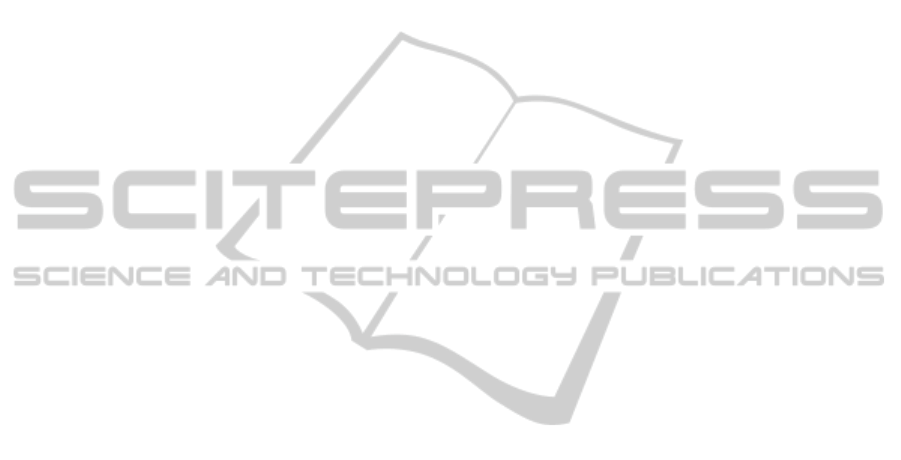
5 CONCLUSIONS
A preliminary step for early, fast, sensitive, and
noninvasive LC diagnosis based on biomarkers in
breath has been looked into and described. It has
been proven that the combination of functionalized
SiNW FET sensors and ANNs are able to more than
adequately classify the eleven different polar and
nonpolar VOCs studied and accurately estimate their
partial pressures in artificial gaseous samples. The
neural network models of the databases generated by
the FET sensors provided a perfect classification of
the analyzed VOCs (ANN1) and the possibility to
determine their partial pressures with MPEs never
greater than 3.3% (ANN2), which consequently
validates both the classifier (ANN1) and the
estimator (ANN2) models.
These promising results open a door to further
research with artificial breath and, in the end, real
breath samples. The final goal of this project is to
precisely define the biomarker profiles in breath of
healthy controls and LC patients and, ideally, the
profiles of every LC stage to be able to detect
potential LC patients and diagnose this disease at the
earliest stage possible. This assisted diagnosis could
help the medical staff make decisions and
conceivably allow identifying early and, most
importantly, curable LC cases.
ACKNOWLEDGEMENTS
The research leading to these results has achieved
funding from the European Union Seventh
Framework Programme (FP7/2007–2013) under
grant agreement no. HEALTH-F4-2011-258868.
REFERENCES
Anand, P., Kunnumakara, A. B., Sundaram, C.,
Harikumar, K.B., Tharakan, S. T., Lai, O. S., Sung, B.,
Aggarwa, B. B. (2008) ‘Cancer is a Preventable
Disease that Requires Major Lifestyle Changes’,
Pharm. Res., vol. 25, no. 92, pp.097-2116.
Blase, X., Serra-Fernández, M. V. (2008) ‘Preserved
Conductance in Covalently Functionalized Silicon
Nanowires’, Physical Review Letters, vol. 100, no. 4.
Cancilla, J. C., Torrecilla, J. S., Matute, G. (2014)
‘Current Applications of Artificial Neural Networks in
Biochemistry with Emphasis on Cancer Research’,
Curr. Biochem. Eng., vol. 1.
Chen, K. I., Li, B. R., Chen, Y. T. (2011) ‘Silicon
Nanowire Field-Effect Transistor-Based Biosensors
for Biomedical Diagnosis and Cellular Recording
Investigation’, Nano Today, vol. 6, pp. 131-154.
Cui, Y., Zhong, Z., Wang, D., Wang, W.U., Lieber, C.M.
