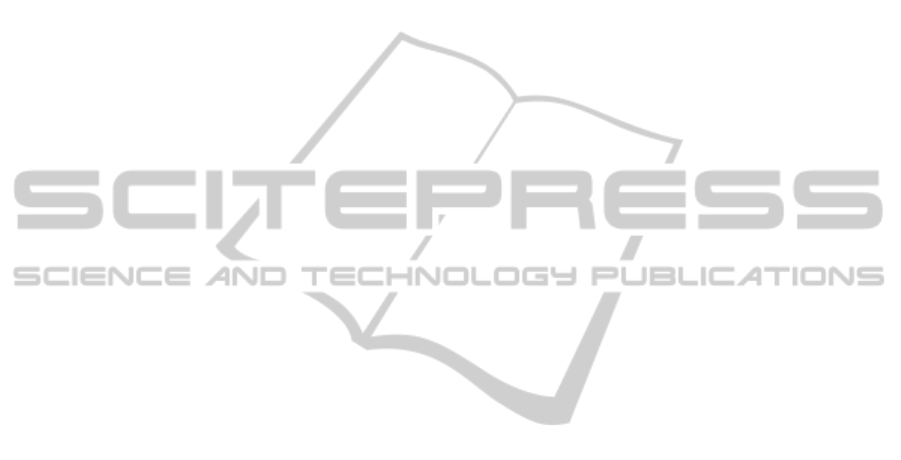
6. In detail, everything, including no pricing informa-
tion at all (x
2B1
), seems to be be better than advertising
”no deposit” (x
2B2
). The next best text feature in terms
of pricing is to give an exact value for the percentage
that a user can save on the advertiser’s website (x
2B3
).
This feature is performing credibly better than those
mentioned above. What is the best way to communi-
cate pricing in Paid Search Ads - given the data - is
to provide exact pricing information from the adver-
tiser’s database (x
2B4
). In fact it is credibly superior to
any other feature in terms of pricing communication,
given the observed data.
4 CONCLUSIONS AND
OUTLOOK
What we applied in this paper offers a valid way to
evaluate text features and other nominal predictive
variables where tests are an essential part of the daily
business. In terms of substantive issues it is the hard
facts that the potential customers are looking for when
they research in a search engine. The more spe-
cific information on pricing is provided in an ad
- the better is the chance of winning the customers
click. Building up trust is one good feature for an ad-
vertiser to support this effect or even substitute parts
of this positive effect if they do not have competi-
tive prices or special rebates available. In this spe-
cific case this has been achieved by communicating
the existence of a credible trust seal in the ad-copy.
What we did not asses in this research but would find
interesting for an ongoing investigation is the ques-
tion whether these findings have additional impact on
the conversion probability on the advertiser’s landing-
page as well. Applying Bayesian ANOVA to mul-
tivariate tests in Online Advertising, especially Paid
Search Advertising, has various advantages compared
to applying conventional Analysis of Variance. This
is especially true for unbalanced data like the present
one. One obvious limitation to the results is that they
should probably only be true for advertisers with com-
petitive prices. Additionally this test should be re-
peated for a number of other advertisers from various
industries to answer the question whether these obser-
vations can be generalized.
REFERENCES
Agarwal, A., Hosanagar, K., and Smith, M. D. (2011). Lo-
cation, Location, Location: An Analysis of Profitabil-
ity of Position in Online Advertising Markets. Journal
of Marketing Research, 48(6):1057.1073.
Alby, T. and Funk, B. (2011). Search Engine Marketing in
Small and Medium Companies: Status Quo and Per-
spectives. In Cruz-Cunha, M. and Varaj
˜
ao, J., editors,
E-Business Managerial Aspects, Solutions and Case
Studies, pages 206–221. igi-global.
Blask, T. (2013). Applying Bayesian Parameter Estimation
to A / B Tests in E-Business Applications : Examining
the Impact of Green Marketing Signals in Sponsored
Search Advertising. In Proceedings of the Interna-
tional Conference on e-Business (ICE-B 2013).
Blask, T., Funk, B., and Schulte, R. (2011). Should Com-
panies Bid on their Own Brand in Sponsored Search?
In Proceedings of the International Conference on e-
Business (ICE-B 2011), pages 14–21, Seville, Spain.
Chapelle, O. and Zhang, Y. (2009). A dynamic bayesian
network click model for web search ranking. In
Proceedings of the 18th international conference on
World wide web - WWW ’09, page 10, New York, New
York, USA. ACM Press.
Craswell, N., Zoeter, O., Taylor, M., and Ramsey,
B. (2008). An experimental comparison of click
position-bias models. In Proceedings of the interna-
tional conference on Web search and web data mining
- WSDM ’08, page 8, New York, New York, USA.
ACM Press.
Dave, K. S. and Varma, V. (2010). Learning the click-
through rate for rare/new ads from similar ads. In
Proceeding of the 33rd international ACM SIGIR con-
ference on Research and development in information
retrieval - SIGIR ’10, number July, page 897, New
York, New York, USA. ACM Press.
Gauzente, C. (2009). Information search and paid results
proposition and test of a hierarchy-of-effect model.
Electronic Markets, 19(2-3):163–177.
Gluhovsky, I. (2010). Forecasting Click-Through Rates
Based on Sponsored Search Advertiser Bids and In-
termediate Variable Regression. October, 10(3):1–28.
Jansen, B. and Mullen, T. (2008). Sponsored search: an
overview of the concept, history, and technology. In-
ternational Journal of Electronic Business, 6(2):114–
131.
Jansen, B. J., Flaherty, T. B., Baeza-Yates, R., Hunter, L.,
Kitts, B., and Murphy, J. (2009). The Components and
Impact of Sponsored Search. Computer, 42(5):98–
101.
Jansen, J. (2011). The Serious Game of Bidding on Key-
words. In Understanding Sponsored Search Core El-
ements of Keyword Advertising, chapter 8, pages 176–
201. Cambridge University Press.
Joachims, T., Granka, L., Pan, B., Hembrooke, H., and
Gay, G. (2005). Accurately interpreting clickthrough
data as implicit feedback. In Proceedings of the 28th
annual international ACM SIGIR conference on Re-
search and development in information retrieval - SI-
GIR ’05, page 154, New York, New York, USA. ACM
Press.
Joachims, T., Granka, L., Pan, B., Hembrooke, H., Radlin-
ski, F., and Gay, G. (2007). Evaluating the accuracy
of implicit feedback from clicks and query reformula-
tions in Web search. ACM Transactions on Informa-
tion Systems, 25(2):1–26.
DoSpecificTextFeaturesInfluenceClickProbabilitiesinPaidSearchAdvertising?
61