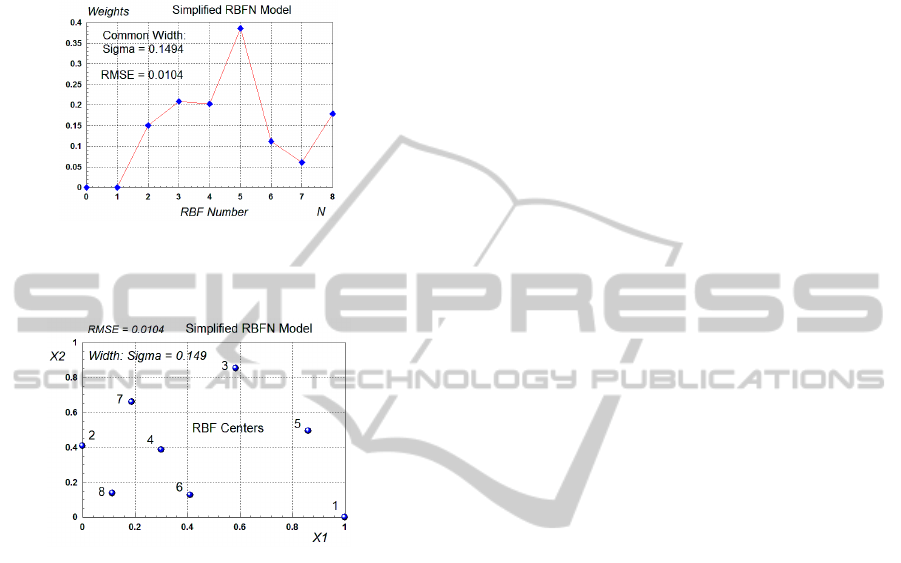
similar. Therefore a conclusion could be made that
the Simplified RBFN model is able to achieve
almost the same accuracy as the Reduced RBFN
model, but with smaller number of parameters,
namely L=26 versus L=33.
Figure 15: The values of the Weights for the best
Simplified RBFN model with N=8 RBFs and one common
Width.
Figure 16: Locations of all 8 Centers of the best
Simplified RBFN model with N=8 and RMSE = 0.0104.
It is seen from Fig. 15 and Fig. 16 that RBF1 (shown
as Center 1 in Fig. 16) is inactive, because its weight
is zero (as seen from Fig. 15). This is another case of
redundancy in the parameters (or in the number of
RBFs) of the model.
6 CONCLUSIONS
The investigations in this paper were focused on the
performance analysis of the RBFN models with two
slightly different structures, namely the Reduced
RBFN and Simplified RBFN models.
One of the three optimization strategies
explained in this paper is the one-step Strategy3,
which optimizes simultaneously all three groups of
parameters, namely the Centers of the RBFs, their
Widths and the Weights. A modified version of the
PSO algorithm with constraints was used for tuning
the parameters of both Reduced and Simplified
RBFN models on a test nonlinear example with
different number of the RBFs.
The Simplified RBFN model has the smallest
number of parameters, because it uses one common
width for all RBFs, unlike the Reduced RBFN
model that uses different widths for the RBFs.
The simulation results have shown that despite
the smaller number of parameters, the Simplified
RBFN models are able to achieve almost the same
accuracy, as the Reduced RBFN models. Therefore
the Simplified RBFN could be the preferable choice
for creating RBFN models.
The further research is focused on solving
another optimization problem such as the optimal
selection of the RBF units used in creating the
RBFN models.
ACKNOWLEDGEMENTS
This paper has been produced with the financial
assistance of the European Social Fund, project
number BG051PO001-3.3.06-0014. The authors are
responsible for the content of this material, which
under no circumstances can be considered as an
official position of the European Union and of the
Ministry of Education and Science of Bulgaria.
REFERENCES
Poggio, T., Girosi, F., 1990. Networks for approximation
and learning. Proceedings of the IEEE, 78, 1481-1497.
Musavi, M., Ahmed, W., Chan, K., Faris, K., Hummels,
D., 1992. On the training of radial basis function
classifiers. Neural Networks, 5, 595–603.
Park, J., Sandberg, I.W., 1993. Approximation and radial-
basis-function networks. Neural Computation, 5, 305–
316.
Eberhart, R.C., Kennedy, J., 1995. Particle swarm
optimization. In: Proc. of IEEE Int. Conf. on Neural
Network, Perth, Australia (1995) 1942–1948.
Yousef, R., 2005. Training radial basis function networks
using reduced sets as center points. International
Journal of Information Technology, Vol. 2, pp. 21.
Zhang, J.-R., Zhang, J., Lok, T., Lyu, M., 2007. A hybrid
particle swarm optimization, back-propagation
algorithm for feed forward neural network training.
Applied Mathematics and Computation 185, 1026–
1037.
Poli, R., Kennedy, J., Blackwell, T., 2007. Particle swarm
optimization. An overview. Swarm Intelligence 1, 33–
57.
Pedrycz, W., Park, H.S., Oh, S.K., 2008. A Granular-
Oriented Development of Functional Radial Basis
Function Neural Networks. Neurocomputing, 72, 420–435.
SIMULTECH2014-4thInternationalConferenceonSimulationandModelingMethodologies,Technologiesand
Applications
450