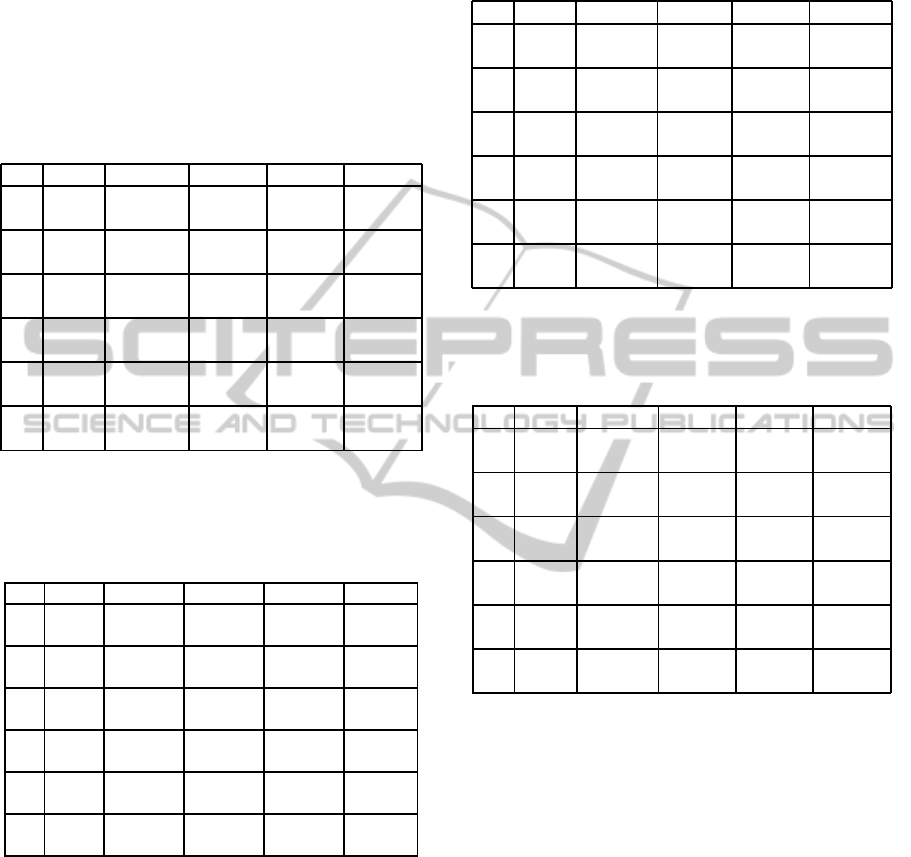
as a reference. For each estimation, all statistics are
calculated based on 10
4
samples and it is repeated 100
times to calculate the mean and standard deviation of
the estimates. For the MCMC method, we set 1000
times of iteration for the burn-in period.
Table 1: Means (standard deviations). Simulating quantiles
q such that 1 − p = F
S
n
(q) where F
S
n
(·) is a cumulative
distribution function of S
n
, S
n
=
∑
n
i=1
X
i
and P(X
i
> x) =
(1+ x)
−2
.
n 1− p MCMC SM DLW MC
10 1e-2 40.179 41.007 40.166 40.038
(0.130) (0.246) (0.459) (1.78)
1e-3 108.587 109.33 108.29 84.821
(0.197) (0.847) (1.081) (47.23)
1e-5 1008.1 1003.1 1007.5 609.42
(0.495) (18.5) (1.51) (1594)
30 1e-2 84.585 85.841 84.681 84.362
(0.324) (0.395) (1.237) (2.739)
1e-3 202.557 203.56 202.29 171.16
(0.373) (1.53) (2.40) (71.26)
1e-5 1760.3 1753.7 1759.0 114.23
(0.903) (41.12) (1.487) (443.5)
Table 2: Means (standard deviations). Simulating quantiles
q such that 1 − p = F
S
n
(q) where F
S
n
(·) is a cumulative
distribution function of S
n
, S
n
=
∑
n
i=1
X
i
and P(X
i
> x) =
(1+ x)
−3
.
