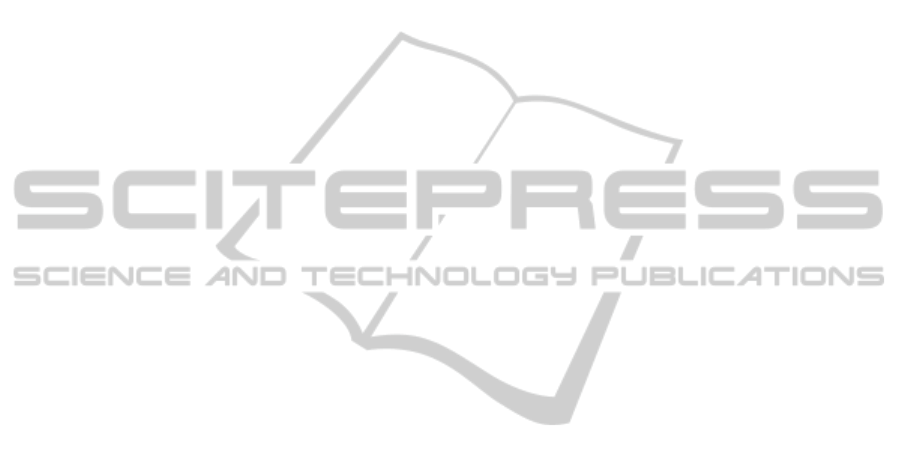
8. Kim, Y. K. and H. Altan, Using dynamic simulation for demonstrating the impact of
energy consumption by retrofit and behavioural change in Building Simulation 2013
Conference2013: Chambery, France.
9. Wei, S., R. Jones, and P. de Wilde, Driving factors for occupant-controlled space heating in
residential buildings. Energy and Buildings, 2014. 70(0): p. 36-44.
10. Fabi, V., et al., Occupants' window opening behaviour: A literature review of factors
influencing occupant behaviour and models. Building and Environment, 2012. 58(0): p.
188-198.
11. Gardner, G.T. and P.C. Stern, The Short List: The Most Effective Actions U.S. Households
Can Take to Curb Climate Change. Environment: Science and Policy for Sustainable
Development, 2008. 50(5): p. 12-25.
12. DesignBuilder. DesignBuilder website. 2014; Available from: http://www.design
builder.co.uk/.
13. DOE. EnergyPlus Energy Simulation Software 2014; Available from: v jkhttp://apps1.eere.
energy.gov/buildings/energyplus/.
14. Kalogirou, S., Artificial neural networks in energy applications in buildings. International
Journal of Low Carbon Technologies, 2006. 1(3).
15. Azadeh, A., S.F. Ghaderi, and S. Sohrabkhani, Annual electricity consumption forecasting
by neural network in high energy consuming industrial sectors. Energy Conversion and
Management, 2008. 49(8): p. 2272-2278.
16. Swan, L.G., V.I. Ugursal, and I. Beausoleil-Morrison, Occupant related household energy
consumption in Canada: Estimation using a bottom-up neural-network technique. Energy
and Buildings, 2011. 43(2–3): p. 326-337.
17. Luo, X., et al., A fuzzy neural network model for predicting clothing thermal comfort.
Computers & Mathematics with Applications, 2007. 53(12): p. 1840-1846.
18. Kalogirou, S.A., Long-term performance prediction of forced circulation solar domestic
water heating systems using artificial neural networks. Applied Energy, 2000. 66(1): p.63-74.
19. Kalogirou, S.A., S. Panteliou, and A. Dentsoras, Artificial neural networks used for the
performance prediction of a thermosiphon solar water heater. Renewable Energy, 1999.
18(1): p. 87-99.
20. Lee, W.-Y., J.M. House, and N.-H. Kyong, Subsystem level fault diagnosis of a building's
air-handling unit using general regression neural networks. Applied Energy, 2004. 77(2): p.
153-170.
21. Curtiss, P.S., M.J. Brandemuehl, and J.F. Kreider, Energy management in central HVAC
plants using neural networks. ASHRAE Transactions, 1994.
22. Rafiq, M.Y., G. Bugmann, and D.J. Easterbrook, Neural network design for engineering
applications. Computers & Structures, 2001. 79(17): p. 1541-1552.
23. Werbos, P.J., Beyond Regression: New tools for prediction and analysis in the behavioural
science, 1974, Harvard University.
24. MathWorks. Neural Network Toolbox. 2014; Available from: http://www.mathworks.
co.uk/products/neural-network/.
10