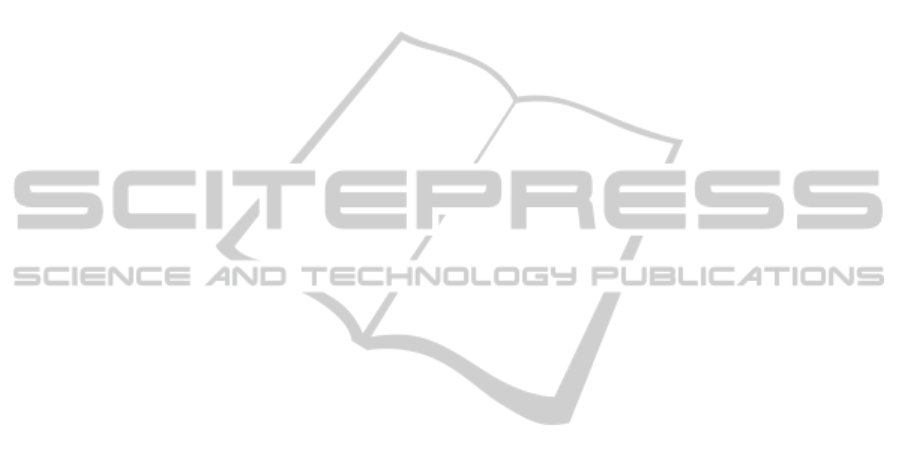
remarkable shift in how smart thermal control has
been considered till these days. Comparing the EQM
based
smart thermal control efficiency with
commonly used approaches (based on white, grey or
black thermal models), is unbalanced considering
their different application conditions. In fact, trying
to operate an MPC (Model Predictive Control) in
few days on a completely unknown building is not
conceivable. It goes the same when asking the EQM
based control for the same efficiency as a MPC
based control in a fully identified building’s thermal
process. Yet, perspectives remain possible to
improve RIDER STC efficiency. For the CIPPD
based
smart thermal control, adaptive and dynamic
thermal comfort model could be considered in order
to ensure more personalized and individualized
thermal comfort adjustment. Yet, the PPD’s choice
satisfies the data unavailability issues. The transition
toward an adaptive and dynamic thermal comfort
model shall, thus, be supported by
online learning
techniques. The CIPPD identification could also be
improved by using bipolar utility scale wish gives
more expressivity to the thermal comfort models and
could lead to a better approximation of the PPD
function. The EQM based
smart thermal control
provides a methodology in order to improve the
qualitative based thermal control efficiency.
Therefore, each step implementation technique
could be discussed. For instance, uncertainty
management in influence functions can be improved.
Ambiguous measurements coming from thermal
disturbances (
i.e., windows and door opening)
should complete this point. Sensors data precision
can be studied as well. Qualitative interactions
between the control enhancement parameters could
also be studied in order to compute enhancement
recommendations based on subsets of control
parameters variations instead of singletons. This will
warrantee the EQM control convergence to a global
improved control experience
rather than a local one.
The scalability of the RIDET STC solution could
also be discussed. In fact, we have shown in section
3.2.3 that multi-room transition needs some settings
and it goes the same for any scale transition. The
scalability could have been made automatic by
providing different scales templates in the RIDER
STC final solution. This task is simplified thanks to
the EQM modularity.
REFERENCES
Aydinalp, M., Ugursal, V.I., Fung, A.S., 2002. Modeling
of the appliance, lighting, and sapce-cooling enrgy
consumptions in the residential sector using neural
networks, Applied Energy, vol. 2, n°71, pp. 87-110.
Calvino, F., Gennusca, M.L., Rizzo, G., Scaccianoce, G.,
2004. The control of indoor thermal comfort
conditions: introduicing a fuzzy adaptive controller,
Energy and Buildings, vol. 36, pp. 97-102.
Denguir, A., Trousset, F., Montmain, J., 2012. Comfort as
a Multidimensional Preference Model for Energy
Efficiency Control Issues, In SUM 2012, pp. 486-499.
Denguir, A., Trousset, F., Montmain, J., 2014.
Approximate Reasoning for an Efficient, Scalable and
Simple Thermal Control Enhancement, IN IPMU,
Montpellier.
Dong, B., Cao, C., Lee, S.E., 2005. Applying support
vector machines to predict building energy
consumption in tropical region,Energy and Buildings,
vol. 5, n°37, pp. 545-553.
Dounis, A.I., Santamouris, M.J., Lefas, C.C,. Argirious,
A., 1995. Design of a fuzzy set environment comfort
system, Energy and Buildings, vol. 1, n°22, pp. 81-87.
Dubois, D., 1989. Order of magnitude reasoning with
fuzzy relations, Artificial Intelligence, vol. 3, n°14, pp.
69-94.
European Parliament and Council (EP&C)., 2012.
Directive 2012/27EU of European Parliament and
Council of 27 October 2012 on the energy
performance of buildings, Official Journal of the
European Union, pp. 1-56.
Fanger, P.O., 1967, Calculation of Thermal Comfort:
Introduction of a basic Comfort Equation. ASHRAE
Trans. Vol. 73.
Fishburn, P.C. 1970. Utility Theory for Decision-Making.
John Wiley & Sons, New York.
Gagge, A.P., Fobelets, A.P., Berglund, L.G., 1986. A
Standard Predictive Index of Human Response to the
Thermal Environment. ASHRAE Trans, vol. 92.
Grabisch, M. k-Ordered Discrete Fuzzy Measures and
Their Representation. In: Fuzzy sets and systems, vol.
92, pp. 167-189. (1997).
Kalogirou, S.A., Bojic, M., 2000. Artificial neural
networks for the prediction of the energy consumption
of a passive solar building, Energy, vol. 5, n°25, pp.
479-491.
Krantz, D.H., Luce, R.D., Suppes, P., Tversky, A. 1971.
Foundations of measurement, Additive and Poly-
nomial Representations, vol. 1. Academic Press.
Kuipers, B., 1986. Qualitative simulation, Artificial
Intelligence, vol. 29, pp. 289-388.
Labreuche, C.2011. Construction of a Choquet integral
and the value functions without any com-
mensurateness assumption in multi-criteria decision
making. In EUSFLAT-LFA, Aix-les-Bains, France.
Li, Q., Meng, Q.L., Cai, J.J., Hiroshi, Y., Akashi, M.,
2009. Applying support vector machine to predict
hourly cooling load in the building, Applied Energy,
vol. 10, n°86, pp. 2249-2256.
Ma, Y., Borrelli, F., Hencey, B., Packard, A., Bortoff, S.,
2009. Model predictive control of thermal energy
storage in building cooling systems. In Conference on
AggregatedPerformanceandQualitativeModelingBasedSmartThermalControl
75