n 1 − p MCMC SM DLW MC
10 1e-2 14.205 14.853 14.195 14.182
(0.069) (0.090) (0.154) (0.305)
1e-3 25.651 26.125 25.588 24.965
(0.062) (0.171) (0.412) (2.212)
1e-5 103.64 104.23 103.40 5.283
(0.091) (0.799) (0.553) (16.03)
30 1e-2 29.845 31.054 29.943 29.949
(0.297) (0.287) (0.519) (0.500)
1e-3 46.016 46.725 46.277 44.608
(0.184) (0.286) (1.041) (2.688)
1e-5 158.057 158.460 157.620 13.847
(0.152) (1.080) (0.273) (28.53)
From the table 1 and the table 2, the MCMC based
method perform well comparing with other methods.
For simulating conditional expectations in the table 3
and the table 4, we see MCMC based method can per-
form a little bit less than other methods in some pa-
rameter settings. However, when we see the extreme
tail, our proposed method works the best.
Next we consider random sums, meaning the
number of summands N is random. For numerical
experiments, we calculate the case N is geometrically
distributed:
P(N = n) = ρ(1 −ρ)
(n−1)
, (18)
Table 3: Means (standard deviations). Simulating con-
ditional expectations E(S
n
|S
n
> a
n
) where P(S
n
> a
n
) =
1− p, S
n
=
∑
n
i=1
X
i
and P(X
i
> x) = (1+ x)
−2
.
n 1− p MCMC SM DLW MC
10 1e-2 71.694 73.065 71.831 72.252
(1.44) (1.06) (1.22) (8.75)
1e-3 208.67 209.37 209.30 213.42
(4.16) (3.60) (4.99) (65.8)
1e-5 2005.4 2009.8 2009.3 4787.8
(35.5) (37.1) (30.9) (23168)
30 1e-2 139.03 140.55 139.14 140.76
(3.74) (2.22) (3.09) (17.34)
1e-3 374.25 375.76 378.24 391.06
(6.45) (5.00) (11.49) (96.36)
1e-5 3496.7 3500.0 3496.9 745.7
(58.7) (65.2) (59.8) (3671)
Table 4: Means (standard deviations). Simulating con-
ditional expectations E(S
n
|S
n
> a
n
) where P(S
n
> a
n
) =
1− p, S
n
=
∑
n
i=1
X
i
and P(X
i
> x) = (1+ x)
−3
.
n 1− p MCMC SM DLW MC
10 1e-2 19.206 20.044 19.257 19.495
(0.218) (0.167) (0.395) (0.905)
1e-3 36.321 36.911 36.463 41.032
(0.282) (0.327) (0.776) (5.53)
1e-5 153.50 154.39 153.83 132.74
(0.901) (1.326) (2.705) (491.7)
30 1e-2 36.922 38.603 37.200 37.744
(0.930) (0.902) (1.169) (1.581)
1e-3 61.125 62.013 62.066 69.369
(0.728) (0.416) (1.814) (7.973)
1e-5 230.22 230.27 230.00 225.14
(1.40) (1.92) (1.47) (932)
with some different parameter settings. This time we
simulate 5000 number of iteration and 1000 number
of burn-in period with 25 batches to calculate each
means and standard deviations of estimates. ES (ex-
pected shortfall) represents the conditional expecta-
tion. Note that we calculate relative errors instead of
standard deviations in order to see how the accuracy
changes. Table 7 shows that, when calculating quan-
tiles, the more high quantiles we estimate, the more
estimates become accurate. For expected short falls,
relative errors do not change remarkably but the es-
timates are sufficiently accurate. For other settings,
meaning X
i
’s follow Weibull and Log-normal distri-
butions, see the Appendix.
SIMULTECH2014-4thInternationalConferenceonSimulationandModelingMethodologies,Technologiesand
Applications
334