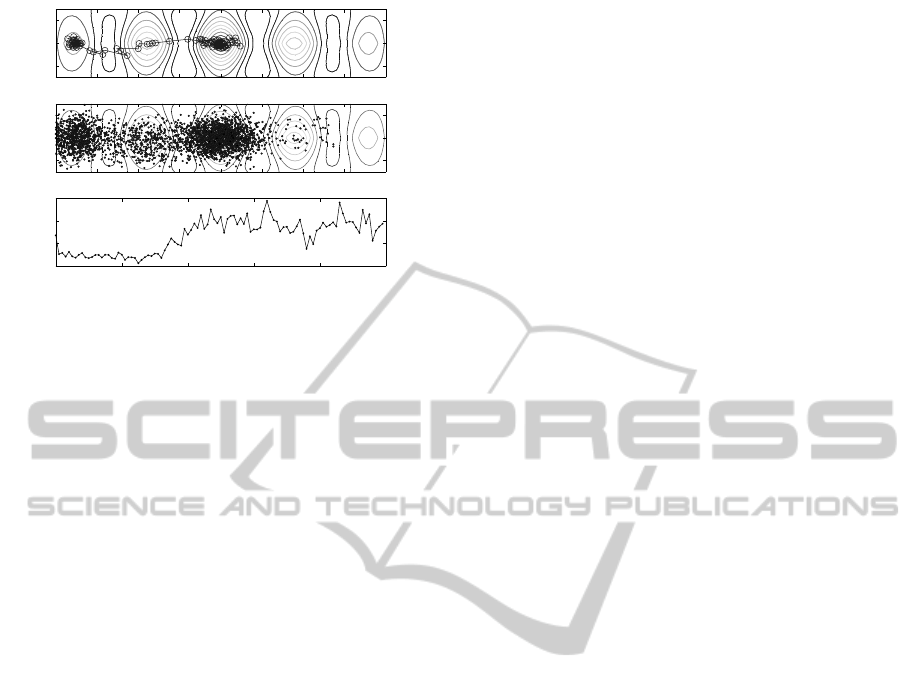
−2 −1.5 −1 −0.5 0 0.5 1 1.5 2
−1
0
1
−2 −1.5 −1 −0.5 0 0.5 1 1.5 2
−1
0
1
0 20 40 60 80 100
0
0.5
1
1.5
Figure 15: Ursem function optimization with the operator
of impatience without knowledge. Upper panel: population
mean location; middle panel: paths of all individuals; lower
panel: average fitness; m = 32, n = 2, σ = 0.2.
5 CONCLUSIONS
In this paper a mechanism of impatience was checked
as a method to preserve diversifiction of a popula-
tion and thereby escaping a local optimum trap. Two
versions of the impatience operator were examined:
with and without extra knowledge concerning (esti-
mated) position of a local optimum. Both versions
increase diversity of population. However, when an
impatience is related to a current population mean, the
polarization of a population was observed (a popula-
tion is divided into dipol-like sub-populations). When
the impatience operator is related to a local optimum
placement the polarization effect was not observed.
In contrary to other diversity preserving methods
(fitness sharing and clearing), the impatience mecha-
nism is parameter-free and increases a computational
effort only slightly. The mechanism is related to a cur-
rent population state and/or already explored parts of
a search space, so it can be used in dynamical land-
scapes.
Actions of both versions of impatience operators
were tested to check the efficiency of crossing a sad-
dle between the local and the global optimum of bi-
modal multidimensional fitness functions. Both ver-
sions demonstrated high efficiency in crossing mul-
tidimensional, width and deep saddles. Preliminary
results of applying the mechanisms to more complex
test functions are promissing and they will stimulate
our future work.
ACKNOWLEDGEMENTS
I want to thank Prof. R. Galar for encouraging me to
rediscover oldies-but-goldies ideas as well as for con-
tinuous and fruitful discussions on the subject.
REFERENCES
Chen, T., He, J., Chen, G., and Yao, X. (2010). Choosing
selection pressure for wide-gap problems. Theoretical
Computer Science, 411(6):926–934.
Chorazyczewski, A. and Galar, R. (1998). Visualization of
evolutionary adaptation in R
n
. In Evolutionary Pro-
gramming VII, pages 657–668.
DeJong, K. (1975). An analysis of the behavior a class of
genetic adaptive systems. PhD thesis, University of
Michigan.
Dick, G. and Whigham, P. A. (2006). Spatially-structured
evolutionary algorithms and sharing: Do they mix? In
et al., T. W., editor, SEAL 2006, LNCSno. 4247, pages
457–464. Springer-Verlag.
Galar, R. (1989). Evolutionary search with soft selection.
Biological Cybernetics, 60:357–364.
Galar, R. and Kopciuch, P. (1999). Impatience and polar-
ization in evolutionary processes. In Proc. of KAEiOG
Conference, pages 115–122. in Polish.
Goldberg, D. and Deb, K. (1991). A comparative analy-
sis of selection schemes used in genetic algorithms.
In Foundations of Genetic Algorithms, pages 69–93.
Morgan Kaufmann.
Goldberg, D. and Richardson, J. (1987). Genetic algorithms
with sharing for multi-modal function optimisation. In
Proc. of the 2nd Int. Conf. on Genetic Algorithms and
Their Applications, pages 41–49.
Karcz-Duleba, I. (2006). Dynamics of two-element popula-
tions in the space of population states. IEEE Transac-
tions on Evolutionary Computation, 10(2):199–209.
Mengshoel, O. and Goldberg, D. (2008). The crowding ap-
proach to niching in genetic algorithms. Evolutionary
Computation, 16(3):315–354.
Obuchowicz, A. (1997). The evolutionary search with soft
selection and deterioration of the objective function.
In Proc. 6th Int. Conf. Intel. Inform. Systems, pages
288–295.
Rogers, A. and Pr¨ugel-Bennett, A. (1999). Genetic drift in
genetic algorithm selection schemes. IEEE Transac-
tions on Evolutionary Computation, 3(4):298–303.
Sareni, B. and Kr¨ahenb¨uhl, L. (1998). Fitness sharing and
niching methods revisited. IEEE Transactions on Evo-
lutionary Computation, 2(3):97–106.
Tomassini, M. (2005). Spatially structured evolutionary al-
gorithms. Springer-Verlag.
ImpatienceMechanisminSaddles'Crossing
183