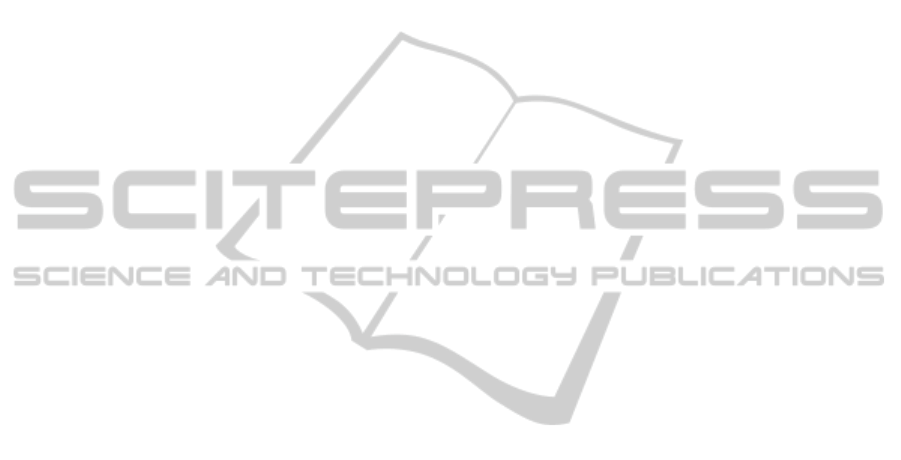
results and discusses advantages and disadvantages
of these methods.
Machine learning algorithms can be successfully
employed with the presented data set with the
average accuracy 81.4%.
We also split the data to the training and the test
set to identify courses for which ML cannot be
successfully applied to courses with more than 81%
or less than 61.5% successful students in the training
set. The results in the test set were also not so
convincing when there was a significant difference
between the training set and the test set.
On the other hand, we can apply the following
discovered rule for easier courses. All students with
the weighted average grade ≤ 3 and the ratio of
gained credits to credits to gain > 0.8 are successful.
This fills the gap.
We also defined rules based on the grade
averages of students and their friends. One
conclusion was that the prediction was more
accurate when only close friends were considered.
This approach offered the precision about 97% but
decreased the recall to 53%.
In the future work, we intend to find the
appropriate balance of using these methods and to
combine precise association rules to get the most
accurate predictions with a reliable recall. The
courses evinced the relations with other courses will
be explored. We also intend to enrich the data with
temporal features that can improve the current
results.
These predictions will constitute a part of the
course enrollment recommender system which will
help students to select courses and warn them
against difficult courses they have to pass.
ACKNOWLEDGEMENTS
We thank Lubomír Popelínský, colleagues of
Knowledge Discovery Lab, and also all colleagues
of IS MU development team for their assistance.
This work has been partially supported by Faculty of
Informatics, Masaryk University.
REFERENCES
Bydžovská, H. and Popelínský L. 2014. Course
Recommendation from Social Data. Proceedings of
the 6
th
International Conference on Computer
Supported Education, Volume 1, pp. 268-275.
Bydžovská, H. and Popelínský L. 2014 II. The Influence
of Social Data on Student Success Prediction.
Proceedings of the 18
th
International Database
Engineering & Applications Symposium, pp. 374-375.
Jannach, D. and Zanker, M. and Felfernig, A. and
Friedrich, G. 2011. Recommender Systems An
Introduction, Cambridge University Press.
Kasprzak, J. and Brandejs, M. and Čuhel, M. and Obšívač,
T. 2010. Access rights in enterprise full-text search. In
Proceedings of the 12
th
International Conference on
Enterprise Information Systems, Volume 1, pp. 32–39.
Lavrač, N. and Železný, F. and Flach, P. A. 2002. RSD:
Relational Subgroup Discovery through First-Order
Feature Construction. In 12th International
Conference on Inductive Logic Programming, pp.
149-165.
Lavrač, N and Kavšek, B. 2006. APRIORI-SD: Adapting
Association Rule Learning to Subgroup Discovery.
Applied Artificial Intelligence, pp. 543-583.
Liu, B. and Hsu, W. and Ma, H. 1998 Integrating
classification and association rule mining. Proceedings
of the 4th international conference on Knowledge
Discovery and Data mining KDD'98, page 80-86.
AAAI Press.
Nooy, W. and Mrvar, A. and Batagelj, V. 2011.
Exploratory Social Network Analysis with Pajek.
Structural Analysis in the Social Sciences. Cambridge
University Press.
Poldin, O. and Valeeva D. and Yudkevich M. 2014.
Friendship and study assistance ties of university
students. In WP BRP Series: Sociology / SOC, pages
1-17.
Peña-Ayala, A. 2014. Educational data mining: A survey
and a data mining-based analysis of recent works. In
Expert Systems with Applications , pp. 1432–1462.
Thammasiri, D. and Delen, D. and Meesad, P. and Kasap
N. 2014. A critical assessment of imbalanced class
distribution problem: The case of predicting freshmen
student attrition. In Expert Systems with Applications
41, pp. 321–330.
Vialardi, C. and Agapito, J. and Shafti, L. and Ortigosa, A.
2009. Recommendation in higher education using data
mining techniques. In T. Barnes, M. Desmarais, C.
Romero, and S. Ventura (eds.), Proceedings of the 2nd
International Conference on Educational Data Mining
(EDM’09), pages 191–199.
Vialardi, C. and Chue, J. and Barrientos, A. and Victoria,
D. and Estrella, J. and Ortigosa, A. and Peche, J. 2010.
A case-study: Data mining applied to student
enrollment. In Proceedings of Third Educational Data
Mining Conference, Pennsylvania, USA, pages 333–
335.
Witten, I. and Frank, E and Hall, M. 2011. Data Mining:
Practical Machine Learning Tools and Techniques,
3rd edition, Morgan Kaufmann Publishers.
TowardsStudentSuccessPrediction
169