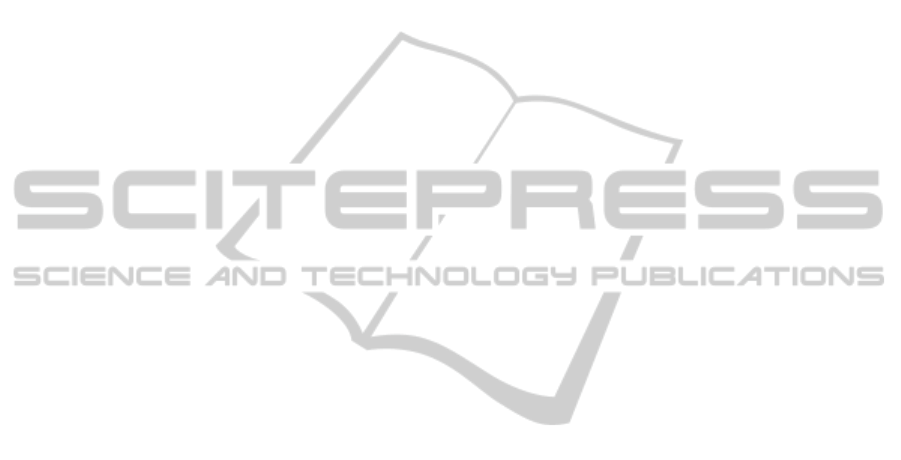
Therefore, we have counted such cases and the result
is depicted in Fig. 5. The worst efficiency in terms of
missed change points has been observed for features
7–11 (Tab. 1). The features exploited in our study
represents the properties of frequency distribution of
the input signals at different frequency scales. Con-
sequently, due to the fact that the input data in our
study contains mostly speech, the features which ex-
hibit the variability details of speech signal have led
to the most promising results.
In the most cases the fact of change point detection
is more important than the obtained accuracy. Thus,
the selection of feature vector size together with the
selection of the feature type is significant for the fi-
nal performance of the segmentation process. Also,
the parametrization stage should be carefully config-
ured for the expected types of audio segments and the
target application.
5 CONCLUSIONS
In this paper an analysis of auditory features effi-
ciency for BIC-based audio segmentation has been
performed. For several examples 12 feature sets have
been examined. As the result, the features BFB,
LFCC, GFCC, GTACI and the GTEMX give promis-
ing results and they are competitive to the MFCC fea-
ture widely used in many audio segmentation sys-
tems. Due to the variability of the content in seg-
ment boundaries, better results seem to be achieved
in case of using joint different features. Also, in typ-
ical segmentation algorithm, an analysis window se-
lection and moving strategy have an important influ-
ence on the segmentation results. Furthermore, the
fusion and clustering methods of the obtained change
points may improve significantly the result for sig-
nals with several audio classes. Finally, an analysis of
features based on cochleagram and correlogram with
generalized likelihood ratio (GLR) and Hotteling’s T
2
trajectories is the future subject.
ACKNOWLEDGEMENTS
This work was sponsored by the Polish National
Science Center under a research project for years
2011-2014 (grant No. N N516 492240).
REFERENCES
Castan, D., Ortega, A., Villalba, J., Miguel, A., and
Lleida, E. (2013). Segmentation-by-classification sys-
tem based on factor analysis. In IEEE International
Conference on Acoustics, Speech and Signal Process-
ing (ICASSP), pages 783–787.
Cettolo, M. and Vescovi, M. (2003). Efficient audio seg-
mentation algorithms based on the bic. In IEEE Inter-
national Conference on Acoustics, Speech and Signal
Processing (ICASSP 2003).
Chen, S. and Gopalakrishnan, P. (1998). Speaker, envi-
ronment and channel change detection and clustering
via the bayesian information criterion,. In In Proc.
DARPA Broadcast News Transcription and Under-
standing Workshop.
Cheng, S. and Wang, H. (2003). A sequential metric-based
audio segmentation method via the bayesian informa-
tion criterion. In Proceedings EUROSPEECH 2003,
Geneva, Switzerland.
Cheng, S., Wang, H., and Fu, H. (2008). Bic-based audio
segmentation by divide-and-conquer. In IEEE Inter-
national Conference on Acoustics, Speech and Signal
Processing (ICASSP 2008).
Cooke, M. (2005). Modelling Auditory Processing and Or-
ganisation. Cambridge University Press.
Foote, J. and Cooper, M. (2003). Media segmentation using
self-similarity decomposition. In SPIE Storage and
Retrieval for Multimedia Databases, volume 5021,
pages 167–175.
Garofolo, J., Fiscus, J., and Le, A. (2004). 2002 Rich Tran-
scription Broadcast News and Conversational Tele-
phone Speech. Linguistic Data Consortium.
Ghitza, O. (1994). Auditory models and human perfor-
mance in tasks related to speech coding and speech
recognition. IEEE Transactions on Speech Audio Pro-
cessing, 2:115–132.
Rabiner, L. and Schafer, W. (2010). Theory and Applica-
tions of Digital Speech Processing. Prentice-Hall, 1st
edition.
Shao, Y. and Wang, D. (2009). Robust speaker identifica-
tion using auditory features and computational audi-
tory scene analysis. In IEEE International Conference
on Acoustics, Speech and Signal Processing (ICASSP
2009).
Smith, J. (2011). Spectral Audio Signal Processing. W3K
Publishing, 1st edition.
Wang, D. and Brown, G. J. (2006). Computational Auditory
Scene Analysis. John Wiley & Sons, Inc., 1st edition.
Wu, C. and Hsieh, C. (2006). Multiple change-point audio
segmentation and classification using an mdl-based
gaussian model. IEEE Transactions on Audio, Speech,
and Language Processing, 14(2).
Xue, H., Li, H., Gao, C., and Shi, Z. (2010). Computation-
ally efficient audio segmentation through a multi-stage
bic approach. In 3rd International Congress on Image
and Signal Processing (CISP2010).
AuditoryFeaturesAnalysisforBIC-basedAudioSegmentation
53