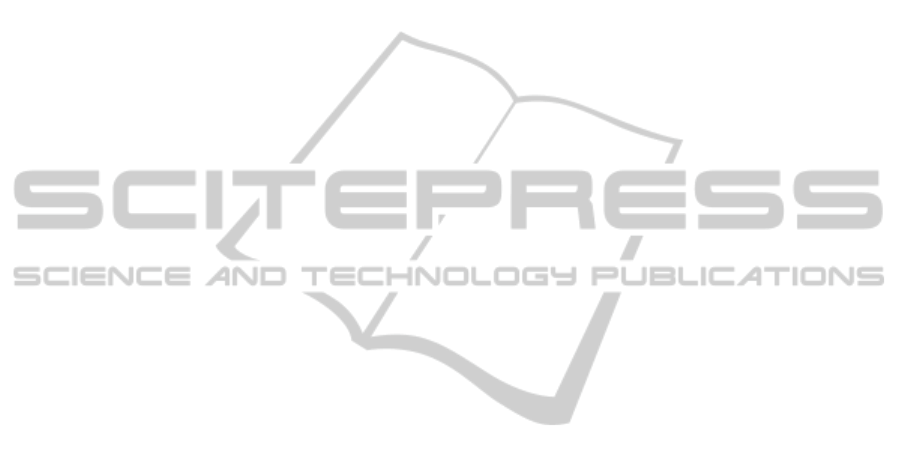
work in this area has considered online content of Ji-
hadists and their supporters (Conway, 2006) with a
particular emphasis on the content of Jihadi videos
including various types of videos and their impact on
their audiences (Salem et al., 2006), (Kimmage and
Ridolfo, 2007), (Salem et al., 2008).
For example, the HBO documentary called Bagh-
dad ER (Baghdad, 2006), which dealt with the sub-
ject of providing emergency medical care to wounded
US personnel in the battlefield, was re-created by Al-
Qaeda based in Mesopotamia by replacing the origi-
nal soundtrack with their own, and by making an en-
tirely different beginning and ending to show that the
US forces are sustaining loses and being defeated in
combat.
Similarly, in Iraq and Afghanistan, terror groups
use videos to demonstrate their victories over the op-
posing side. Viewer-enthusiasm is gauged by how
quickly the video spreads over the internet and into
news media sites (Farwell, 2010). Because of this un-
conventional approach, they have a greater chance of
getting news coverage via satellite TV which has a
large number of viewers in the Arab world and simi-
lar conflict zones.
The main idea is to identify the impact of videos
to the audiences. Funders and policy makers have
shown an increased interest in learning the ways of vi-
olent radicalization (Council of the European Union,
2005). A high profile example is Hussain Osman, one
of the London bombers, who claimed to have been
influenced by watching videos of the conflict in Iraq
along with reading about jihad in an online forum.
In this paper, we create a network of YouTube
video uploaders to investigate the possibilities of rad-
icalization via the Internet specifically from YouTube
as opposed to analysis of jihadist sites. We aim to find
the users that are registered on YouTube and have up-
loaded videos and/or have commented on video con-
tent related to a given context. Hence, our work is
closely related to the work of (Chen et al., 2003; Wen
et al., 2007; Das et al., 2008).
With respect to data collection, analysis, and vi-
sualization, Coplink (Chen et al., 2003) was one of
the first systems to successfully address the domain of
criminal network investigation. The system was first
deployed at the Tucson Police Department. The sys-
tem collects, combines, and analyzes data from vari-
ous sources and generates overviews of the informa-
tion for the investgators to help them solve cases.
With respect to the idea of context, (Wen et al.,
2007) presents an intelligent information system that
performs an investigation task for detecting frauds.
The authors have contributed by developing two no-
tions: 1. Context and 2. Context-awareness. Re-
lated to context, the paper defines the term investiga-
tion context and with regard to context-awareness the
investigator can adaptively retrieve data and evaluate
the relevant information for the ongoing investigation.
With respect to collection of user networks from
social media, (Das et al., 2008) focuses on improv-
ing performance in information collection of a so-
cial graph of users’ neighbours in a dynamic social
network. In the study, the author has introduced a
sampling based algorithm for quickly approximating
quantities of interest, the vicinity of a user’s social
graph that explores the variants of correlation across
the sample. The algorithm can be used to rank the
items in the neighbourhood of a user.
Pippal et al. (Pippal et al., 2014) provide a recent
survey of data mining approaches and methods in so-
cial networking sites, including micro-blogging, twit-
ter, YouTube, instagram, blogs, forums, etc. Closely
related work is done by Agarwal and Sureka (Ag-
garwal and Sureka, 2014) where the authors analyse
YouTube metadata for privacy invading and harrass-
ment content. He et al. model user comments on
YouTube videos as a bipartite graph to predict the
popularity of videos and other item on the Web. (He
et al., 2014)
The purpose of our study is to develop a tool for
context-based monitoring of social media data based
on a set of monitoring metrics. To our knowledge, no
one has defined monitoring metrics for YouTube with
the intent to develop a tool to explore and monitor
influential videos, users, and networks.
4 THE YOUTUBE MONITORING
TOOL
The YouTube monitoring tool is a part of our frame-
work entitled ”Keyword-based Social Network Anal-
ysis Framework” (KSNAF). KSNAF aims at support-
ing collection, monitoring, and mining of social me-
dia data from a contextual perspective. Overall, the
framework must meet the following requirements:
1. The framework enables context-based search. In
the case of YouTube, the framework provides fa-
cilities to collect videos that match a given context
based on keywords defined by the investigator.
2. The framework determines relationships among
the users that are retrieved as the result of a
context-based search. In the case of YouTube, the
framework can build a social network based on
the users’ activities (comments on videos).
3. The framework enables investigators to view
data related to a given set of monitoring met-
AToolforMonitoringofYouTubeContent
173