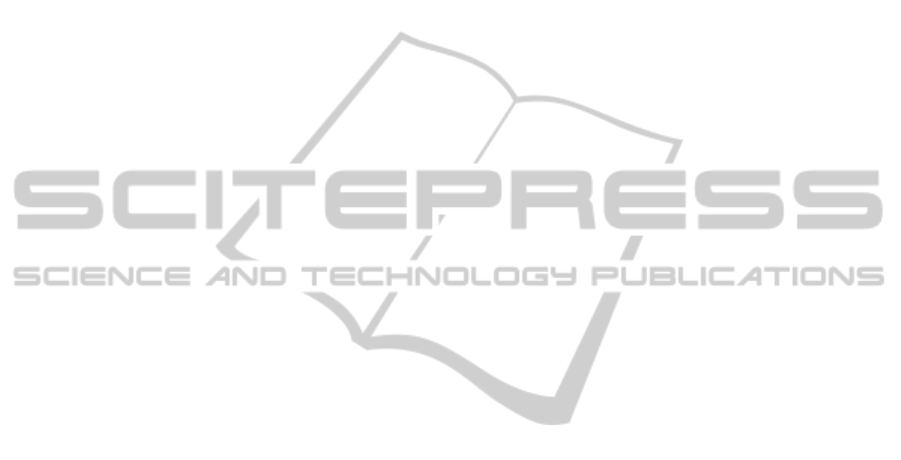
the model helps identity, classify, characterize and
evaluate data in ways that can contribute to decision
making. The characterization of data spots related to
the business process fosters understanding about the
data and their attributes fordecision supportpurposes.
The absence of such model results can lead to an in-
complete basis for decision making. The limitation
of the presented model results from the nature of pro-
cesses, which have a static character and do not com-
pletely account for customer behavior, e.g., multiple
runs through the process of information gathering.
The presented process model suggests different
opportunities for further research. The proposed
model was applied in the field of online advertis-
ing. It should also be tested in different scenarios to
determine the degree of possible generalization and
application-specific needs, particularly with regard to
the identification of the related business process. Fur-
thermore, the development of a graphical representa-
tion could foster the decision making process.
REFERENCES
Author (2013). Big Data - Characterizing an Emerging Re-
search Field using Topic Models (under review). In-
ternational Journal of Technology and Management.
Braun, M. and Moe, W. W. (2013). Online display adver-
tising: Modeling the effects of multiple creatives and
individual impression histories. Marketing Science,
32(5):753–767.
Bucklin, R. E. and Sismeiro, C. (2003). A model of web
site browsing behavior estimated on clickstream data.
Journal of Marketing Research, 40(3):249–267.
Byrd, T., Cossick, K., and Zmud, R. (1992). A synthesis of
research on requirements analysis and knowledge ac-
quisition techniques. Mis Quarterly, 16(1):117–138.
Chatterjee, P., Hoffman, D. L., and Novak, T. P. (2003).
Modeling the clickstream: Implications for web-based
advertising efforts. Marketing Science, 22(4):520–
541.
Chaudhuri, S., Dayal, U., and Ganti, V. (2001). Database
technology for decision support systems. Computer,
34(12):48–55.
Cho, C.-H. (2003). Factors influencing clicking of ban-
ner ads on the www. CyberPsychology & Behavior,
6(2):201–215.
Danaher, P. J. and Mullarkey, G. (2003). Factors affecting
online advertising recall: A study of students. Journal
of Advertising Research, 43(3):252–267.
Davis, G. B. (1982). Strategies for information require-
ments determination. IBM Systems Journal, 21(1):4–
30.
Forcada, M. L., Ginest´ı-Rosell, M., Nordfalk, J., O’Regan,
J., Ortiz-Rojas, S., P´erez-Ortiz, J. A., S´anchez-
Mart´ınez, F., Ram´ırez-S´anchez, G., and Tyers, F. M.
(2011). Apertium: a free/open-source platform for
rule-based machine translation. Machine Translation,
25(2):127–144.
Gardner, S. R. (1998). Building the data warehouse. Com-
munications of the ACM, 41(9):52–60.
Giorgini, P., Rizzi, S., and Garzetti, M. (2005). Goal-
oriented requirement analysis for data warehouse de-
sign. Proceedings of the 8th ACM international work-
shop on Data warehousing and OLAP - DOLAP,
page 47.
Golfarelli, M., Maio, D., and Rizzi, S. (1998). The dimen-
sional fact model: A conceptual Model for Data Ware-
houses. International Journal of Cooperative Infor-
mation Systems, 7(2-3):215–247.
Hevner, A. R., March, S. T., Park, J., and Ram, S. (2004).
Design science in information system research. MIS
Quarterly, 28(1):75–105.
Hilbert, M. and L´opez, P. (2011). The world’s technolog-
ical capacity to store, communicate, and compute in-
formation. Science (New York, N.Y.), 332(60):60–65.
Inmon, W. H. (2005). Building the Data Warehouse. John
Wiley & Sons, Indianapolis, 4th edition.
Kotonya, G. and Sommerville, I. (1998). Requirements En-
gineering: Processes and Techniques. John Wiley &
Sons.
LaValle, S., Lesser, E., and Shockley, R. (2011). Big Data,
Analytics and the Path From Insights to Value. MIT
Sloan Management Review, 52(2):21–31.
List, B., Schiefer, J., and Tjoa, A. (2000). Process-oriented
requirement analysis supporting the data warehouse
design process a use case driven approach. In DEXA
’00 Proceedings of the 11th International Conference
on Database and Expert Systems Applications, pages
593–603.
Madnick, S. E., Wang, R. Y., Lee, Y. W., and Zhu, H.
(2009). Overview and Framework for Data and In-
formation Quality Research. ACM Journal of Data
and Information Quality, 1(1):1–22.
Manyika, J., Chui, M., Brown, B., Bughin, J., Dobbs, R.,
Roxburgh, C., and Byers, A. H. (2011). Big data : The
next frontier for innovation , competition , and pro-
ductivity. Technical Report June, McKinsey Global
Institute.
Maz´on, J., Pardillo, J., and Trujillo, J. (2007). A
model-driven goal-oriented requirement engineering
approach for data warehouses. In Advances in Con-
ceptual Modeling – Foundations and Applications,
pages 255–264. Springer, Berlin Heidelberg.
Moody, D. L. and Kortink, M. A. R. (2000). From Enter-
prise Models to Dimensional Models : A Methodol-
ogy for Data Warehouse and Data Mart Design Ob-
jectives of Dimensional Modelling. In 2nd DMWD,
volume 2000.
Morrison, D. G. (1969). On the interpretation of dis-
criminant analysis. Journal of Marketing Research,
6(2):156–163.
Mudambi, S. and Schuff, D. (2010). What makes a helpful
online review? A study of customer reviews on Ama-
zon. com. MIS quarterly, 34(1):185–200.
Nottorf, F. (2013). Modeling the clickstream across mul-
tiple online advertising channels using a binary logit
DecisionSupportandOnlineAdvertising-DevelopmentandEmpiricalTestingofaDataLandscape
121