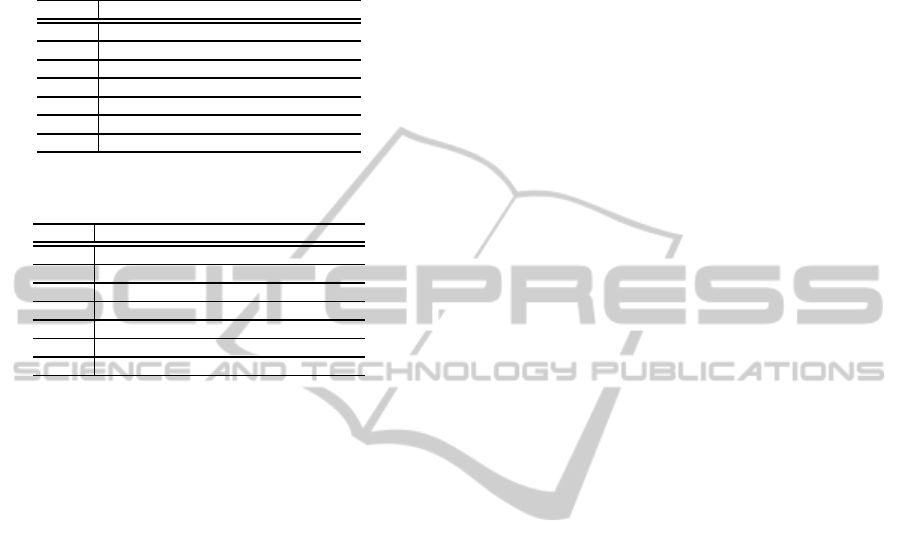
Table 5: The objective function values of preferred solu-
tions each selected by PSSA among the solutions obtained
from MQEA-PS and DMQEA, respectively.
(a) Average objective values of a preferred so-
lution finally selected by PSSA among the solu-
tions obtained from MQEA-PS
f
1
f
2
f
3
f
4
f
5
DTLZ1 0.0599 0.0002 0.1402 0.0002 0.4596
DTLZ2 0.0000 0.0000 0.0000 0.0000 1.0000
DTLZ3 0.0021 0.0002 0.0027 0.1232 5.5938
DTLZ4 1.0000 0.0000 0.0000 0.0000 1.0000
DTLZ5 0.0000 0.0000 0.0000 0.0000 1.0000
DTLZ6 0.0002 0.0002 0.0002 0.0003 1.8851
DTLZ7 0.6302 0.0022 0.6022 0.0005 9.2351
(b) Average objective values of a preferred so-
lution finally selected by PSSA among the solu-
tions obtained from DMQEA
f
1
f
2
f
3
f
4
f
5
DTLZ1 0.0685 0.0005 0.1401 0.0005 0.5994
DTLZ2 0.0000 0.0000 0.0000 0.0000 1.0003
DTLZ3 0.0000 0.0000 0.0000 0.0000 4.4475
DTLZ4 1.0251 0.0000 0.0000 0.0000 1.0000
DTLZ5 0.0000 0.0000 0.0000 0.0000 1.0001
DTLZ6 0.0004 0.0002 0.0004 0.0006 4.1782
DTLZ7 0.1235 0.0017 0.3517 0.0026 10.7801
measures representing user’s preference for objec-
tives. By employing the secondary objectives-based
nondominated sorting in each archive generation pro-
cess, DMQEA could generate the preferable and di-
verse solutions. For the performance comparisons
among MQEA, MQEA-PS, DMQEA, and NSGA-
II, seven DTLZ functions were used as benchmark
functions, and hypervolume and diversity were em-
ployed as performance metrics. The experimental re-
sults confirmed that the proposed DMQEA was able
to generate more optimized solutions for the preferred
objectives compared with the other algorithms.
ACKNOWLEDGEMENTS
This work was supported by the Technology Innova-
tion Program, 10045252, Development of robot task
intelligence technology, funded by the Ministry of
Trade, Industry & Energy (MOTIE, Korea).
REFERENCES
Bajwa, G., Choo, E., and Wedley, W. (2008). Effectiveness
analysis of deriving priority vectors from reciprocal
pairwise comparison matrices. Asia-Pacific Journal
of Operational Research, 25(3):279–299.
Bosman, P. A. and Thierens, D. (2003). The bal-
ance between proximity and diversity in multiobjec-
tive evolutionary algorithms. evolutionary computa-
tion. IEEE Transactions on Evolutionary Computa-
tion, 7(2):174–188.
Cui, X., Li, M., and Fang, T. (2001). Study of population di-
versity of multiobjective evolutionary algorithm based
on immune and entropy principles. In Proc. of IEEE
Congress on Evolutionary Computation, pages 1316–
1321.
Deb, K., Pratap, A., Agarwal, S., and Meyarivan, T. (2002).
A fast and elitist multiobjective genetic algorithm:
Nsga-ii. IEEE Transactions on Evolutionary Compu-
tation, 6(2):182–197.
Han, K.-H. and Kim, J.-H. (2002). Quantum-inspired evo-
lutionary algorithm for a class of combinatorial opti-
mization. IEEE Transactions on Evolutionary Com-
putation, 6(6):580–593.
Han, K.-H. and Kim, J.-H. (2004). Quantum-inspired evo-
lutionary algorithms with a new termination criterion,
hε gate, and two phase scheme. IEEE Transactions on
Evolutionary Computation, 8(2):156–169.
Hey, T. (1999). Quantum computing: an introduction. Com-
puting and Control Engineering Journal, 10(3):105–
112.
Kim, J.-H., Han, J.-H., Kim, Y.-H., Choi, S.-H., and Kim,
E.-S. (2012). Preference-based solution selection
algorithm for evolutionary multiobjective optimiza-
tion. IEEE Transactions on Evolutionary Computa-
tion, 16(1):20–34.
Kim, J.-H., Kim, Y.-H., Choi, S.-H., and Park, I.-W.
(2009). Evolutionary multi-objective optimization in
robot soccer system for education. IEEE Computa-
tional Intelligence Magazine, 4(1):31–41.
Kim, Y.-H., Kim, J.-H., and Han, K.-H. (2006). Quantum-
inspired multiobjective evolutionary algorithm for
multiobjective 0/1 knapsack problems. In Proc. of
IEEE Congress on Evolutionary Computation, pages
2601–2606.
Laumanns, M., Thiele, L., Deb, K., and Zitzler, E. (2002).
Combining convergence and diversity in evolutionary
multiobjective optimization. IEEE Transactions on
Evolutionary Computation, 10(3):263–282.
Lee, K.-B. and Kim, J.-H. (2012). Multiobjective particle
swarm optimization with preference-based sort and its
application to path following footstep optimization for
humanoid robots. IEEE Transactions on Evolutionary
Computation, 17(6):755–766.
Li, H., Zhang, Q., Tsang, E., and Ford, J. (2004). Hy-
brid estimation of distribution algorithm for multiob-
jective knapsack problem. Evolutionary Computation
in Combinatorial Optimization, pages 145–154.
Ryu, S.-J., Lee, K.-B., and Kim, J.-H. (2012). Improved
version of a multiobjective quantum-inspired evolu-
tionary algorithm with preference-based selection. In
Proc. of IEEE World Congress on Computational In-
telligence, pages 1672–1678.
Zitzler, E. (1999). Evolutionary algorithms for multi-
objective optimization: methods and applications.
Berichte aus der Informatik, Shaker Verlag, Aachen-
Maastricht.
ECTA2014-InternationalConferenceonEvolutionaryComputationTheoryandApplications
214