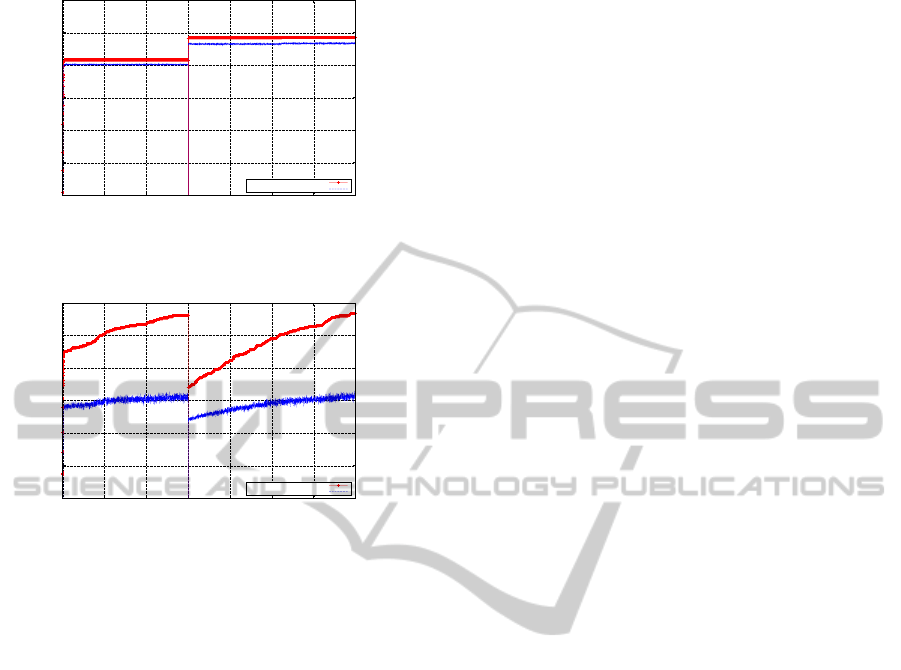
780
800
820
840
860
880
900
0 1000 2000 3000 4000 5000 6000 7000
Fitness
Generations
Thirty 3-Bit Deceptive Changing Landscape - Online/Offline - 6-Bit MGA Representation - No Missense
Off-line Performance
On-line Performance
Figure 28: 6-3-1 MGA Representation - Thirty 3-Bit De-
ceptive Problem - No Missense.
780
800
820
840
860
880
900
0 1000 2000 3000 4000 5000 6000 7000
Fitness
Generations
Thirty-3 Bit Deceptive Changing Landscape - Online/Offline - Increased Mutation - SGA
Off-line Performance
On-line Performance
Figure 29: Thirty 3-Bit Deceptive Problem - SGA - In-
creased Mutation.
6 CONCLUSION
From the above experiments the results indicate that
overall the MGA appears robust over both static and
changing landscapes. By incorporating a tunable GP-
map, the MGA offers the ability to vary the gran-
ularity of the representation, which appears benefi-
cial over various landscapes and the performance of
the MGA varies slightly depending on the level of
granularity in the representation. Over the static One
Max landscapes, the MGAs off-line and on-line per-
formances were quite similar to those of the SGA
over both the easier 30-bit problem and the more dif-
ficult 90-bit problem. On reason for this is that the
problem, by its nature, is relatively easy for a GA to
solve. What is of interest is that the MGA, in terms
of robustness, performed as well as the SGA. How-
ever, over the deceptive changing landscape experi-
ments the MGA outperformed the SGA, both on-line
and off-line, particularly over the more difficult ten 3-
bit deceptive problem. In relation to the use of Tran-
scription, it appear from the results that the advan-
tage of this phase is not apparent over less difficult
landscapes, but once the level of difficulty increases,
as with the more difficult deceptive problem, Tran-
scription proved useful. one possible reason for this
is that the Transcription phase performs a form varia-
tion, quite similar to inversion.
The inclusion of a missense mutation operator,
which operated at the RNA level, results indicated
that it assisted in the search strategy, over both diffi-
cult and less difficult landscapes. To examine whether
this was a result of additional mutation, we carried
out experiments which increased the mutation rate
for the SGA and results indicated that over the more
challenging deceptive landscape additional mutations
failed to assist the search strategy. This we feel, indi-
cates that the use of missense mutation, which oper-
ates at levels within the GP-map may be beneficial.
To conclude, the inclusion of a biologically in-
spired tunable GP-map which allows the granular-
ity of the GP-map to be altered appears to assist in
searching various landscapes and further research into
the levels of diversity maintained and population dy-
namics by the various MGA representations is re-
quired to gain a greater understanding.
REFERENCES
Goldberg, D. E., Korb, B., and Deb, K. (1990). Messy
genetic algorithms: Motivation, analysis, and first re-
sults. Complex Systems, 3(5):493–530.
Goldberg, D. E. and Smith, R. E. (1987). Nonstationary
function optimization using genetic algorithm with
dominance and diploidy. In Proceedings of the 2nd
International Conf. on Genetic Algorithms on Ge-
netic Algorithms and Their Application, pages 59–68,
Hillsdale, NJ, USA. L. Erlbaum Associates Inc.
Grefenstette, J. J. and Cobb, H. G. (1993). Genetic algo-
rithms for tracking changing environments. In Proc.
of the 5th Int. Conf. on Genetic Algorithms and their
Applications, pages 523–530. Morgan Kaufmann.
Hill, S. and O’Riordan, C. (2011). Examining the use of a
non-trivial fixed genotype-phenotype mapping in ge-
netic algorithms to induce phenotypic variability over
deceptive uncertain landscapes. In Proceedings of the
2011 Congress of Evolutionary Computation (CEC
2011). New Orleans, USA.
Kubalik, J. (2005). Using genetic algorithms with real-
coded binary representation for solving non-stationary
problems. In Ribeiro, B., Albrecht, R. F., Dobnikar,
A., Pearson, D. W., and Steele, N., editors, Adaptive
and Natural Computing Algorithms, pages 222–225.
Springer Vienna.
Morrison, R. W. and DeJong, K. A. (2002). Measurement
of population diversity. In In 5th International Con-
ference EA, 2001, volume 2310 of Incs. Springer.
Whitley, L. D. (1991). Fundamental principles of deception
in genetic search. In Rawlins, G. J., editor, Founda-
tions of genetic algorithms, pages 221–241. Morgan
Kaufmann, San Mateo, CA.
Yang, S. (2006). On the design of diploid genetic algorithms
for problem optimization in dynamic environments.
In Evolutionary Computation, 2006. CEC 2006. IEEE
Congress on, pages 1362–1369.
ECTA2014-InternationalConferenceonEvolutionaryComputationTheoryandApplications
222