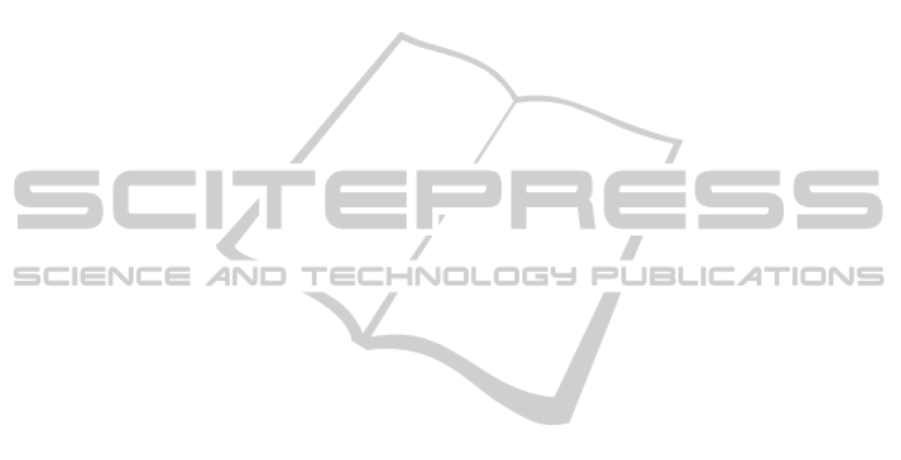
easily extended to any given Western language due
to the actually large availability of resources such
as POS taggers and stemming algorithms. Prelim-
inary evaluation results suggest us that once a sat-
isfactory set of language-specific resources is avail-
able, the overall quality of the generated KPs is not
affected by the language switch. The four different
classes of knowledge considered provide a concep-
tual framework with a higher level of abstraction than
other state-of-the-art systems, featuring a clear sepa-
ration between language dependent and independent
KP selection criteria. Such framework allows us to
overcome several shortcomings of the current sys-
tems which often consider only one or two classes
of knowledge. Moreover, the unsupervised nature
of our approach allows our system to accomplish its
task with no need of training data, which is a major
advantage for non-English languages because of the
tremendous lack of annotated data corpora that we are
experiencing nowadays.
Results gathered so far show a promising outlook
and the system can be effectively employed in sev-
eral application domains, such as digital libraries and
recommender systems.
Our future work will therefore address all the ma-
jor issues highlighted by the expert evaluation, such as
a still high number of KPs perceived as too generic.
We also aim at improving the overall underlined con-
ceptual model of human KP generation, by further
analysing the four knowledge classes identified and
by refining the reasoning process exploited in the sys-
tem. We plan to observe how experts identify KPs,
for instance, by thinking-aloud interviews. The user
interaction should be improved as well, since the sys-
tem actually acts as a black box giving little or no
hints to the final user of the process that selected a
particular KP, and this encourages distrust in the sys-
tem. In order to address this issue, the development
of an interactive explanation and result tracking inter-
face is ongoing. Finally, specific attention will be de-
voted to the evaluation issues, both (i) for improving
and completing the evaluation of our approach and
(ii) for contributing to the development of a method-
ological standard for evaluating KP extraction and KP
inference capabilities systems.
REFERENCES
Barker, K. and Cornacchia, N. (2000). Using noun phrase
heads to extract document keyphrases. In Advances in
Artificial Intelligence, pages 40–52. Springer.
Danilevsky, M., Wang, C., Desai, N., Guo, J., and Han, J.
(2013). Kert: Automatic extraction and ranking of
topical keyphrases from content-representative docu-
ment titles. arXiv preprint arXiv:1306.0271.
DAvanzo, E., Magnini, B., and Vallin, A. (2004).
Keyphrase extraction for summarization purposes:
The lake system at duc-2004. In Proceedings of the
2004 document understanding conference.
De Nart, D. and Tasso, C. (2014). A domain independent
double layered approach to keyphrase generation. In
WEBIST 2014 - Proceedings of the 10th International
Conference on Web Information Systems and Tech-
nologies, pages 305–312. SCITEPRESS Science and
Technology Publications.
El-Beltagy, S. R. and Rafea, A. (2009). Kp-miner: A
keyphrase extraction system for english and arabic
documents. Information Systems, 34(1):132–144.
Fagan, J. (1987). Automatic phrase indexing for document
retrieval. In Proceedings of the 10th Annual Interna-
tional ACM SIGIR Conference on Research and De-
velopment in Information Retrieval, SIGIR ’87, pages
91–101, New York, NY, USA. ACM.
Ferragina, P. and Scaiella, U. (2010). Tagme: On-the-fly
annotation of short text fragments (by wikipedia enti-
ties). In Proceedings of the 19th ACM International
Conference on Information and Knowledge Manage-
ment, CIKM ’10, pages 1625–1628, New York, NY,
USA. ACM.
Frank, E., Paynter, G. W., Witten, I. H., Gutwin, C., and
et al. (1999). Domain-specific keyphrase extraction.
In Proc. Sixteenth International Joint Conference on
Artificial Intelligence, pages 668–673. Morgan Kauf-
mann Publishers.
Hulth, A. (2003). Improved automatic keyword extraction
given more linguistic knowledge. In Proceedings of
the 2003 Conference on Empirical Methods in Nat-
ural Language Processing, EMNLP ’03, pages 216–
223, Stroudsburg, PA, USA. Association for Compu-
tational Linguistics.
Krapivin, M., Marchese, M., Yadrantsau, A., and Liang,
Y. (2008). Unsupervised key-phrases extraction from
scientific papers using domain and linguistic knowl-
edge. In Digital Information Management, 2008.
ICDIM 2008. Third International Conference on,
pages 105–112.
Litvak, M., Last, M., and Friedman, M. (2010). A new ap-
proach to improving multilingual summarization us-
ing a genetic algorithm. In Proceedings of the 48th
Annual Meeting of the Association for Computational
Linguistics, pages 927–936. Association for Compu-
tational Linguistics.
Liu, Z., Li, P., Zheng, Y., and Sun, M. (2009). Cluster-
ing to find exemplar terms for keyphrase extraction.
In Proceedings of the 2009 Conference on Empirical
Methods in Natural Language Processing: Volume 1 -
Volume 1, EMNLP ’09, pages 257–266, Stroudsburg,
PA, USA. Association for Computational Linguistics.
Matsuo, Y. and Ishizuka, M. (2004). Keyword extraction
from a single document using word co-occurrence sta-
tistical information. International Journal on Artifi-
cial Intelligence Tools, 13(01):157–169.
Paukkeri, M.-S., Nieminen, I. T., P
¨
oll
¨
a, M., and Honkela,
T. (2008). A language-independent approach to
KDIR2014-InternationalConferenceonKnowledgeDiscoveryandInformationRetrieval
84