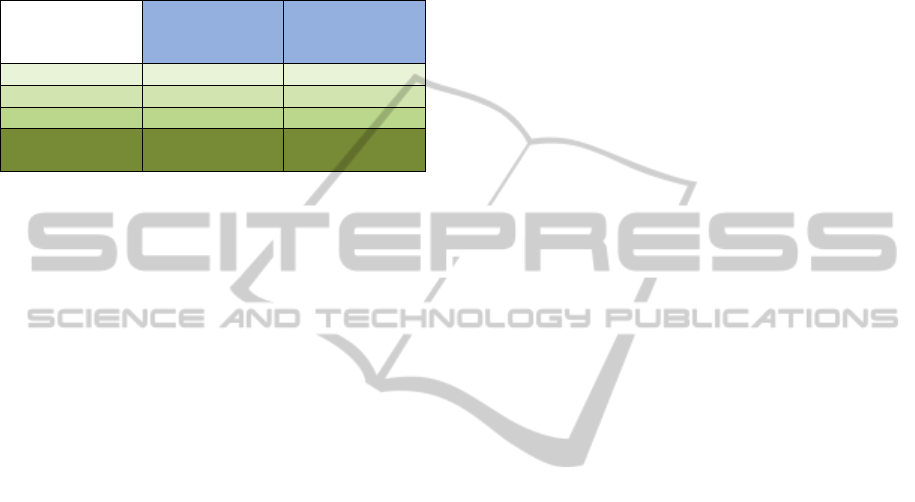
than recognition rates found by (Mahdi, 2008). This
result shows that our system of tracking and
characterization of lip movements contributes to
improve visual recognition of visemes.
Table 3: Experimental results are found with the SVM of
polynomial kernel and with three different cases of
training.
Recognition
Rate (Mahdi,
2008)
Recognition
Rate (Our
method)
/Ba/ 72.73% 91.66%
/Bi/ 90.91% 81.8181%
/Bou/ 81.82% 91.6666%
Recognition
Rate
81.82% 88.57%
4 CONCLUSIONS
Systems of visual recognition of the speech require
visual descriptors. So, to extract these descriptors, it
is necessary to make a localizing and an automatic
tracking of the labial gestures.
Our objective is to provide a method for
segmenting the labial area and to tracking and
characterizing the lip movements with the best
possible precision to achieve better recognition of
visemes.
In this paper, we presented early our
segmentation method to extract the outer contour of
the lips. Then, we track the lip contour using the
particle filter and extract lip descriptors throughout
the syllable sequence. Finally we chose the SVM
method for training and recognition of visemes. This
approach has been tested with success on our
audiovisual corpus.
Our lip-reading system can be improved by
integrating many new perspectives. In fact, we plan
to expand the content of our corpus by adding others
visemes, different words and even phrases from
different languages. We can also add audio
information.
REFERENCES
Beaumesnil, B., 2006. Real Time Tracking for 3D
Realistic Lip Animation. In ICPR, International
Conference Pattern Recognition. IEEE.
Bouvier, C., 2010. Segmentation Region-Contour des
contours des lèvres. Prepared in the laboratory
GIPSA-lab/DIS within the Graduate School
Electronics, Electrotechnics, Automation & Signal
Processing Laboratory of Computer Vision and
Systems Université Laval.
Kalbkhani, H., Amirani, M., 2012. An Efficient Algorithm
for Lip Segmentation in Color Face Images Based on
Local Information. In JWEET’01, Journal of World’s
Electrical Engineering and Technology. Science-line.
Liu, X., Cheung Y., 2011. A robust lip tracking algorithm
using localized color active contours and deformable
models.In ICASSP, IEEE International Conference on
Acoustics, Speech and Signal Processing.IEEE.
Mahdi, W., Werda, S., Ben Hamadou, A., 2008. A hybrid
approach for automatic lip localization and viseme
classification to enhance visual speech recognition.In
ICAE’03, Integrated Computer-Aided Engineering.
ACM.
Majumdar, J., Kiran, S., 2013. Particle Filter Integrating
Color Model for Tracking. In IJETAE’07,
International Journal of Emerging Technology and
Advanced Engineering.
Meng, J., Liu, J., Zhao, J., Wang, J., 2014. Research of
Real-time Target Tracking Base on Particle Filter
Framework. In Jofcis’06. Journal of Computational
Information Systems. Binary Information Press.
Nicolas, E., 2003. Segmentation des lèvres par un modèle
déformable analytique. Prepared at the Laboratory of
Images and Signals (LIS) within the Doctoral School.
Segura, C., Hernando, J., 2014. 3D Joint Speaker Position
and Orientation Tracking with Particle Filters. In
Sensors’02. Sensors and Transducers Journal. MDPI.
Shirinzadeh, F., Seyedarabi, H., Aghagolzadeh, A., 2012.
Facial Features Tracking Using Auxiliary Particle
Filtering and Observation Model Based on
Bhattacharyya Distance. In IJCTE’05. International
Journal of Computer Theory and Engineering.
EBSCO.
Stillittano, S., Girondel, V., Caplier, A., 2013. Lip contour
segmentation and tracking compliant with lip-reading
application constraints. In Mach. Vis. Appl.’01.
Proceedings of Mach. Vis. Appl. Springer-Verlag.
Sunil, M., Patnaik, S., 2013. Automatic Lip Tracking and
Extraction of Lip Geometric Features for Lip Reading.
In IJMLC’02. International Journal of Machine
Learning and Computing. IACSIT.
Sunil, M., Patnaik, S., 2014. Lip reading using DWT and
LSDA. In IACC. IEEE International Advance
Computing Conference . IEEE.
LipTrackingUsingParticleFilterandGeometricModelforVisualSpeechRecognition
179