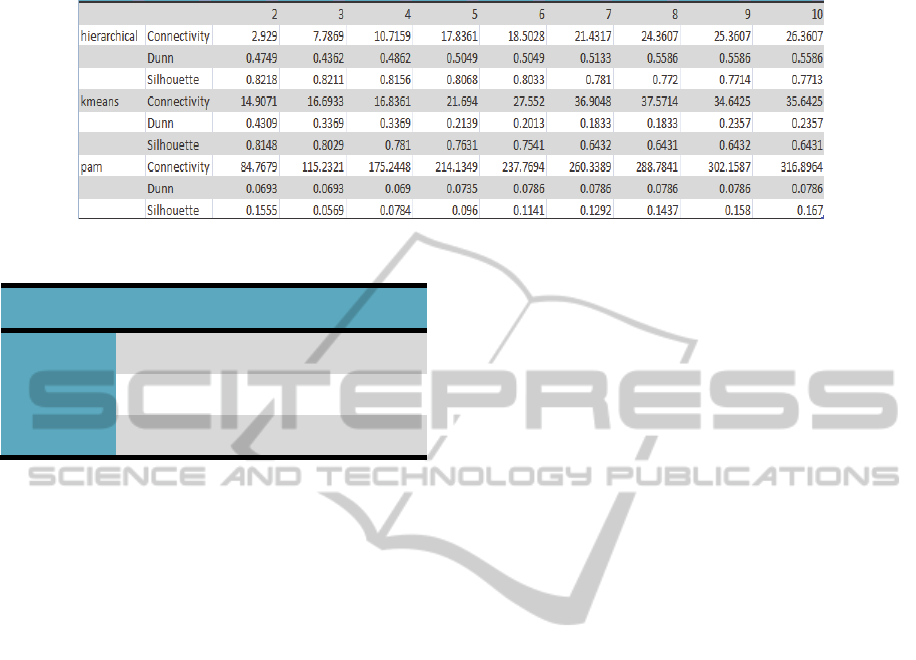
Table 6: Comparison of results using: “Ward”, “K-means” and “PAM”.
Table 7: Optimum results for grouping.
Score Method Clusters
Connectivity
2.9290 hierarchical 2
Dunn
0.5586 hierarchical 8
Silhouette
0.8218 hierarchical 2
most used in violent and non violent conversations.
The word clouds and term graphs were very useful
to visualize the most used words and their frequency
in each type of conversationIt was observed that in
both, violent and non violent conversations, there are
terms that are used with similar frequencies, but in
the violent conversations these words are
accompanied by other words considered “bad
words” or “rudeness”.
Once the word grouping was performed, eight
groups of words that appear frequently together in
conversations (violent or non violent) were obtained.
Not all the grouping techniques used were adequate,
but the hierarchic technique ward was the most
efficient given that the closeness of their words was
much better than k-means and PAM.
When classifying terms in violent or non violent
using SVM it was observed that some terms cannot
be classified due to the fact that they appear with
similar frequency in both types of conversations. In
the training sets of SVM it was observed that the
performance depends on the size of the test set.
Using the procedure presented in this work it is
convenient to experiment with a larger number of
video segments, and also use a better pre-processing
that can include synonyms and removal of gender in
most words. Also it is convenient to try more data
mining techniques in order to make a thorough
comparison and obtain better results due to the
larger number of terms.
With the procedure presented in this work it is
possible to design a system capable of classifying
automatically conversations as violent and non
violent. And this system can evolve to make this
classification in real-time in order to trigger some
alarm when a conversation turns violent in order to
alert security personnel to take measures.
Another idea that can be explored is to pre-
classify the video segments in categories like sports,
political, family, commercial, etc. and also by region
or social context in order to help the classification
process.
REFERENCES
clValid: An R Package for Cluster Validation, [Online],
Available at: http://www.jstatsoft.org/v25/i04/paper
[Retrieved January 2014]
Meyer, D., “Support Vector Machines: The Interface to
libsvm in package e1071”, September 2012, [Online],
Available at: http://cran.r-project.org/web/packages/
e1071/vignettes/svmdoc.pdf [Retrieved January 2014]
Brun, R. E., Senso, J. A., Minería textual, [Online],
Available at:
http://www.elprofesionaldelainformacion.com/conteni
dos/2004/enero/2.pdf [Retrieved January 2014]
Montes-y-Gómez, M., Minería de texto: Un nuevo reto
computacional, [Online], Available at: http://ccc.
inaoep.mx/~mmontesg/publicaciones/2001/MineriaTe
xto-md01.pdf [Retrieved January 2014]
Villanueva, V. J., Escribano, M., Isorna, M., Pellicer, J.,
Alapont, L., Pellicer, P., Programa de apoyo al ámbito
familiar: Agresividad y violencia, Editorial IES Pablo
Serrano. Andorra (Teruel), España, 2007.
Adobe Premiere Pro CS6, [Online], Available at:
http://www.adobe.com/mena_en/products/premiere.ht
ml [Retrieved January 2014]
Modelos de análisis de voz para Adobe Premiere Pro CS6,
[Online], Available at: http://www.adobe.com/es/
products/premiere/extend.displayTab3.html,
[Retrieved January 2014]
RStudio v0.97.551, [Online], Available at: http://www.
rstudio.com/ide/download/desktop [Retrieved January
2014]
ViolenceRecognitioninSpanishWordsusingDataMining
215