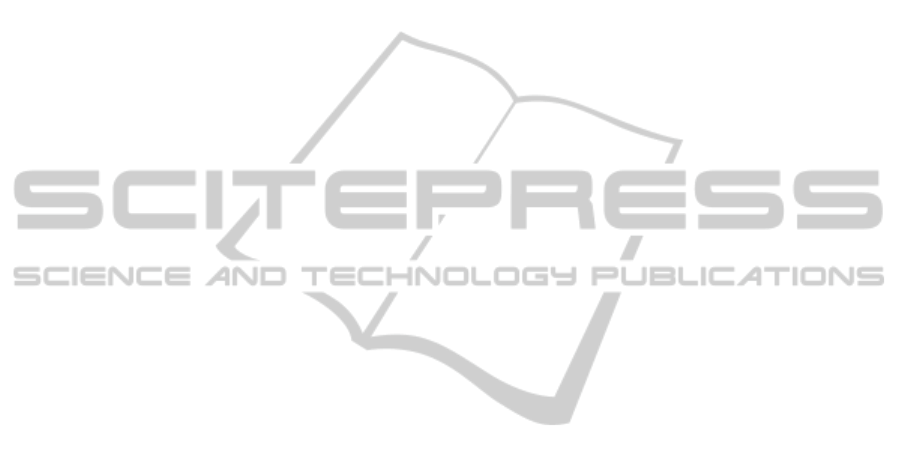
Graf, A. B. A. and Borer, S. (2001). Normalization in sup-
port vector machines. In in Proc. DAGM 2001 Pattern
Recognition, pages 277–282. SpringerVerlag.
Grauman, K. and Darrell, T. (2005). The pyramid match
kernel: discriminative classification with sets of image
features. In Computer Vision, 2005. ICCV 2005. Tenth
IEEE International Conference on, volume 2, pages
1458–1465 Vol. 2.
Ikizler-Cinbis, N. and Sclaroff, S. (2010). Object, scene
and actions: Combining multiple features for human
action recognition. In Computer Vision–ECCV 2010,
pages 494–507. Springer.
Iosifidis, A., Tefas, A., and Pitas, I. (2013a). Dy-
namic action recognition based on dynemes and ex-
treme learning machine. Pattern Recognition Letters,
34(15):1890–1898.
Iosifidis, A., Tefas, A., and Pitas, I. (2013b). Multi-
dimensional sequence classification based on fuzzy
distances and discriminant analysis. Knowledge
and Data Engineering, IEEE Transactions on,
25(11):2564–2575.
Kapsouras, I., Karanikolos, S., Nikolaidis, N., and Tefas,
A. (2013). Feature comparison and feature fusion for
traditional dances recognition. In Engineering Appli-
cations of Neural Networks, pages 172–181. Springer.
Kl¨aser, A., Marszalek, M., and Schmid, C. (2008). A spatio-
temporal descriptor based on 3d-gradients. In BMVC.
British Machine Vision Association.
Lazebnik, S., Schmid, C., and Ponce, J. (2006). Beyond
bags of features: Spatial pyramid matching for rec-
ognizing natural scene categories. In Proceedings
of the 2006 IEEE Computer Society Conference on
Computer Vision and Pattern Recognition - Volume 2,
CVPR ’06, pages 2169–2178, Washington, DC, USA.
IEEE Computer Society.
Li, B., Ayazoglu, M., Mao, T., Camps, O. I., and Sznaier, M.
(2011). Activity recognition using dynamic subspace
angles. In Computer Vision and Pattern Recogni-
tion (CVPR), 2011 IEEE Conference on, pages 3193–
3200. IEEE.
Niu, W., Long, J., Han, D., and Wang, Y.-F. (2004). Human
activity detection and recognition for video surveil-
lance. In Multimedia and Expo, 2004. ICME’04. 2004
IEEE International Conference on, volume 1, pages
719–722. IEEE.
Peng, X., Wu, X., Peng, Q., Qi, X., Qiao, Y., and Liu, Y.
(2013). Exploring dense trajectory feature and en-
coding methods for human interaction recognition. In
Proceedings of the Fifth International Conference on
Internet Multimedia Computing and Service, pages
23–27. ACM.
Raptis, M., Kokkinos, I., and Soatto, S. (2012). Dis-
covering discriminative action parts from mid-level
video representations. In Computer Vision and Pat-
tern Recognition (CVPR), 2012 IEEE Conference on,
pages 1242–1249. IEEE.
Ravichandran, A., Chaudhry, R., and Vidal, R. (2013). Cat-
egorizing dynamic textures using a bag of dynamical
systems. IEEE Transactions on Pattern Analysis and
Machine Intelligence, 35(2):342–353.
Robertson, N. and Reid, I. (2006). A general method for
human activity recognition in video. Computer Vision
and Image Understanding, 104(2):232–248.
Rousseau, F. and Vazirgiannis, M. (2013). Graph-of-word
and tw-idf: New approach to ad hoc ir. In Proceedings
of the 22nd ACM International Conference on Infor-
mation & Knowledge Management, CIKM ’13, pages
59–68, New York, USA. ACM.
Sch¨olkopf, B. and Smola, A. J. (2001). Learning with Ker-
nels: Support Vector Machines, Regularization, Opti-
mization, and Beyond. MIT Press, Cambridge, MA,
USA.
Szummer, M. and Jaakkola, T. (2002). Partially labeled
classification with markov random walks. In Ad-
vances in Neural Information Processing Systems,
pages 945–952. MIT Press.
Turaga, P., Chellappa, R., Subrahmanian, V. S., and Udrea,
O. (2008). Machine recognition of human activities:
A survey. Circuits and Systems for Video Technology,
IEEE Transactions on, 18(11):1473–1488.
Wang, H., Kl¨aser, A., Schmid, C., and Liu, C.-L. (2011).
Action Recognition by Dense Trajectories. In IEEE
Conference on Computer Vision & Pattern Recog-
nition, pages 3169–3176, Colorado Springs, United
States.
Wang, H. and Schmid, C. (2013). Action recognition with
improved trajectories. In IEEE International Confer-
ence on Computer Vision, Sydney, Australia.
Willamowski, J., Arregui, D., Csurka, G., Dance, C. R., and
Fan, L. (2004). Categorizing nine visual classes using
local appearance descriptors. In ICPR Workshop on
Learning for Adaptable Visual Systems.
Wu, S., Oreifej, O., and Shah, M. (2011). Action recogni-
tion in videos acquired by a moving camera using mo-
tion decomposition of lagrangian particle trajectories.
In Computer Vision (ICCV), 2011 IEEE International
Conference on, pages 1419–1426. IEEE.
Zhang, J., Lazebnik, S., and Schmid, C. (2007). Local fea-
tures and kernels for classification of texture and ob-
ject categories: a comprehensive study. International
Journal of Computer Vision, 73:2007.
NCTA2014-InternationalConferenceonNeuralComputationTheoryandApplications
202