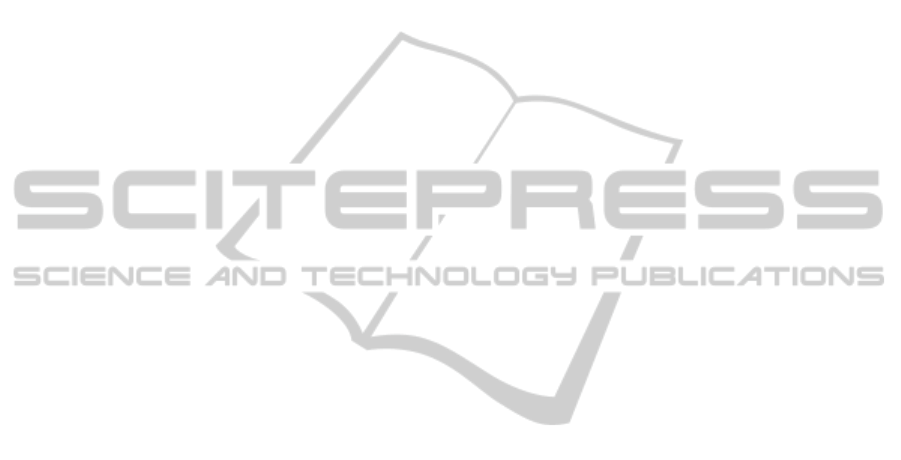
α value, when the reference signal changes. There are
two different reasons which may cause these anoma-
lies. As stated earlier, control effort is obtained so that
the process output can track the reference signal and
hence process output expression is the reason behind
this reduction. Process output expression uses two
MLFs for its computation (see (36), (32) and (33)).
MLF takes a long time for computation and the na-
ture of MLF changes very rapidly for small α values.
The other reasons behind irregularity can be the infi-
nite memory nature of the FO system. For small α
values a larger amount of past values are considered
for computation.
Both these observations are not seen as soon as
constraints are applied on the input as well as out-
put. In this paper, unconstrained case was considered
since for an optimal solution one needs to first con-
firm that solution exists for unconstrained case before
going to constrained situation. In optimal theory, it is
said that if the control law does not deliveroptimal so-
lution and robustness in the unconstrained case then it
will not give optimal solution in the constrained case
(Rossiter, 2003). Unfortunately the opposite does not
hold true and hence it is worthwhile to check the per-
formance of the system for unconstrained case first.
From these results it is evident that FO system’s
model can be used in its true form using approxima-
tion method for MPC. Furthermore, the obtained pro-
cess and model output depicts the behaviour of the
original FO system in terms of nature of plot and the
required settling time. Systems with small values of α
tend to take large time to settle. The reference trajec-
tory is followed faithfully. The reference signal used
for the simulation contains both positive and negative
steps and hence it can be inferred that MPC for FO
systems can work for all types of limiting reference
signals.
6 CONCLUSION
Fractional-order systems are represented using FO
model and for MPC, the model is required for pre-
diction purposes. A discrete state-space model de-
livers straightforward prediction equations with one
condition that the model should be of strictly proper
nature. Control law was obtained for unconstrained
MPC problem and was utilized to obtain results.
It was observed that MPC can be utilized for FO
plants once the model of the FO plant is obtained and
a unique analytical expression can be used to depict
process output for FO plants. Approximation method
was used to obtain model of the FO plant and its au-
thenticity was verified using frequency and step re-
sponse. Obtained results reveal that MPC can be used
for FO plants and the model used works fine. More-
over, use of systematic expression obtained for pro-
cess output makes it realistic. Hence, classical servo
problem can be solved for a class of linear FO systems
with the help of approximation method using MPC.
Use of other approximation methods like modi-
fied Oustaloup, Carlson will be interesting for MPC
of FO systems. Furthermore, a comparison of these
approximation methods for a single FO system might
reveal some motivating results and hence the study of
MPC with constraints for FO systems would be quite
interesting and worth exploring as a future scope.
REFERENCES
Bemporad, A. (2006). Model predictive control design:
New trends and tools. IEEE Conference on Decision
and Control.
Boudjehem, D. and Boudjehem, B. (2012). A fractional
model predictive control for fractional order systems.
Fractional Dynamics and Control Springer, pages 59–
71.
Camacho, E. and Bordons, C. (2004). Model Predictive
control, volume 2. Springer.
Caponetto, R., Dongola, G., and Fortuna, L. Fractional Or-
der Systems Modeling and Control Applications, vol-
ume 72. World Scientific Publishing Co. Pvt. Ltd.,
second edition.
Dzieli´nski, A. and Sierociuk, D. (2010). Fractional or-
der model of beam heating process and its experi-
mental verification. In New trends in nanotechnology
and fractional calculus applications, pages 287–294.
Springer.
Enacheanu, O., Riu, D., Reti`ere, N., Enciu, P., et al. (2006).
Identification of fractional order models for electrical
networks. In Proceedings of IEEE Industrial Elec-
tronics IECON 2006–32nd Annual Conference on,
pages 5392–5396.
Gabano, J.-D. and Poinot, T. (2011). Fractional modelling
and identification of thermal systems. Signal Process-
ing, 91(3):531–541.
Garcia, C., Prett, D., and Morari, M. (1989). Model predic-
tive control: Theory and practice-a survey. Automat-
ica, 25(3):335–348.
Hortelano, M. R., de Madrid y Pablo, A. P., Hierro, C., and
Berlinches, R. H. (2010). Generalized predictive con-
trol of arbitrary real order. New trends in nanotechnol-
ogy and fractional calculus applications, Springer.
J.M.Maciejowski. Predictive Control with Constraints.
Prentice Hall.
Mansouri, R., Bettayeb, M., and Djennoune, S. (2010). Ap-
proximation of high order integer systems by frac-
tional order reduced-parameters models. Mathemat-
ical and Computer Modelling, 51(1):53–62.
ModelPredictiveControlforFractional-orderSystem-AModelingandApproximationBasedAnalysis
371