(2003) ‘High Performance Silicon Nanowire Field
Effect Transistors’, Nano Letters, vol. 3, no. 2.
Demuth, H., Beale, M., Hagan, M. (2005) ‘Neural
Network Toolbox for Use with MATLAB® User’s
Guide’. Version 4.0.6. Ninth printing Revised for
Version 4.0.6 (Release 14SP3), Natick, MA (USA).
Desai, K. M., Survase, S. A., Saudagar, P. S., Lele, S. S.,
Singhal, P.S. (2008) ‘Comparison of Artificial Neural
Network (ANN) and Response Surface Methodology
(RSM) in Fermentation Media Optimization: Case
Study of Fermentative Production of Scleroglucan’,
Biochem. Eng. J., vol. 41, num. 3, pp. 266-273.
Farrington, S. M., Lin-Goerke, J., Ling, J., Wang, Y.,
Burczak, J. D., Robbins, D. J., Dunlop, M. G. (1998)
‘Systematic Analysis of hMSH2 and hMLH1 in
Young Colon Cancer Patients and Controls’, Am. J.
Hum. Genet., vol. 63, pp. 749-759.
Flores-Fernández, J. M., Herrera-López, E. J., Sánchez-
Llamas, F., Rojas-Calvillo, A., Cabrera-Galeana, P.A.,
Leal-Pacheco, G., González-Palomar, M. G., Femat,
R., Martínez-Velázquez, M. (2012) ‘Development of
an Optimized Multi-biomarker Panel for the Detection
of Lung Cancer Based on Principal Component
Analysis and Artificial Neural Network Modeling’,
Expert Syst. Appl., vol. 39, no. 12, pp. 10851-10856.
Gueguim-Kana, E. B., Oloke, J. K., Lateef, A., Adesiyan,
M.O. (2012) ‘Modeling and optimization of biogas
production on saw dust and other co-substrates using
Artificial Neural network and Genetic Algorithm’,
Renew. Energy., vol. 46, pp. 276-281.
Jain, A. K., Mao, J., Mohiuddin, K. M. (1996) ‘Artificial
Neural Networks: A Tutorial’, Computer, vol. 29, no.
3, pp. 31-44.
Jemal, A., Bray, F., Center, M. M., Ferlay, J., Ward, E.,
Forman, D. (2011) ‘Global Cancer Statistics’, CA
Cancer J. Clin., vol. 61, pp. 69-90.
Knoerzer, K., Juliano, P., Roupas, P., Versteeg, C. (2011)
‘Innovative Food Processing Technologies: Advances
in Multiphysics Simulation’, Oxford (UK), Wiley-
Blackwell.
Li, Y., Qian, F., Xiang, J., Lieber, C. M. (2006) ‘Nanowire
Electronic and Optoelectronic Devices’, Materials
Today, vol. 9, no. 10.
McGrath, M., Lee, I. M., Buring, J., De-Vivo, I. (2011)
‘Common Genetic Variation Within IGFI, IGFII,
IGFBP-1, and IGFBP-3 and Endometrial Cancer Risk’
Gynecol. Oncol., vol. 120 no. 2, pp. 174-178.
Oliferenko, A. A., Oliferenko, P. V., Torrecilla, J. S.,
Katritzkya, A. R. (2013) ‘Rebuttal to “comments on
“Boiling Points of Ternary Azeotropic Mixtures
Modeled with the Use of Universal Solvation Equation
and Neural Networks”’, Industrial & Engineering
Chemistry Research, vol. 52, pp. 545-546.
Palancar, M. C., Aragon, J. M., Torrecilla, J. S. (1998)
‘pH-Control System Based on Artificial Neural
Networks; Industrial & Engineering Chemistry
Research’, vol. 37, no. 7, pp. 2729-2740.
VolatileOrganicCompoundDetectionwithFETSensorsandNeuralNetworkDataProcessingasaPreliminaryStepto
EarlyLungCancerDiagnosis
63