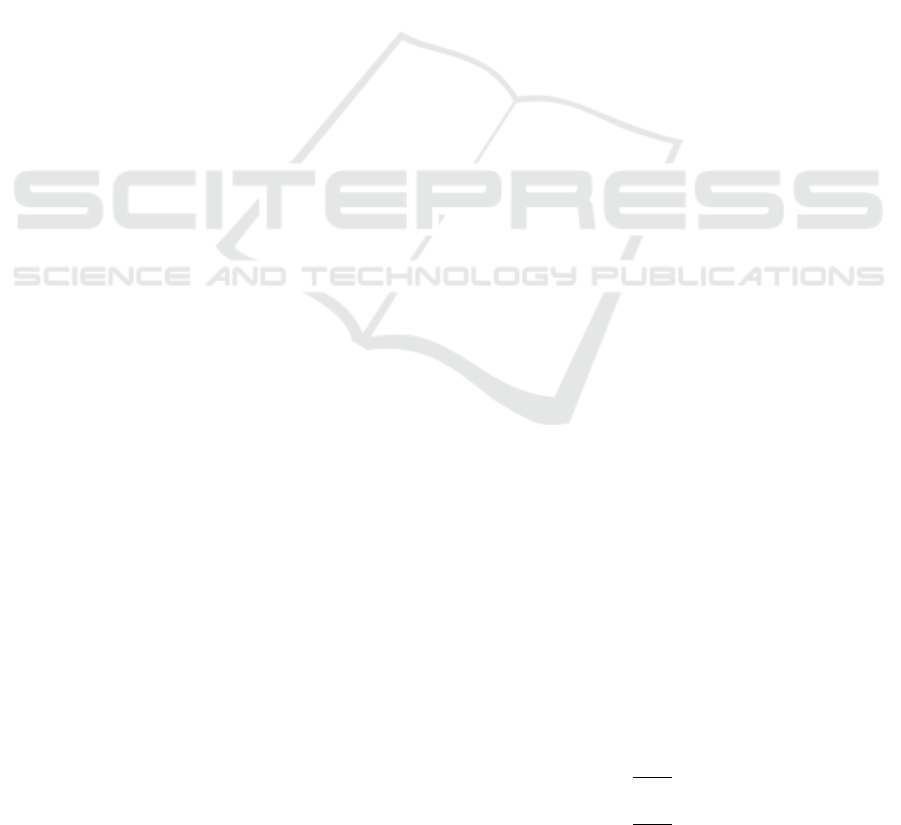
An Analytical Approach to Evaluating Bivariate Functions of
Fuzzy Numbers with One Local Extremum
Arthur Seibel and Josef Schlattmann
Workgroup on System Technologies and Engineering Design Methodology
Hamburg University of Technology, 21073 Hamburg, Germany
Keywords:
Parameter Uncertainties, Bivariate Functions, Fuzzy Numbers, Analytical Fuzzy Calculus.
Abstract:
This paper presents a novel analytical approach to evaluating continuous, bivariate functions of independent
fuzzy numbers with one local extremum. The approach is based on a parametric α-cut representation of fuzzy
numbers and allows for the inclusion of parameter uncertainties into mathematical models.
1 INTRODUCTION
There is an increasing effort in the scientific commu-
nity to provide suitable methods for the inclusion of
uncertainties into mathematical models. One way to
do so is to introduce parametric uncertainty by rep-
resenting the uncertain model parameters as fuzzy
numbers (Dubois and Prade, 1980) and evaluating the
model equations by means of Zadeh’s extension prin-
ciple (Zadeh, 1975). The evaluation of this classical
formulation of the extension principle, however, turns
out to be a highly complex task (Klimke, 2006). For-
tunately, Buckley and Qu (1990) provide an alterna-
tive formulation that operates on α-cuts and is appli-
cable to continuous functions of independent fuzzy
numbers. Powerful numerical techniques have been
developed to implement this alternative formulation
(Moens and Hanss, 2011). However, there is no gen-
eral analytical approach for a calculus with fuzzy
numbers. For this purpose, a practical analytical ap-
proach to evaluating continuous, monotonic functions
of independent fuzzy numbers was introduced by the
authors (Seibel and Schlattmann, 2013, 2014), which
is based on the alternative formulation of the exten-
sion principle. In this paper, we extend this approach
to bivariate functions of fuzzy numbers with one local
extremum and no saddle points.
An outline of this paper is as follows. In Section
2, we give a definition of fuzzy numbers and present
two important types. In Section 3, we briefly recall
Zadeh’s extension principle and introduce the alter-
native formulation based on α-cuts. In Section 4, we
describe our analytical approach and give two illustra-
tive examples. Finally, in Section 5, some conclusions
are drawn.
2 FUZZY NUMBERS
Fuzzy numbers (Dubois and Prade, 1980) are a spe-
cial class of fuzzy sets (Zadeh, 1965), which can be
defined as follows.
A normal, convex fuzzy set ˜x over the real line
R is called fuzzy number if there is exactly one ¯x ∈R
with µ
˜x
( ¯x) = 1 and the membership function is at least
piecewise continuous. The value ¯x is called the modal
or peak value of ˜x.
Theoretically, an infinite number of possible types
of fuzzy numbers can be defined. However, only few
of them are important for engineering applications
(Hanss, 2005). These typical fuzzy numbers shall be
described in the following.
2.1 Triangular Fuzzy Numbers
Due to its very simple, linear membership function,
the triangular fuzzy number (TFN) is the most fre-
quently used fuzzy number in engineering. In order
to define a TFN with the membership function
µ
˜x
(x) =
1 +
x − ¯x
τ
L
, ¯x −τ
L
≤ x ≤ ¯x,
1 −
x − ¯x
τ
R
, ¯x < x ≤ ¯x + τ
R
,
(1)
we use the parametric notation (Hanss, 2005)
˜x = tfn( ¯x, τ
L
,τ
R
),
where ¯x denotes the modal value, τ
L
denotes the left-
hand, and τ
R
denotes the right-hand spread of ˜x (cf.
Figure 1). If τ
L
= τ
R
, the TFN is called symmetric. Its
α-cuts x(α) =
x
L
(α),x
R
(α)
result from the inverse
89
Seibel A. and Schlattmann J..
An Analytical Approach to Evaluating Bivariate Functions of Fuzzy Numbers with One Local Extremum.
DOI: 10.5220/0005026500890094
In Proceedings of the International Conference on Fuzzy Computation Theory and Applications (FCTA-2014), pages 89-94
ISBN: 978-989-758-053-6
Copyright
c
2014 SCITEPRESS (Science and Technology Publications, Lda.)