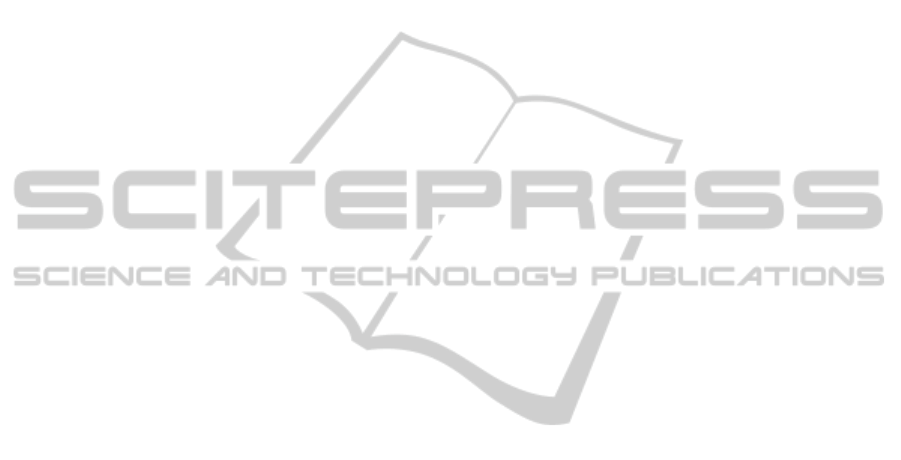
The TDNN optimised and trained with the responses
to band-limited GWN predicts the responses
accurately for unseen band-limited GWN and
sinusoidal inputs, significantly better than the AR
model in the case of GWN stimuli. Furthermore, the
TDNN trained with the average response is also able
to predict responses in different individuals,
although with limited accuracy. The accuracy of the
average response TDNN model was not statistically
different to that of the individual models, which
suggests that, in this case, the average response is a
good representation of the system. Furthermore, the
NMSE values are similar to those obtained with
Wiener methods in locusts electrophysiological
responses of tibial motor neurons (Dewhirst,
Simpson et al. 2009), which suggests that ANNs
could be a good approach to model nervous systems.
The errors in the predictions are related to the
levels of measurement noise, background
spontaneous activity and individual differences in
the responses (Schneidman, Brenner et al. 2000,
Faisal, Selen et al. 2008, Marder and Taylor 2011).
There is, however, an underlying response common
to all individuals that the TDNN is able to model
and predict accurately, but the noise and the inherent
response from each animal cannot be predicted with
a generic model.
Therefore, the TDNN model of the average
reflex response exceeds the performance of the AR
model and is a good candidate model to be
considered towards the understanding of nervous
systems and motor control. It could also be used in
the design of treatment for neuromuscular injuries,
such as drop foot. Similar reflexes could also be
applied in the design of active prosthesis or
autonomous robots.
ACKNOWLEDGEMENTS
Alicia Costalago Meruelo was supported by a
studentship from The Institute for Complex Systems
Simulation (ICSS), funded by the Engineering and
Physical Sciences Research Council (UK) and the
University of Southampton.
REFERENCES
Angeline, P. J., G. M. Saunders and J. B. Pollack (1994).
"An evolutionary algorithm that constructs recurrent
neural networks." Neural Networks, IEEE
Transactions on 5(1): 54-65.
Benardos, P. G. and G.-C. Vosniakos (2007). "Optimizing
feedforward artificial neural network architecture."
Eng. Appl. Artif. Intell. 20(3): 365-382.
Bishop, C. M. (1995). Neural networks for pattern
recognition, Oxford university press.
Burrows, M. (1996). The neurobiology of an insect brain.
Oxford University Press, Oxford ;.
Burrows, M. and G. A. Horridge (1974). "The
Organization of Inputs to Motoneurons of the Locust
Metathoracic Leg." Philosophical Transactions of the
Royal Society of London. Series B, Biological Sciences
269(896): 49-94.
Burrows, M., G. J. Laurent and L. H. Field (1988).
"Proprioceptive inputs to nonspiking local
interneurons contribute to local reflexes of a locust
hindleg." The Journal of Neuroscience 8(8): 3085-
3093.
Büschges, A. (2005). "Sensory Control and Organization
of Neural Networks Mediating Coordination of
Multisegmental Organs for Locomotion." Journal of
Neurophysiology 93(3): 1127-1135.
Bush, B. M., J. P. Vedel and F. Clarac (1978).
"Intersegmental reflex actions from a joint sensory
organ (CB) to a muscle receptor (MCO) in decapod
crustacean limbs." The Journal of Experimental
Biology 73(1): 47-63.
Carvalho, A. R., F. M. Ramos and A. A. Chaves (2011).
"Metaheuristics for the feedforward artificial neural
network (ANN) architecture optimization problem."
Neural Comput. Appl. 20(8): 1273-1284.
Dewhirst, O. P. (2013). Validation of Nonlinear System
Identification Models of the Locust Hind Limb
Control System using Natural Stimulation. PhD
Thesis, Southampton University.
Dewhirst, O. P., N. Angarita-Jaimes, D. M. Simpson, R.
Allen and P. L. Newland (2012). "A system
identification analysis of neural adaptation dynamics
and nonlinear responses in the local reflex control of
locust hind limbs." J Comput Neurosci: 1-20.
Dewhirst, O. P., D. M. Simpson, R. Allen and P. L.
Newland (2009). Neuromuscular reflex control of limb
movement - validating models of the locusts hind leg
control system using physiological input signals.
Neural Engineering, 2009. NER '09. 4th International
IEEE/EMBS Conference.
Eiben, A. E. and J. E. Smith (2003). Introduction to
Evolutionary Computing, SpringerVerlag.
Faisal, A. A., L. P. J. Selen and D. M. Wolpert (2008).
"Noise in the nervous system." Nat Rev Neurosci 9(4):
292-303.
Field, L. H. and M. Burrows (1982). "Reflex Effects of the
Femoral Chordotonal Organ Upon Leg Motor
Neurones of the Locust." Journal of Experimental
Biology 101(1): 265-285.
Field, L. H. and T. Matheson (1998). Chordotonal Organs
of Insects. Advances in Insect Physiology. P. D.
Evans, Academic Press. Volume 27: 1-228.
Field, L. H. and F. C. Rind (1981). "A single insect
chordotonal organ mediates inter- and intra-segmental
NCTA2014-InternationalConferenceonNeuralComputationTheoryandApplications
30