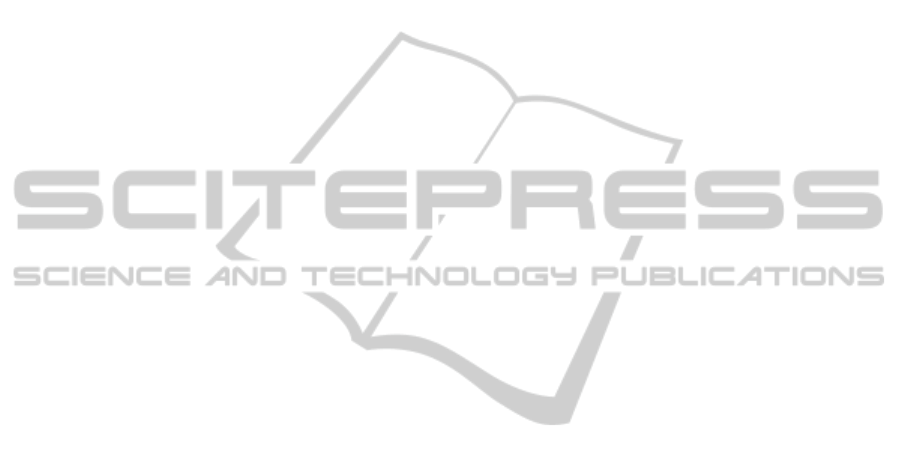
It was observed that for a particular data sequence,
usually one or two structures of dynamic models
yielded a sufficient number of significant compo-
nents. This property indicates that the method might
“choose” the suitable dynamic features for the given
data. However, it requires further testing.
4.2 Open Problems
Generic aiming and flexibility of the described
method is balanced by its limited performance. Prac-
tical applicability of the method may be enhanced by
considering several topics, among others
• assuming bounded noise rather than Gaussian,
• reconsidering advantages and disadvantages of
dynamic component models,
• validating of the mixture by other means than con-
vergence of its transition table,
• trying a better criterion for comparison (e.g.
Kullback-Leibler divergence of Dirichlet pdfs,
dynamic clustering in parameter space etc.)
• employing components of different dynamic or-
der,
The QB approximation is more consistent with the
Bayesian methodology used in this work than the ML
one. Therefore, fixing the convergence issue in case
of QB approximation is desirable.
As the method is unsupervised in the described
phase, no critical value of
¯
ρ was set up. The question
is how to make this set up methodically and gener-
ally. The method is based on occurrence of specific
features with a specific set of data sequences and ad-
equate model. Stability of these features within the
set indicates data similarity. The question is whether
these features can be extracted by the method or
added externally by an expert.
The supervision is a crucial topic. It might im-
prove the performance and set the critical value of
¯
ρ
but, on the other hand, lead to tailored solutions with
loss of intended generality.
Other models than AR or other technology could
be taken into account as well.
Last but not least, the method is based on extract-
ing information from data. Hence, a wider set of data,
including those with real faults, should be studied.
ACKNOWLEDGEMENTS
This research has been supported by EUROSTARS
Project 7D12004 E!7662.
REFERENCES
Bo
ˇ
skoski, P., Ga
ˇ
sperin, M., and Petelin, D. (2013). Bearing
fault prognostics using R
´
enyi entropy based features
and Gaussian process models. Mechanical Systems
and Signal Processing. Submitted.
Dedecius, K. and Jirsa, L. (2010). Bayesian Soft Sensing in
Cold Sheet Rolling. In Proc. of the 6th Int. Workshop
on Data-Algorthms-Decision Making. UTIA AV
ˇ
CR.
Ettler, P. and Dedecius, K. (2013). Overview of bounded
support distributions and methods for Bayesian treat-
ment of industrial data. In Proceedings of the 10th
international conference on informatics in control,
automation and robotics (ICINCO), pages 380–387,
Reykjavik, Iceland.
Ettler, P., Juricic, D., Dedecius, K., and Preglej, A. (2011).
Advanced soft sensor technology to be used for cold
rolling mills. In Emerging Technologies & Factory
Automation (ETFA), 2011 IEEE 16th Conference on,
pages 1–4. IEEE.
Ettler, P., Puchr, I., and Dedecius, K. (2013). Bayesian
model mixing for cold rolling mills: Test results. In
Fikar, M. and Kvasnica, M., editors, Proceedings of
the 2013 International Conference on Process Control
(PC), pages 359–364,
ˇ
Strbsk
´
e Pleso, Slovakia.
He, X., Wang, Z., and Zhou, D. H. (2008). State estima-
tion for time-delay systems with probabilistic sensor
gain reductions. Asia-Pacific Journal of Chemical En-
gineering, 3(6):712–716.
Isermann, R. (2011). Fault Diagnosis Applications: Model
Based Condition Monitoring, Actuators, Drives, Ma-
chinery, Plants, Sensors, and Fault-tolerant Systems.
Springer Verlag.
Jirsa, L., Pavelkov
´
a, L., and Dedecius, K. (2013). Pre-
liminaries of probabilistic hierarchical fault detection.
In Preprints of the 3rd International Workshop on
Scalable Decision Making held in conjunction with
ECML/PKDD 2013, pages 1–14, Prague, Czech re-
public.
K
´
arn
´
y, M., B
¨
ohm, J., Guy, T. V., Jirsa, L., Nagy, I., Ne-
doma, P., and Tesa
ˇ
r, L. (2005). Optimized Bayesian
Dynamic Advising: Theory and Algorithms. Springer,
London.
Lu, F., Huang, J., and Xing, Y. (2012). Fault diagnostics
for turbo-shaft engine sensors based on a simplified
on-board model. Sensors, 12(8):11061–11076.
Nagy, I. (2014). Advanced statistical methods
and their applications.
ˇ
CVUT FD, Prague.
http://www.fd.cvut.cz/personal/nagyivan/PhDLoad/
LecturesPhD.pdf, In Czech.
Nagy, I., Suzdaleva, E., K
´
arn
´
y, M., and Mlyn
´
a
ˇ
rov
´
a, T.
(2011). Bayesian estimation of dynamic finite mix-
tures. Int. Journal of Adaptive Control and Signal
Processing, 25(9):765–787.
Rajaman, R. and Ganguli, A. (2004). Sensor fault diag-
nostics for a class of non-linear systems using linear
matrix inequalities. International Journal of Control,
77(10):920–930.
Walambe, R., Gupta, N., Bhange, N., Ananthkrishnan, N.,
Choi, J., Park, I., and Yoon, H. (2010). Novel redun-
TestingofSensorConditionUsingGaussianMixtureModel
557