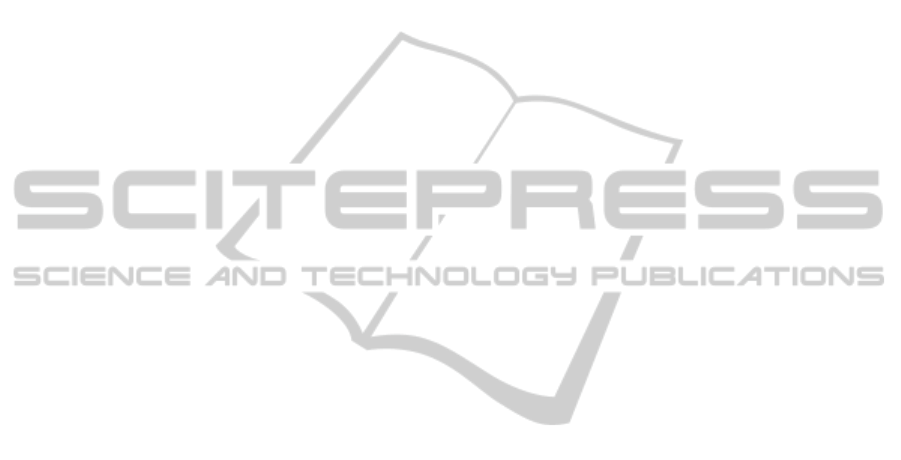
REFERENCES
Anna, G., George, and Kethsy, P., T. .
Anush, K. and Alan, C. (2010). A two-step framework for
constructing blind image quality indices. Int.J IEEE
Signal Processing Letters, 17(5):513–516.
Astola, J. and Kuosmanen., P. (1997). Fundamental of non-
linear digital filtering. CRC Press, Boca Raton, FL.
United States of America.
Bogdan, S. (2010). Peer group switching filter for impulse
noise reduction in color images. Int. J. Pattern Recog-
nition Letters, 133:484–495.
Buades, A., Coll, B., and Morel, J. (2005). A review of
image denoising algorithms with a new one. Multi-
scale Modelling Simulation, 4:490–530.
Chan, T., Esedoglu, S., Park, F., and Yip, M. (2005). Re-
cent developments in total variation image restoration.
Mathematical Models of Computer Vision.
Church, J., Yixin, C., and Rice, S. (2008). A spatial me-
dian filter for noise removal in digital images. IEEE
Southeastcon, pages 618–623.
Fitri, U., Keichi, U., and Gou, K. (2012a). High density
impulse noise removal by fuzzy mean linear alias-
ing window kernel. IEEE International Conference
Signal Processing Communication and Computing,
pages 711–716.
Fitri, U., Keichi, U., and Gou, K. (2012b). Optimization
gaussian noise removal using hybrid filter based on
mean impulse fuzzy and fuzzy aliasing filter meth-
ods. IEEJ Transactions on Electronics, Information
and Systems, 133(1):150–158.
Jian, F., Raymond, H., and Mila, N. (2008). Two-phase
methods for deblurring images corrupted by impulse
plus gaussian noise. Inverse Problem Imaging, pages
187–204.
Jian, F., Raymond, H., and Mila, N. (2010). Fast two-
phase image deblurring under impulse noise. Journal
of Mathematical Imaging and Vision, 36:46–53.
Laskar, R., Bhowmicks.B., Biswas.R., and Kar, S. (2009).
Removal of impulse noise from color image. IEEE
Region 10 TENCON, pages 1–5.
Lezoray, O., Ta, V., and Elmoataz, A. (2008). Impulse noise
spectral clustering and regulation on graph. IEEE In-
ternational Conference on Pattern Recognition, pages
1–4.
Michael, E. and Michal, A. (2006). Image denois-
ing via sparse and redundant representations over
learned dictionaries. IEEE Transactions on Image
ProcessingIEEE Transactions on Image Processing,
15(12):3736–3745.
Michal, A. and Michael, E. (2006). Alfred,b.:k-svd an al-
gorithm for denoising overcomplete dictionaries for
sparse representation. IEEE Transactions on Image
Processing, 54(11):4311–4322.
Tony, F. and Ke, C. (2006). An optimization-based mul-
tilevel algorithm for total variation image denoising.
SIAM Journal of Multi scale Modelling and Simula-
tion, 5:615–645.
Veerakumar, T., Esakkirajan, S., and Ila., V. (2013). Edge
preserving adaptive anisotropic diffusion filter ap-
proach for the suppression of impulse noise in images.
In Press Int. J. Electron. Commun. (AEU).
Wenbin, L. (2007). An efficient algorithm for the removal of
impulse noise from corrupted images. Int. J. Electron.
Commun. (AEU), 61:551–555.
Yingyue, Z., Zhongfu, Y., and Yao, X. (2013). A restoration
algorithm for images contaminated by mixed gaussian
plus random-valued impulse noise. Int.J. Vis Com-
mun. Image Representation, 24:283–294.
Zhou, W., Bovik, A.C Sheikh, H., and Simoncelli, E.
(2004). Image quality assessment: From error mea-
surement to structural similarity. Int.J IEEE Image
Processing, 13:600–612.
SIGMAP2014-InternationalConferenceonSignalProcessingandMultimediaApplications
106