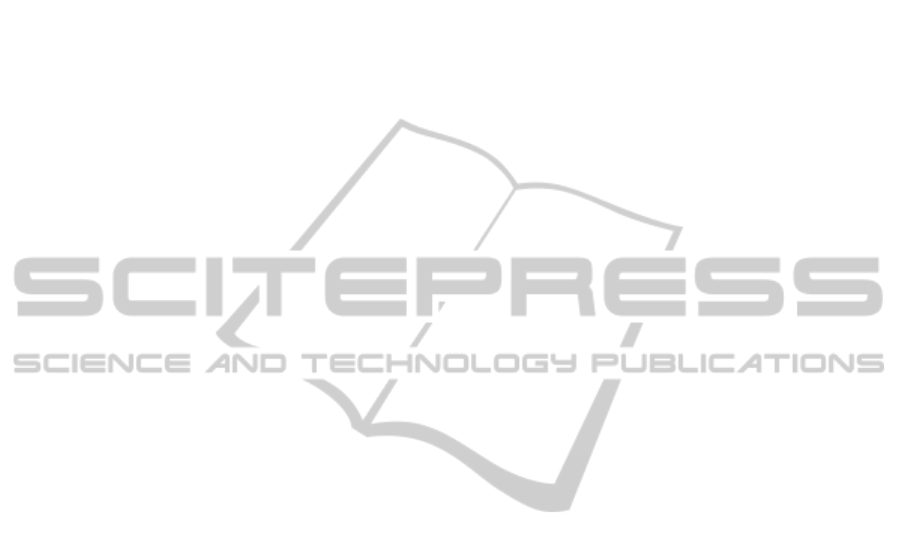
5 CONCLUSION
Although during the last years, research on and with
swarm intelligence has reached an impressive state,
there are still many open problems, and new
application areas are continually emerging for the
optimization paradigms.
We undertook a comparative study of EFPA with
classical FPA over a test-suite comprising 5 well-
known numerical benchmarks and the Loney’s
solenoid problem. Our simulation results indicate
that the EFPA remains always better than FPA. In
near future, we are planning to compare the EFPA
with good performing algorithms available in
literature, such differential evolution and covariance
matrix adaptation evolution strategy.
REFERENCES
Abdel-Raouf, O., El-Henawy, I., Abdel-Baset, M. (2014).
A novel hybrid flower pollination algorithm with
chaotic harmony search for solving Sudoku puzzles,
International Journal of Modern Education and
Computer Science, 3: 38-44.
Ali, M. M. (2007). Synthesis of the β-distribution as an aid
to stochastic global optimization. Computational
Statistics & Data Analysis, 52(1): 133-149.
Ciuprina, G., Ioan, D., and Munteanu, I. (2002). Use of
intelligent-particle swarm optimization in
electromagnetics. IEEE Transactions on Magnetics
38(2): 1037-1040.
Coelho, L. S. and Alotto, P. (2007). Loney’s solenoid
design using artificial immune network with local
search based on Nelder-Mead simplex method,
COMPUMAG, Aachen, Germany.
Coelho, L. S., Guerra, F. A., Batistela, N. J., and Leite, J.
V. (2013). Multiobjective cuckoo search algorithm
based on Duffing’s oscillator applied to Jiles-Atherton
vector hysteresis parameters estimation. IEEE
Transactions on Magnetics 49: 1745-1748.
Dorigo, M. and Stützle, T. (2004). Ant colony
optimization, MIT Press.
Di Barba, P. and Savini, A. (1995). Global optimization of
Loney’s solenoid by means of a deterministic
approach. International Journal of Applied
Electromagnetics and Mechanics 6(4): 247-254.
Eberhart, R. C., Shi, Y., and Kennedy, J. (2001). Swarm
intelligence. The Morgan Kaufmann Series In
Evolutionary Computation.
Engelbrecht, A. P. (2007). Computational intelligence: an
introduction, 2nd edition, John Wiley & Sons Ltd.,
New York, USA.
Fernández, V. A., Galetto, L., and Astegiano, J. (2009).
Influence of flower functionality and pollination
system on the pollen size-pistil length relationship.
Organisms Diversity & Evolution 9(2): 75-82.
Gandomi, A. H. and Yang, X. -S. (2014). Chaotic bat
algorithm. Journal of Computational Science 5(2):
224-232.
Gandomi, A. H., Alavi, A. H. (2012). Krill herd: a new
bio-inspired optimization algorithm. Communications
in Nonlinear Science and Numerical Simulation
17(12): 4831-4845.
Karaboga, D. (2005). An idea based on honey bee swarm
for numerical optimization. Technical Report-TR06,
Erciyes University, Engineering Faculty, Computer
Engineering Department, Turkey.
Kasinger, H., Vauer, B. (2006). Beyond swarm
intelligence: building self-managing systems based on
pollination. GI Jahrestagung 1 93, LNI, 169-176.
Kaur, G., Singh, D. (2012). Pollination based optimization
for color image segmentation. International Journal of
Computer Engineering & Technology 3(2): 407-414.
Lévy, P. (1925). Calcul des probabilites. Gauthier Villars,
Paris, France.
Nolan, J. P. (2010). Stable distributions: models for heavy
tailed data, Birkhauser, Boston, USA.
Weng, L., Liu, Q., Xia, M., Song, Y. D. (2014). Immune
network-based swarm intelligence and its application
to unmanned aerial vehicle (UAV) swarm
coordination. Neurocomputing 125: 134-141.
Yang, X. -S. (2010). A new metaheuristic bat-Inspired
algorithm. In: Gonzalez J. R. et al. (editors). Nature
Inspired Cooperative Strategies for Optimization
(NISCO 2010). Berlin: Springer, 65-74.
Yang, X. -S. (2009). Firefly algorithms for multimodal
optimization. Stochastic Algorithms: Foundations and
Applications, SAGA. Lecture Notes in Computer
Sciences vol. 5792, 169-178.
Yang, X. -S. (2012). Flower pollination algorithm for
global optimization. Unconventional Computation and
Natural Computation, Lecture Notes in Computer
Science, vol. 7445, 240-249.
EnhancedFlowerPollinationApproachAppliedtoElectromagneticOptimization
227