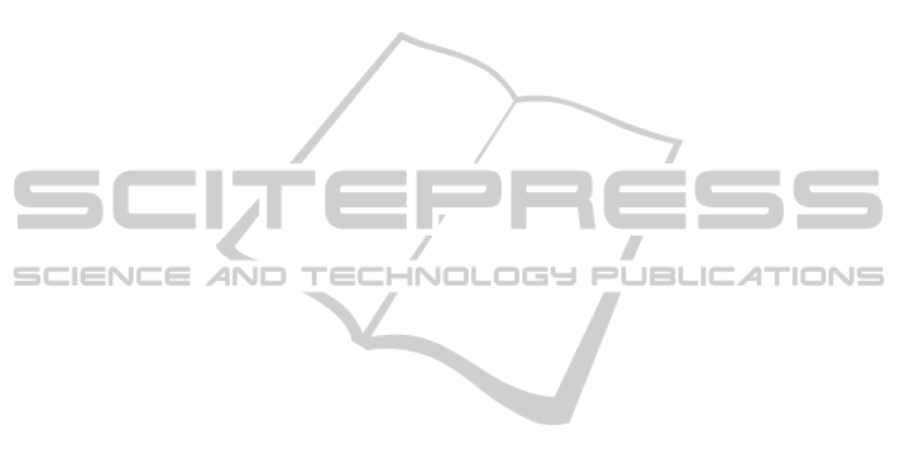
2.2 Transactive Memory System at the
Cluster Level
A Transactive Memory System (TMS) is a shared
system that people in closed relationships develop
for encoding, storing, and retrieving information
about different substantive domains (Wegner, 1987;
Ren and Argote, 2011). The basic idea is that
individual knowledge in a group consists of internal
knowledge (held in his mind) and external
knowledge (which the individual can access using
the TMS) (Jackson and Klobas, 2008). As such,
TMS supposes that individuals play the role of
external memory for other individuals who in turn
encode meta-memories (i.e., the label or subject of
the knowledge as well as its location, but not the
knowledge itself) (Nevo and Wand, 2005).
TMS at the group level
Originally, researches on TMS were developed
at dyad or team group level of analysis. TMS
implies a cooperative division of learning,
remembering and communicating knowledge within
the group (Wegner, 1987). Over time, knowledge in
TMS becomes more specialized or differentiated
among members as a result of the division of
learning; at the same time, shared or integrated
knowledge increases as individuals develop a shared
cognitive representations of “who knows what”
(Brandon and Hollingshead, 2004; Ren and Argote,
2011).
As a result, three components are crucial to
TMS: cognitive interdependence, expertise related to
task and people, and shared mental models (Brandon
and Hollingshead, 2004). First, cognitive
interdependence describes the extent to which team
member’s work outcomes depend on a combination
of their own input and the input of others members.
As such, it motivates and sustains the development
of TMS. Task interdependence led thus to a higher
level of TMS, which in turn led to improved team
performance (Ren and Argote, 2011). Second,
Brandon and Hollingshead (2004) expand the basic
notion of labels and location (who knows what) into
a more explicit portrait of relations between Task,
Expertise and People (TEP). Third, shared mental
model concern not only a shared representation of
“who knows what” or “TPE” units, but also macro-
organizations of those “TPE” units. These shared
mental models have implications for the
effectiveness of the TMS. Brandon and
Hollingshead (2004) propose to evaluate their
development along three dimensions: accuracy,
sharedness and validation.
TMS at the organizational level
Only four studies have recently extended the
TMS concept to the organizational level (Ren and
Argote, 2011), including one case study (Jackson
and Klobas, 2008). Generalizing TMS to the
organizational level raises several challenges (Ren
and Argote, 2011). First, members might have more
trouble identifying who knows what in large
organizations than in small groups. Second,
organizations are composed by multiple subgroups
increasingly geographically distributed with less
communication and knowledge sharing across these
subgroups. Finally, when tacit knowledge is
available in a distal part of organization, retrieval
becomes difficult. Because organizations are larger
than work groups and geographically distributed,
Nevo and Wand (2005) shows that TMS might rely
upon advanced technology to locate and shared
information. They suggest that a general directory of
meta-memories should be formed, linking the
different communities and supporting knowledge
transfer between individuals in different
communities. In this case, knowledge transfer is not
provided through repositories but rather through
technology mediated connections.
Extending TMS to a large group requires the use
of artificial directories based on formalized meta-
knowledge integrating three main dimensions (Nevo
and Wand, 2005): conceptual, descriptive and
persuasive. In fact, a set of concepts is needed to
describe the subject of knowledge (ontology can be
used here). Descriptive knowledge can be
formalized to describe the author of knowledge
(location) and to characterize the knowledge (date,
format…). At the persuasive level, source of
credibility and perceptions of expertise should be
formalized. In this line, Jackson and Klobas (2008)
add two insights: in an organizational TMS people
access each other’s knowledge through a
combination of personal and codified directory
system; maintaining these directories which can be
activated for retrieving knowledge when it’s needed
(passive allocation) is more efficient than storing the
knowledge and sending content trough a system
network (repositories and active allocation).
Finally, TMS at the organizational level raises
two main questions (Nevo and Wand, 2005; Jackson
and Klobas, 2008; Ren and Argote, 2011). How an
organizational TMS can be developed with the
assistance of social network and information
technologies? How manage the ability to keep the
meta-knowledge directories updated? These two
questions that remains unanswered at the
organizational level become more crucial at the
TransactiveMemorySysteminClusters-TheKnowledgeManagementPlatformExperience
7