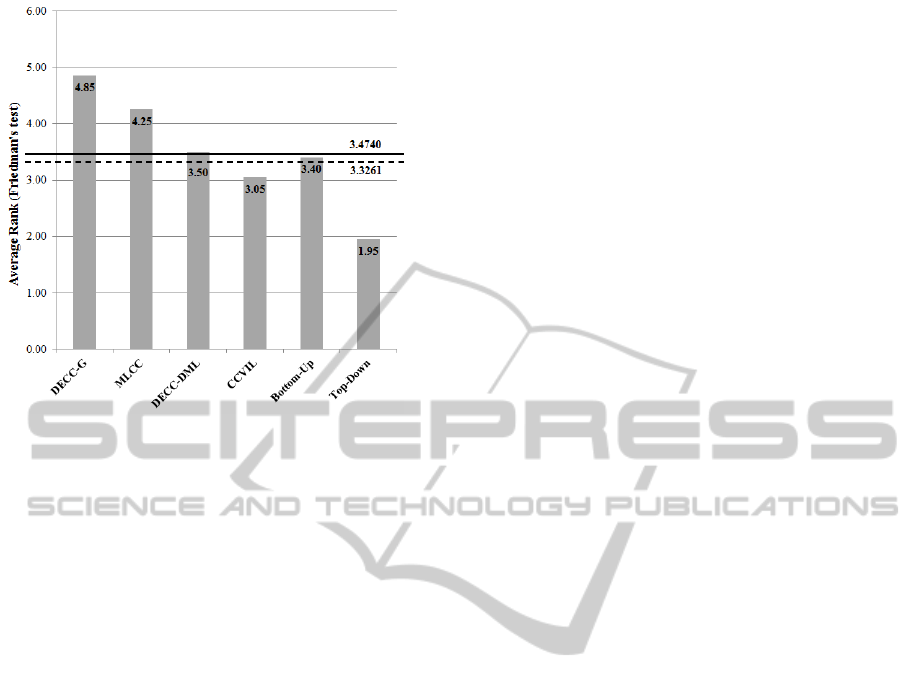
Figure 3: The Graphical Representation of Statistical Anal-
ysis with Friedman’s test and Bonferroni-Dunn’s method.
is proved that the proposed D-HMP-CA is a scalable
method such that it offers competitive performance to
solve large scale global optimization problems com-
pared to the state-of-the-art methods.
Although D-HMP-CA offers a great performance
to solve large scale global optimization problems, it
can be further improved by incorporating more ad-
vanced decomposition strategies. Detecting variable
interactions in dimension decomposition approach is
considered as future direction for this research.
ACKNOWLEDGEMENTS
This work is made possible by the support from
the National Science Foundation under Grant DEB-
0816400 and NSERC Discovery under Grant No.
327482.
REFERENCES
Chen, W., Weise, T., Yang, Z., and Tang, K. (2010). Large-
scale global optimization using cooperative coevo-
lution with variable interaction learning. In Paral-
lel Problem Solving from Nature (PPSN XI), volume
6239, pages 300–309.
Garca, S., Molina, D., Lozano, M., and Herrera, F. (2009).
A study on the use of non-parametric tests for ana-
lyzing the evolutionary algorithms’ behaviour: A case
study on the cec’2005 special session on real parame-
ter optimization. Journal of Heuristics, 15:617–644.
Li, X. and Yao, X. (2012). Cooperatively coevolving parti-
cle swarms for large scale optimization. IEEE Trans-
actions on Evolutionary Computation, 16 (2):210–
224.
Lin, C.-J., Weng, C.-C., Lee, C.-L., and Lee, C.-Y.
(2009). Using an efficient hybrid of cooperative par-
ticle swarm optimization and cultural algorithm for
neural fuzzy network design. In International Con-
ference on Machine Learning and Cybernetics, pages
3076–3082.
Liu, Y., Yao, X., Zhao, Q., and Higuchi, T. (2001). Scal-
ing up fast evolutionary programming with coopera-
tive coevolution. In IEEE Congress on Evolutionary
Computation (CEC), pages 1101–1108.
Olorunda, O. and Engelbrecht, A. (2009). An analysis
of heterogeneous cooperative algorithms. In IEEE
Congress on Evolutionary Computation (CEC), pages
1562–1569.
Omidvar, M. N., Li, X., and Yao, X. (2010). Cooperative
co-evolution with delta grouping for large scale non-
separable function optimization. In IEEE Congress on
Computational Intelligence (CEC), pages 1–8.
Potter, M. and De Jong, K. (1994). A cooperative coevolu-
tionary approach to function optimization. In The 3rd
Conference on Parallel Problem Solving from Nature
(PPSN), volume 2, pages 249–257.
Raeesi N., M. R., Chittle, J., and Kobti, Z. (2014). A new
dimension division scheme for heterogenous multi-
population cultural algorithm. In The 27th Florida
Artificial Intelligence Research Society Conference
(FLAIRS-27), Pensacola Beach, FL, USA.
Raeesi N., M. R. and Kobti, Z. (2013). Heteroge-
neous multi-population cultural algorithm. In IEEE
Congress on Evolutionary Computation (CEC), pages
292–299, Cancun, Mexico.
Raeesi N., M. R. and Kobti, Z. (2014). Heterogeneous
multi-population cultural algorithm with a dynamic
dimension decomposition strategy. In The 27th Cana-
dian Conference on Artificial Intelligence (Canadian
AI), Montreal, QC, Canada. (To Be Appeared).
Reynolds, R. G. (1994). An introduction to cultural algo-
rithms. In Sebald, A. V. and Fogel, L. J., editors,
Thirs Annual Conference on Evolutionary Program-
ming, pages 131–139, River Edge, New Jersey. World
Scientific.
Tang, K., Li, X., P.N., S., Yang, Z., and Weise, T. (2009).
Benchmark functions for the CEC’2010 special ses-
sion and competition on large scale global optimiza-
tion. Technical report, NICAL, USTC, Hefei, Anhui,
China.
van den Bergh, F. and Engelbrecht, A. (2000). Coopera-
tive learning in neural networks using particle swarm
optimizers. South African Computer Journal, pages
84–90.
Yang, Z., Tang, K., and Yao, X. (2008a). Large scale evo-
lutionary optimization using cooperative coevolution.
Information Sciences, 178 (15):2985–2999.
Yang, Z., Tang, K., and Yao, X. (2008b). Multilevel co-
operative coevolution for large scale optimization. In
IEEE Congress on Evolutionary Computation (CEC),
pages 1663–1670.
DynamicHeterogeneousMulti-PopulationCulturalAlgorithmforLargeScaleGlobalOptimization
191