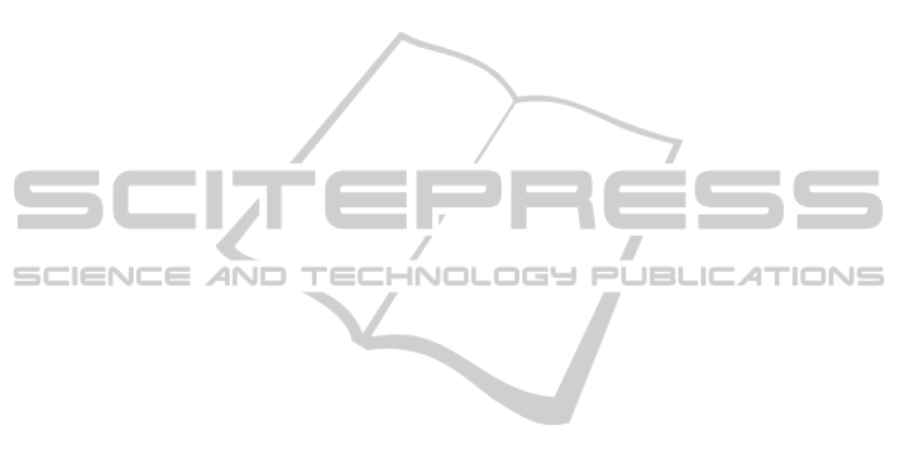
from the point of view of the evaluator. There exists
another form of bias, the kind that is inherent in the
design of the ontologies. An ontology (a model of do-
main knowledge) represents the domain in the context
of the time, place, and cultural environment in which
it was created as well as the modellers perception of
the domain (Brank et al., 2005; Brewster et al., 2004).
The problem lies in the unexplored potential in-
fluence of this subjectivity in the evaluation results. If
we take a data-driven approach to ontology evaluation
for example, it would be interesting to see how the
evaluation results spread over each dimension of the
domain knowledge (i.e. temporal, categorical, etc.).
This is based on equating subjectivity/bias to the dif-
ferent dimensions of domain knowledge. To give a
concrete example, let us take the results of Brewster
et al. (Brewster et al., 2004). These are expressed as
a vector representation of the similarity score of each
ontology showing how closely each ontology repre-
sents the domain corpus. This offers a somewhat one
dimensional summarization of this score (coverage)
where one ontology will be picked ahead of the others
based on a high score. It, however, leaves unexplored
how this score changes over the years (temporal), for
example. This could reveal very important informa-
tion such as the relevance of the ontology, meaning
that the ontology might be aging and needs to be up-
dated as opposed to a rival ontology. The results of
Ouyang et al. (Ouyang et al., 2011) are a perfect ex-
emple of this need. They reveal that the results of
their coverage showed a correlation between the cor-
pus used and the resultant coverage. This revelation is
consistent with the notion of dynamic domain knowl-
edge. In fact, a changing domain knowledge has been
attributed to the reasons for changes to the ontologies
themselves (Nonaka and Toyama, 2005). This offers
an avenue to explore and account for bias as well as
its influence on the evaluation results. This forms the
main research interest of this paper.
Thus far, to the best of our knowledge, no research
in ontology evaluation has been undertaken to account
for subjectivity. This has not been especially done to
measure subjectivity in the context of a scale as op-
posed to binary (yes- it is subjective, or no - it is not
subjective). Hence, this provides a means to account
for the influences of bias (subjectivity) on the individ-
ual metrics of evaluation that are being measured.
4 THEORETICAL FRAMEWORK
The framework presented in this paper which is rem-
iniscent of Vrandecic’s framework for ontology eval-
uation (Vrandecic, 2010) is depicted and summarized
in Figure 1. Sections 5 through 8 explain the fun-
damental components of this framework and provide
details on how they relate to each other. An ontol-
ogy (O) has been defined as a formal specification of
a domain of interest through the definition of the con-
cepts in the domain and the relationships that hold
between them. An ontology set (S) is a collection
of ontologies, ∃O ∈ S. Evaluation methods evaluate
an ontology or a set of ontologies. For the purposes
of a data-driven approach to ontology evaluation, the
evaluation is conducted from the viewpoint of a do-
main corpus. Put simply, evaluation methods evaluate
ontologies against the domain corpus by using met-
rics and their measures to measure the correctness or
quality of the ontologies. In other terms, an ontology
evaluation which is the result of the application of an
evaluation methodology, is expressed by metrics. In a
data-driven ontology evaluation undertaking, the do-
main corpus is a proxy for the domain of interest. We
argue that this proxy is non-static and changes over
several dimensions including the temporal, categori-
cal, etc. These dimensions are argued to be the bias
factors and this work endeavours to explore their in-
fluence on ontology evaluation.
5 THE CORPUS
Current research in data-driven ontology evaluation
assume that domain knowledge is constant. Hence,
the premise of this paper:
Premise
Literature has suggested that an ontology (a model of
domain knowledge) represents the domain in the con-
text of the time, place, and cultural environment in
which it was created as well as the modeller’s per-
ception of the domain (Brank et al., 2005; Brewster
et al., 2004). We argue that this extends to domain
knowledge. Domain knowledge or concepts are dy-
namic and change over multiple dimensions includ-
ing the temporal, spatial and categorical dimensions.
There has been recent attempts to formalize this in-
herent diversity, for example, in the form of a knowl-
edge diversity ontology (Thalhammer et al., 2011).
We therefore, argue that any evaluation based on a
corpus should then do it over these dimensions. This
is something that has been overlooked by current re-
search on data-driven ontology evaluation.
5.1 Temporal
As previously mentioned, information about a domain
can be discussed on its temporal axis. This is espe-
KEOD2014-InternationalConferenceonKnowledgeEngineeringandOntologyDevelopment
58