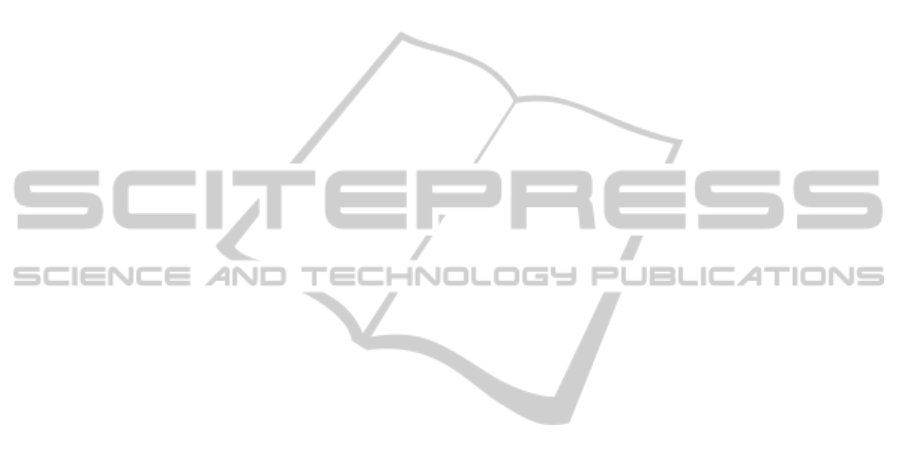
In Proc. International Conference on Security and
Cryptography (SECRYPT), pages 478–481.
Dwork, C. (2006). Differential Privacy. In Automata, Lan-
guages and Programming, volume 4052 of Lecture
Notes in Computer Science, pages 1–12. Springer.
Fung, B. and Yu, P. (2005). Top-Down Specialization for
Information and Privacy Preservation. In Proc. IEEE
ICDE, pages 205–216.
Fung, B. C. M., Wang, K., Chen, R., and Yu, P. S.
(2010). Privacy-preserving data publishing: A sur-
vey of recent developments. ACM Computing Sur-
veys, 42(4):1–53.
Ghinita, G. (2007). Fast Data Anonymization with Low
Information Loss. In Proc. VLDB, pages 758–769.
Groat, M. M., Edwards, B., Horey, J., He, W., and Forrest,
S. (2012). Enhancing privacy in participatory sens-
ing applications with multidimensional data. In Proc.
IEEE PerCom, pages 144–152.
Hu, H., Xu, J., On, S. T., Du, J., and Ng, J. K.-Y. (2010).
Privacy-aware location data publishing. ACM Trans.
Database Systems, 35(3):1–42.
Huang, Z. and Du, W. (2008). OptRR: Optimizing Random-
ized Response Schemes for Privacy-Preserving Data
Mining. In Proc. IEEE ICDE, pages 705–714.
Kabir, M., Wang, H., Bertino, E., and Chi, Y. (2010). Sys-
tematic clustering method for l-diversity model. In
ADC, volume 103, pages 93–102.
Kenig, B. and Tassa, T. (2011). A practical approximation
algorithm for optimal k-anonymity. Data Mining and
Knowledge Discovery, 25(1):134–168.
LeFevre, K., DeWitt, D., and Ramakrishnan, R. (2006).
Mondrian Multidimensional K-Anonymity. In Proc.
IEEE ICDE, pages 25–25.
LeFevre, K., DeWitt, D. J., and Ramakrishnan, R. (2008).
Workload-aware anonymization techniques for large-
scale datasets. ACM Trans. Database Systems,
33(3):1–47.
Li, N., Li, T., and Venkatasubramanian, S. (2007).
t-closeness: Privacy beyond k-anonymity and l-
diversity. In Proc. IEEE ICDE, pages 106–115.
Machanavajjhala, A., Kifer, D., Gehrke, J., and Venkita-
subramaniam, M. (2007). l-diversity: Privacy beyond
k-anonymity. ACM TKDD, 1(1):3–es.
Meyerson, A. and Williams, R. (2004). On the complexity
of optimal K-anonymity. In Proc. ACM PODS, pages
223–228.
Minnesota Population Center. Ipums,
https://www.ipums.org/.
Nergiz, A. E., Clifton, C., and Malluhi, Q. M. (2013). Up-
dating outsourced anatomized private databases. In
Proc. EDBT, page 179. ACM.
Nikolov, A., Talwar, K., and Zhang, L. (2013). The geome-
try of differential privacy: the sparse and approximate
cases. In Proc. ACM STOC, pages 351–360.
Samarati, P. (2001). Protecting respondents identities in mi-
crodata release. IEEE Trans. Knowledge and Data En-
gineering, 13(6):1010–1027.
Sun, X., Wang, H., Li, J., and Ross, D. (2009). Achieving P-
Sensitive K-Anonymity via Anatomy. In Proc. IEEE
International Conference on e-Business Engineering
(ICEBE), pages 199–205.
Sweeney, L. (2002). Achieving k-anonymity privacy pro-
tection using generalization and suppression. In-
ternational Journal of Uncertainty, Fuzziness and
Knowledge-Based Systems, 10(05):571–588.
UCI Machine Learning Repository. Adult Data Set,
http://archive.ics.uci.edu/ml/datasets/Adult.
Wu, S., Wang, X., Wang, S., Zhang, Z., and Tung,
A. K. (2013). K-Anonymity for Crowdsourcing
Database. IEEE Trans. Knowledge and Data Engi-
neering, PP(99).
Xiao, X. and Tao, Y. (2006). Anatomy: simple and effective
privacy preservation. In Proc. VLDB, pages 139–150.
Xiao, X., Yi, K., and Tao, Y. (2010). The hardness and
approximation algorithms for l-diversity. In Proc.
EDBT, pages 135–146.
Xie, H., Kulik, L., and Tanin, E. (2011). Privacy-aware col-
lection of aggregate spatial data. Data & Knowledge
Engineering, 70(6):576–595.
Yao, L., Wu, G., Wang, J., Xia, F., Lin, C., and Wang, G.
(2012). A Clustering K-Anonymity Scheme for Loca-
tion Privacy Preservation. IEICE Trans. Information
and Systems, E95-D(1):134–142.
SECRYPT2014-InternationalConferenceonSecurityandCryptography
360