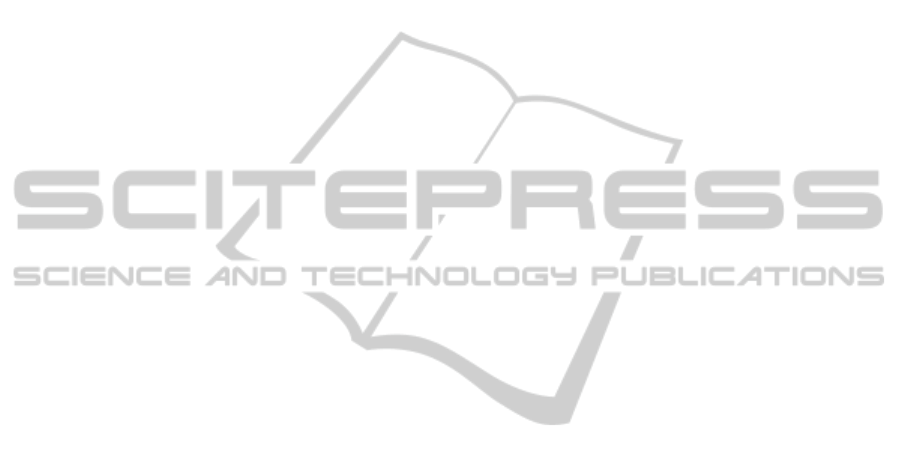
2001) and the one here proposed respectively. The
method A is shortly reported in the Appendix for sake
of notation consistency.
4.2 Estimated Parameters
Table 1 reports the characteristics of the two opti-
mal trajectories implemented from the results of the
two algorithms A and B. As expected, A provides a
higher-determinant trajectory, while its coupling in-
dex is poorer. The trajectory from B, in fact, achieves
a much lower correlation influence among EP, see
Figure 2. Both optimal trajectories have been then
executed 30 times each, and the EP have been esti-
mated in each repetition through eq. (4), so that the
EP reported in Table 2 are the mean over the 30 rep-
etitions of the 30 different random trajectories. Fur-
thermore, in Table 2 the fourth column, indicated as
π
C
, lists the CAD data provided from the robot manu-
facturer from 1 to 40 and the friction values identified
from COMAU from 41 to 58 (the parameters from 53
to 58 are zero because COMAU has a linear model
of the friction). COMAU declares a not negligible
variability in the link weight about the 5%-10% due
to the inaccuracy of the casting process. Looking at
parameters from 1 to 22 and from 33 to 58, the Algo-
rithm B identifies values that are averagely closer to
CAD (except parameter 3, 15 and 46). On the other
hand, looking at the parameters from 23 to 32, that are
the complex aggregates parameters, the Algorithm A
identifies values averagely closer to CAD. This dif-
ference should derive from the numerical procedures
used for the EP selection (Antonelli et al., 1999). In-
deed, different thresholds may aggregate BP around
different EP. This should indicate that the method here
presented should be better applied to the identifica-
tion of BP set introducing a priori knowledge of the
system to overcome the issues on the observability.
Future works will be done on this topic.
4.3 Torque Prediction Power
The prediction power of the estimated parameters has
been validated by 30 different random test trajecto-
ries generated through the ORL library. The trajecto-
ries were wide, covering the entire workspace. The
mean error in the torque prediction has been calcu-
lated for each repetition and for each axis. Figure 3
displays the statistics (median/quartile) of the distri-
bution of mean error of each axis over the 30 repe-
titions. Figure 4 displays the results of joint torques
reconstruction for one paradigmatic experiment. Av-
eragely, the algorithm B provide lower prediction er-
ror for all axes.
5 CONCLUSION
The paper has introduced a novel index to estimate the
average coupling of the trajectory in term of correla-
tion among the essential parameters. Two different al-
gorithm have been implemented and compared, test-
ing their performances. Experimental results demon-
strate how a decoupling trajectory produce a better
estimation. Future works will focused on the prop-
agation of the covariance taking into account that the
dynamic regressor Φ
Φ
Φ is not free of noise. Further-
more, a deep analysis of the physical meaning of the
essential parameters will be investigated.
ACKNOWLEDGMENT
R. Bozzi, and J. C. Dalberto, laboratory technicians
of CNR-ITIA, have been involved in setting up the ex-
periments. This work is partially within FLEXICAST
funded by FP7-NMP EC.
REFERENCES
Antonelli, G., Caccavale, F., and Chiacchio, P. (1999).
A systematic procedure for the identification of dy-
namic parameters of robot manipulators. Robotica,
17(04):427–435.
Atkeson, C. G., An, C. H., and Hollerbach, J. M. (1986).
Estimation of inertial parameters of manipulator loads
and links. Int J of Rob Res, 5(3):101–119.
Benimeli, F., Mata, V., and Valero, F. (2006). A compar-
ison between direct and indirect dynamic parameter
identification methods in industrial robots. Robotica,
24(5):579–590.
Caccavale, F. and Chiacchio, P. (1994). Identification of dy-
namic parameters and feedforward control for a con-
ventional industrial manipulator. Control Engineering
Practice, 2(6):1039–1050.
Calafiore, G., Indri, M., and Bona, B. (2001). Robot dy-
namic calibration: Optimal excitation trajectories and
experimental parameter estimation. J. of Robotic Sys-
tems, 18(2):55–68.
COMAU Robotics (2010). Open realistic robot library.
Gautier, M. (1997). Dynamic identification of robots with
power model. In Rob. and Aut., Proc., IEEE Int. Conf.
on, volume 3, pages 1922 –1927.
Gautier, M. and Khalil, W. (1988). On the identification of
the inertial parameters of robots. In Dec and Contr,
Proc. of IEEE Conf. on, volume 3, pages 2264 –2269.
Gautier, M. and Venture, G. (2013). Identification of stan-
dard dynamic parameters of robots with positive def-
inite inertia matrix. In Intell Rob and Sys, 2013
IEEE/RSJ Int Conf on, pages 5815–5820.
Indri, M., Calafiore, G., Legnani, G., Jatta, F., and Visioli,
A. (2002). Optimized dynamic calibration of a scara
RobotDynamicModelIdentificationThroughExcitationTrajectoriesMinimizingtheCorrelationInfluenceamong
EssentialParameters
481