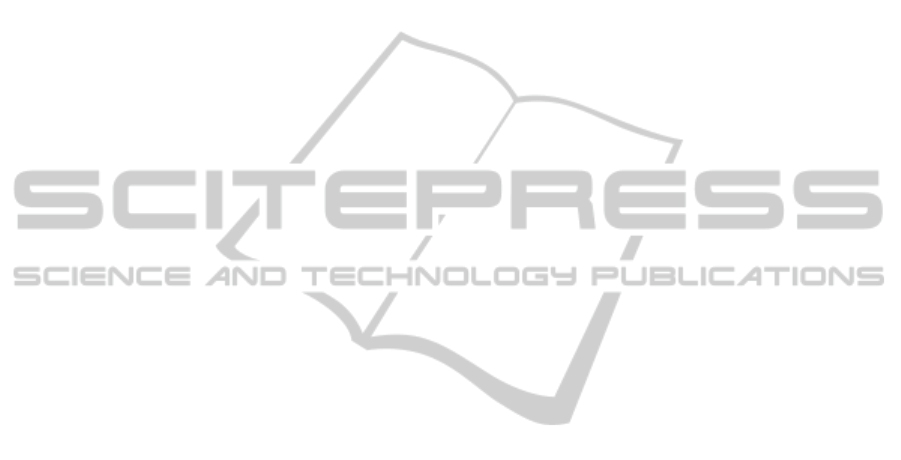
in literature the neural network approach was often
overlooked because of the hard obstacle encountered
in the prediction of the shunt resistance. The solu-
tion of switching to closed forms for the identification
of the model, shifts the problem of the shunt resis-
tance calculus to the solution of an explicit non-linear
equation. In conclusion, NNs can identify the R
S
and
a parameters correctly, making the NN approach vi-
able for model identification in conjunction with the
adoption of reduced forms for the computation of the
three remaining unknown parameters of the one diode
model. The so achieved NN for the estimation of the
series resistance R
S
and the modified ideality factor
a, and the closed form for the computation of the re-
maining dependent parameters constitute a complete
tool extremely easy to be implemented in a microcon-
troller based architecture: this is another successful
step made thanks to Soft Computing techniques in the
development of intelligent systems for the monitoring
and the management of renewable generation plants.
REFERENCES
Askarzadeh, A. and Rezazadeh, A. (2013). Artificial bee
swarm optimization algorithm for parameters iden-
tification of solar cell models. Applied Energy,
102(0):943 – 949.
Blair, N. J., Dobos, A. P., and Gilman, P. (2010). Com-
parison of photovoltaic models in the system advisor
model. In Solar 2013, Baltimore, Maryland.
California Energy Commission (2013). CECPV
calculator version 4.0. [Online]. Avialable:
http://www.gosolarcalifornia.org/tools/nshpcalculator/.
Capizzi, G., Coco, S., Giuffrida, C., and Laudani, A. (2004).
A neural network approach for the differentiation of
numerical solutions of 3-d electromagnetic problems.
Magnetics, IEEE Transactions on, 40(2):953–956.
Carrasco, M., Mancilla-David, F., Fulginei, F. R., Laudani,
A., and Salvini, A. (2013). A neural networks-based
maximum power point tracker with improved dynam-
ics for variable dc-link grid-connected photovoltaic
power plants. International Journal of Applied Elec-
tromagnetics and Mechanics, 43(1).
Cubas, J., Pindado, S., and Victoria, M. (2014). On the an-
alytical approach for modeling photovoltaic systems
behavior. Journal of Power Sources, 247:467 – 474.
Cybenko, G. (1989). Approximation by superpositions of
a sigmoidal function. Mathematics of control, signals
and systems, 2(4):303–314.
Desoto, W., Klein, S., and Beckman, W. (2006). Improve-
ment and validation of a model for photovoltaic array
performance. Solar Energy, 80(1):78–88.
El-Naggar, K., AlRashidi, M., AlHajri, M., and Al-Othman,
A. (2012). Simulated annealing algorithm for pho-
tovoltaic parameters identification. Solar Energy,
86(1):266 – 274.
Fulginei, F. R., Laudani, A., Salvini, A., and Parodi, M.
(2013). Automatic and parallel optimized learning
for neural networks performing MIMO applications.
Advances in Electrical and Computer Engineering,
13(1):3–12.
Hornik, K., Stinchcombe, M., and White, H. (1989). Multi-
layer feedforward networks are universal approxima-
tors. Neural networks, 2(5):359–366.
Hunter, D., Yu, H., Pukish, M. S., Kolbusz, J., and Wil-
amowski, B. M. (2012). Selection of proper neural
network sizes and architectures - A comparative study.
Industrial Informatics, IEEE Trans. on, 8(2):228–240.
Ilonen, J., Kamarainen, J.-K., and Lampinen, J. (2003).
Differential evolution training algorithm for feed-
forward neural networks. Neural Processing Letters,
17(1):93–105.
Ishaque, K. and Salam, Z. (2011). An improved modeling
method to determine the model parameters of photo-
voltaic (PV) modules using differential evolution (de).
Solar Energy, 85(9):2349 – 2359.
Jiang, L. L., Maskell, D. L., and Patra, J. C. (2013). Pa-
rameter estimation of solar cells and modules using
an improved adaptive differential evolution algorithm.
Applied Energy, 112(0):185 – 193.
Kim, C.-T. and Lee, J.-J. (2008). Training two-layered feed-
forward networks with variable projection method.
Neural Networks, IEEE Trans. on, 19(2):371–375.
Laudani, A., Fulginei, F. R., and Salvini, A. (2014a). High
performing extraction procedure for the one-diode
model of a photovoltaic panel from experimental I–V
curves by using reduced forms. Solar Energy, 103:316
– 326.
Laudani, A., Fulginei, F. R., Salvini, A., Lozito, G. M., and
Coco, S. (2014b). Very fast and accurate procedure
for the characterization of photovoltaic panels from
datasheet information. International Journal of Pho-
toenergy, 2014. Article ID 946360.
Laudani, A., Mancilla-David, F., Riganti-Fulginei, F., and
Salvini, A. (2013). Reduced-form of the photovoltaic
five-parameter model for efficient computation of pa-
rameters. Solar Energy, 97(0):122 – 127.
Li, Y., Huang, W., Huang, H., Hewitt, C., Chen, Y., Fang,
G., and Carroll, D. L. (2013). Evaluation of methods
to extract parameters from currentvoltage characteris-
tics of solar cells. Solar Energy, 90(0):51 – 57.
Liu, Y.-H., Liu, C.-L., Huang, J.-W., and Chen, J.-H.
(2013). Neural-network-based maximum power point
tracking methods for photovoltaic systems operating
under fast changing environments. Solar Energy,
89:42–53.
Mancilla-David, F., Riganti-Fulginei, F., Laudani, A., and
Salvini, A. (2014). A neural network-based low-cost
solar irradiance sensor. Instrumentation and Measure-
ment, IEEE Trans. on.
Parodi, M., Fulginei, F. R., and Salvini, A. (2012). Learn-
ing optimization of neural networks used for mimo ap-
plications based on multivariate functions decomposi-
tion. Inverse Problems in Science and Engineering,
20(1):29–39.
Rajasekar, N., Kumar, N. K., and Venugopalan, R. (2013).
NCTA2014-InternationalConferenceonNeuralComputationTheoryandApplications
136