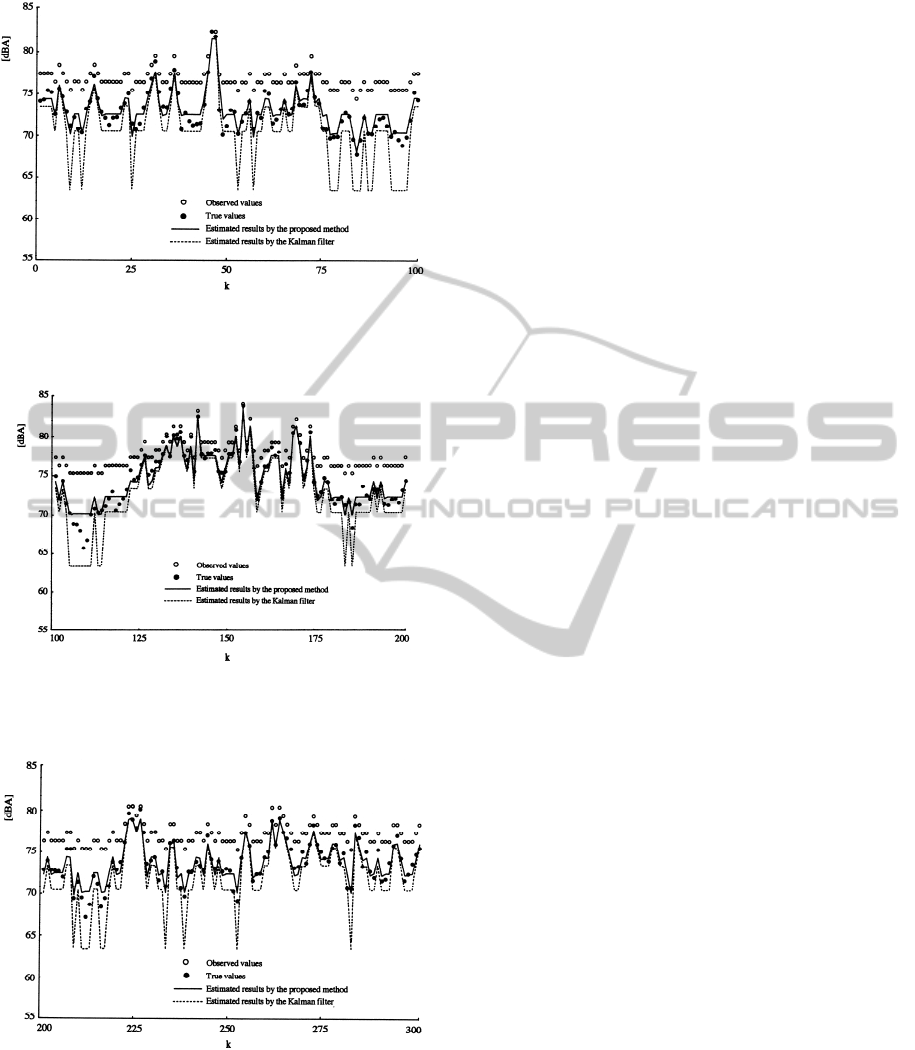
Figure 8: State estimation results for the road traffic noise
during a discrete time interval of [1, 100] sec., based on
the quantized data with 1 dB width.
Figure 9: State estimation results for the road traffic noise
during a discrete time interval of [101, 200] sec., based on
the quantized data with 1 dB width.
Figure 10: State estimation results for the road traffic
noise during a discrete time interval of [201, 300] sec.,
based on the quantized data with 1 dB width.
5 CONCLUSIONS
In this study, new methods for estimating a specific
signal embedded in fuzzy observations have been
proposed within static and dynamic frameworks.
The proposed estimation methods have been realized
by introducing a fuzzy logic approach into the
probabilistic description of the signal. The
effectiveness of the proposed fuzzy signal
processing method has been confirmed
experimentally by applying it to the real sound and
electromagnetic data.
REFERENCES
Bell, B. M., Cathey, F. W., 1993. The iterated Kalman
filter update as a Gaussian-Newton methods. In IEEE
Transactions on Automatic Control, Vol. 38, No. 2, pp.
294-297.
Eykhoff, P., 1984. System identification: parameter and
state estimation, John Wiley & Sons. New York.
Guoshen, Y., 2012. Solving inverse problems with
piecewise linear estimators: from gaussian mixture
models to structured sparsity. In IEEE Transactions on
Image Processing, Vol. 21, No. 5, pp. 2481-2499.
Ikuta, A., Ohta, M., Ogawa, H., 1997. Various regression
characteristics with higher order among light, sound
and electromagnetic waves leaked from VDT. In
Journal of International Measurement Confederation,
Vol. 21, No. 1-2, pp. 25-33.
Ikuta, A., Tokhi, M. O., Ohta, M., 2001. A cancellation
method of background noise for a sound environment
system with unknown structure.In IEICE Transactions
on Fundamentals of Electronics, Communications and
Computer Sciences, Vol. E84-A, No. 2, pp. 457-466.
Ikuta, A., Ohta, M., Siddique, M. N. H., 2005. Prediction
of Probability Distribution for the Psychological
Evaluation of Noise in the Environment Based on
Fuzzy Theory. In International Journal of Acoustics
and Vibration, Vol. 10, No. 3, pp. 107-114.
Julier, S. J., 2002. The scaled unscented transformation. In
Proceedings of American Control Conference, Vol. 6,
pp. 4555-4559.
Kalman, R. E., 1960. A new approach to linear filtering
and prediction problems. In Transactions of the ASME
Series D, Journal of Basic Engineering, Vol. 82, No.1,
pp. 35-45.
Kalman, R. E., Buch, R. S., 1961. New results in linear
filtering and prediction theory. In Transactions of the
ASME Series D, Journal of Basic Engineering, Vol. 83,
No. 1, pp. 95-108.
Kitagawa, G., 1996. Monte carlo filter and smoother for
non-Gaussian nonlinear state space models. In Journal
of Computational and Graphical Statistics, Vol. 5, No.
1, pp. 1-25.
Kushner, H. J., 1967. Approximations to optimal nonlinear
filter. In IEEE Transactions on Automatic Control,
Vol. 12, No. 5, pp. 546-556.
Ohta, M., Koizumi, T., 1968. General statistical treatment
of response of a non-linear rectifying device to a
FCTA2014-InternationalConferenceonFuzzyComputationTheoryandApplications
12