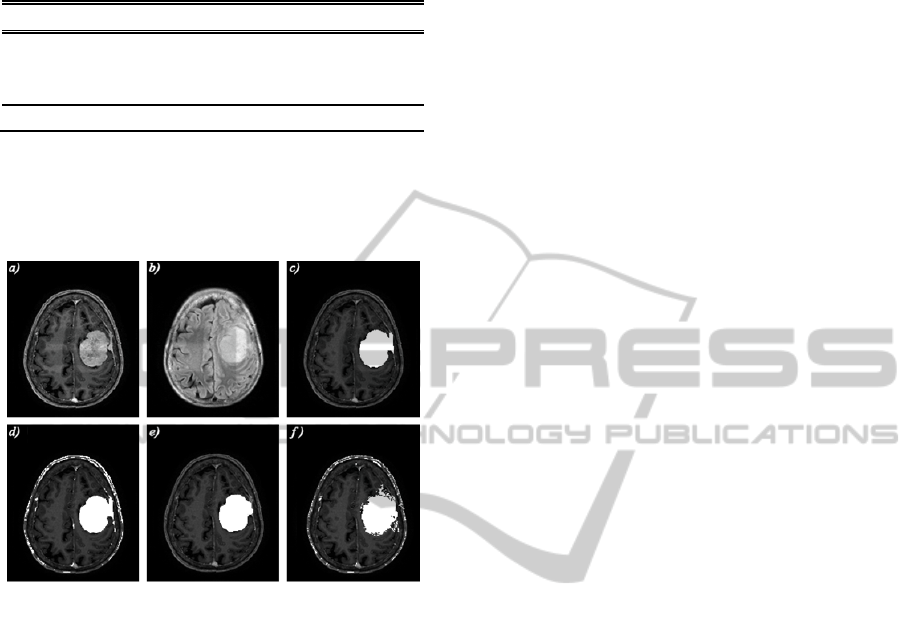
Table 2: Williams’ Indexes obtained by considering the
manual segmentations (E1-E3) and the automatic
segmentation (A) of the 10 cases under study.
W. I. 1 2 3 4 5 6 7 8 9 10
E1 1.0 1.0 1.0 1.0 1.0 0.9 0.9 1.0 0.9 1.0
E2 0.9 0.9 1.0 0.9 0.9 1.0 1.0 1.1 0.9 0.9
E3 0.9 1.0 0.9 1.0 0.9 1.0 1.0 0.7 1.0 1.0
A 1.0 1.0 1.0 1.0 1.0 1.0 1.0 1.1 1.0 1.0
indicate that the automatic segmentation method has
a good behavior with respect to the inter-expert
variability and often it shows a better behavior than
other raters.
Figure 1: Results of one axial slice obtained by the
interpatient analysis. a) Original T1c image, b) Original
T2f image, c) Overlap between original T1c image and the
ground truth mask, d) Overlap between original T1c image
and the intermediate mask obtained by Graph Cut
segmentation, e-f) Overlap between original T1c image
and the final segmentation mask obtained by interpatient
analysis, considering and without considering Graph Cut
stage respectively.
Figure 1 shows an axial slice of one patient and
the results obtained considering and without
considering Graph Cut stage in the overall strategy.
Results are clearly worse when not using the Graph
Cut preliminarily. For the dataset shown in Figure 1,
we obtained a Jaccard coefficient equal to 0.21109
and Volume Error equal to 363.4476 when no Graph
Cut is applied. With the complete procedure, Jaccard
is equal to 0.856 and Volume Error is equal to 7.119.
The mean computation time for the training task is
60 seconds and for the segmentation task performed
by the trained classifier is 159 seconds (without
Graph Cut, 732 s.) on a single CPU running at 2.26
Ghz.
5 CONCLUSIONS
Our objective in this study was to develop a fully
automatic hybrid image segmentation strategy for
meningioma segmentation in Magnetic Resonance
brain images. The supervised segmentation
framework is built on the top of the Graph Cut
algorithm initialized automatically. The strategy was
tested on a preliminary collected data set. The
results prove that the allied use of Support Vector
Machine and Graph Cut produces accurate
segmentation of tumors present in scenarios of
varied complexity. Accuracy results obtained are
encouraging. Future plans contemplate the
acquisition of new data with which to perform a
more significant interpatient analysis and to develop
of a comparative evaluation with other methods.
REFERENCES
Balafar, M., Ramli, A., Saripan, M., Mashohor, S., 2010.
‘Review of brain MRI image segmentation
methods.’Artificial Intelligence Review, 33, 261–274.
Bauer, S., Nolte, L-P., Reyes, M., 2011. Fully automatic
segmentation of brain tumor images using support
vector machine classification in combination with
hierarchical conditional random field regularization.
In: MICCAI Int. Conf. on Medical Image Computing
and Computer Assisted Interventions Berlin:
Springer).
Bauer, S., Wiest, R., Nolte, L-P., Reyes, M., 2013. ‘A
survey of MRI-based medical image analysis for brain
tumor studies.’ Phys. Med. Biol., 58, R97–R129.
Boykov, Y., Kolmogorov, V., 2004. ‘ An experimental
comparison of min-cut/max-flow algorithms for
energy minimization in vision.’ PAMI , 26, 1124- 37.
Boykov, Y., Funka-Lea, G., 2006. ‘ Graph cuts and
efficient n-d image segmentation.’ Int. J. Comput.
Vision, 70, 109-131.
Bouix, S., Martin-Fernandez, M., Ungar, L., Koo,
M.N.M.S., McCarley, R.W., Shenton, M.E., 2007.
‘On evaluating brain tissue classifiers without a
ground truth.’ NeuroImage, 36, 1207–24.
Caroli, M., Locatelli M., Prada F., Beretta F., Martinelli-
Boneschi F., Campanella R., Arienta C., 2005.
‘Surgery for intracranial meningiomas in the elderly: a
clinical–radiological grading system as a predictor of ‘
J Neurosurg, 102, 290–294 .
Clarke, L.P., Velthuizen, R., Camacho, M., Heine, J.,
Vaidyanathan, M., Hall, L., Thatcher, R., Silbiger, M.
S., 1995. ‘ MRI segmentation: methods and
applications.’ Magn Reson Imaging, 13(3), 343–36.
Gordillo, N., Montseny, E, Sobrevilla, P., 2013, ‘State of
the art survey on MRI brain tumor segmentation.’
Magn Reson Imaging, 31(8), 1426-38.
NCTA2014-InternationalConferenceonNeuralComputationTheoryandApplications
156