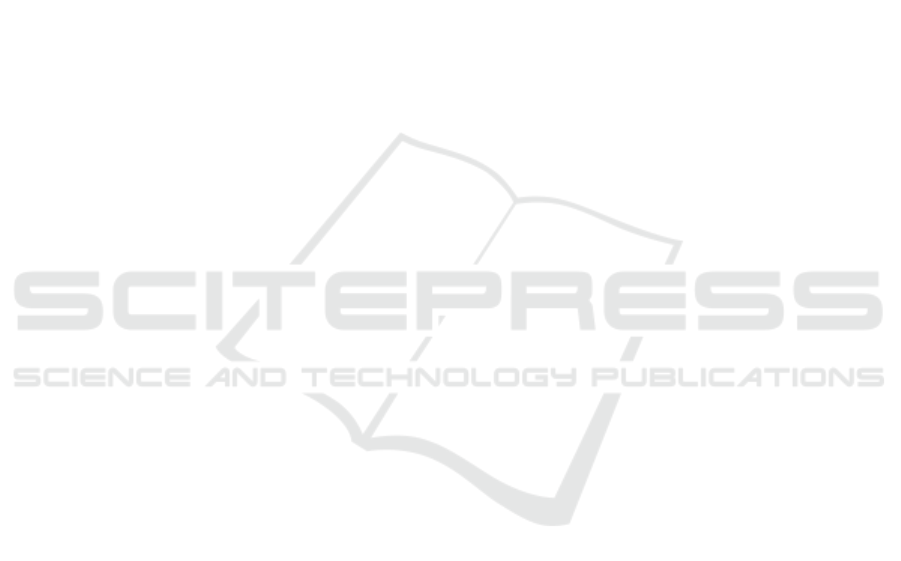
A Multi-Data Mining Approach for Shelf Space Optimization
Considering Customer Behaviour
Sheng-Hsiang Huang
1
, Chieh-Yuan Tsai
1
and Chih-Chung Lo
2
1
Department of Industrial Engineering and Management, Yuan Ze University, Taoyuan, Taiwan
2
Department of Applied Informatics, Fo Guang University, Yilan County, Taiwan
Keywords: Data Mining, Shelf Space Assignment, Moving and Purchase Patterns, Customer Behaviour.
Abstract: A well product-to-shelf assignment strategy can help customers easily find product items and dramatically
increase the retailing store profit. Previous studies in this area usually applied the space elasticity to
optimize product assortment and space allocation models. However, a well product-to-shelf assignment
strategy should not only consider product assortment and space elasticity. Thus, this study develops a
product-to-shelf assignment approach by considering both product association rules and traveling behaviour
of consumer. Specifically, the first task of this research is to develop a method to discover traveling
behaviour of consumer, which includes both product association rules and traveling behaviour of consumer,
in the store. The second task is to construct and solve a product-to-shelf assignment model, based on the
information provided in the first task. In this research, products are classified as major item, minor item and
the others. Only minor will be reassigned. Experimental result shows our proposed method can reassign
minor items to suitable shelves and increase cross-selling opportunity of major and minor items.
1 INTRODUCTION
To implement a retail strategy, store managers
should develop a retail mix that satisfies the need of
its target market. The elements in retail mix include
store location, product assortment, pricing,
advertising and promotion, store design and display,
services and personal selling (Levy and Weitz,
1995). Among that, product assortment and shelf
space allocation are two important issues which
dramatically affect customers’ purchasing decisions
(Yang, 1999). However, using space elasticity for
shelf space allocation needs to estimate a great
quantity of parameters which results in high cost and
errors in the mathematical model.
Recently, the progress of information technology
makes retailers easily collect daily transaction data.
With the novel information technology, retailers can
solidify ephemeral relationships with customers into
long-term and fruitful relationships if they can
discover customer behavior from collected data.
Data mining is one of the most popular technologies
that discover potential customer knowledge from
business databases to assist a policy decision. Chen
and Lin (2007) applied the multi-level association
rule mining to explore the relationships between
products as well as between product categories for
resolving the product assortment and allocation
problems in retailing. Although the association rules
to assist managers in developing better layout for
stores, their method is more suitable for the case of
new stores or joint sales. For an existing store, the
frequent purchase pattern may not maintain if the
customer’s interesting products are not at the
original locations or shelves anymore.
Except purchasing association between products,
customer traveling behaviors/patterns should be
considered in solving product-to-shelf assignment
problem. When shopping in a store, a customer
travels around the aisles of a store, stops at certain
locations, deliberates about his/her consideration,
and chooses the best options. This process is
repeated until the whole shopping trip completes.
Recently, some studies tried to tackle the shopping
path problem. Larson et al. (2005) presented
exploratory analyses of an extraordinary new dataset
that reveals the path taken by individual shoppers
around an actual grocery store, as provided by RFID
tags located on their shopping carts.
As mentioned above, some researchers employed
product association rules mining to improve shelf
space allocation, while other researchers focused on
89
Huang S., Tsai C. and Lo C..
A Multi-Data Mining Approach for Shelf Space Optimization - Considering Customer Behaviour.
DOI: 10.5220/0005037700890095
In Proceedings of the 11th International Conference on e-Business (ICE-B-2014), pages 89-95
ISBN: 978-989-758-043-7
Copyright
c
2014 SCITEPRESS (Science and Technology Publications, Lda.)