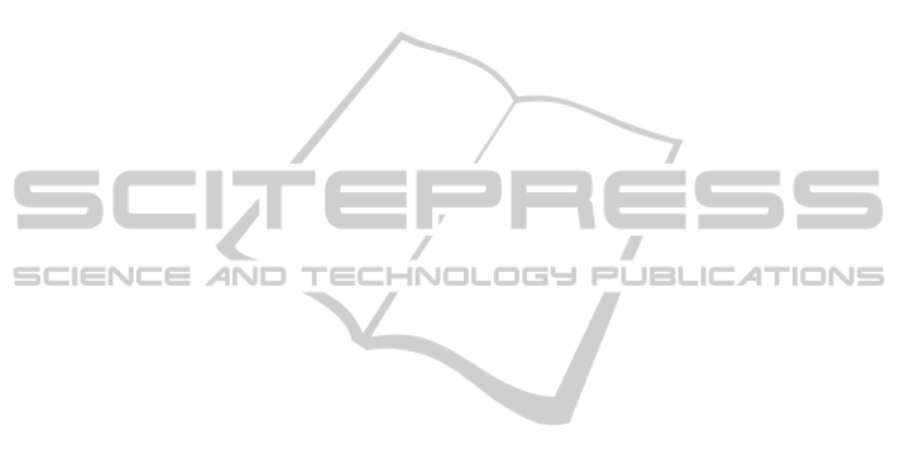
M. P., Montesanto A.). (Edizioni Kappa, Roma 1996)
977–982.
Grim, J. (1999). Information approach to structural opti-
mization of probabilistic neural networks, In Proc. 4th
System Science European Congress, Eds. Ferrer, L. et
al., Valencia: Soc. Espanola de Sistemas Generales,
pp. 527-540.
Grim, J. (1999b). A sequential modification of EM al-
gorithm, In Studies in Classification, Data Analysis
and Knowledge Organization, Eds. Gaul W., Locarek-
Junge H., Springer 1999, pp. 163 - 170.
Grim, J. (2006). EM cluster analysis for categorical data, In
Structural, Syntactic and Statistical Pattern Recogni-
tion. Eds. Yeung D. Y., Kwok J. T., Fred A., Springer:
Berlin, LNCS 4109, pp. 640-648.
Grim, J. (2007). Neuromorphic features of probabilistic
neural networks. Kybernetika, Vol. 43, No. 5, pp.697-
712. http://dml.cz/dmlcz/135807
Grim, J. (2014). Sequential pattern recognition by maxi-
mum conditional informativity, Pattern Recognition
Letters, Vol. 45C, pp. 39-45.
http:// dx.doi.org/10.1016/j.patrec.2014.02.024
Grim, J., Haindl, M., Somol, P. and P. Pudil (2006). A sub-
space approach to texture modelling by using Gaus-
sian mixtures, In Proceedings of the 18th IAPR In-
ternational Conference on Pattern Recognition ICPR
2006, Eds. B. Haralick, T.K. Ho, Los Alamitos, IEEE
Computer Society, pp. 235-238.
Grim, J. and Hora, J. (2008). Iterative principles of recog-
nition in probabilistic neural networks, Neural Net-
works. Vol. 21, No. 6, pp. 838-846.
Grim, J. and Hora, J. (2009). Recognition of Properties
by Probabilistic Neural Networks, In Artificial Neu-
ral Networks - ICANN 2009, Springer: Berlin, LNCS
5769, pp. 165-174.
Grim, J. and Hora, J. (2010). Computational Properties
of Probabilistic Neural Networks, In Artificial Neu-
ral Networks - ICANN 2010 Part II, Springer: Berlin,
LNCS 5164, pp. 52-61.
Grim, J., Hora, J., Boˇcek P., Somol, P. and Pudil, P. (2010).
Statistical Model of the 2001 Czech Census for Inter-
active Presentation, Journal of Official Statistics. Vol.
26, No. 4, pp. 673694. http://ro.utia.cas.cz/dem.html
Grim, J., Kittler, J., Pudil, P. and Somol, P. (2002). Multi-
ple classifier fusion in probabilistic neural networks,
Pattern Analysis and Applications, Vol. 5, No. 7, pp.
221-233.
Grim, J., Pudil, P. and Somol, P. (2000). Recognition of
handwritten numerals by structural probabilistic neu-
ral networks, In Proceedings of the Second ICSC Sym-
posium on Neural Computation, Berlin, 2000. (Bothe
H., Rojas R. eds.). ICSC, Wetaskiwin, pp. 528-534.
Grim, J., Pudil, P. and Somol, P. (2002b). Boosting in proba-
bilistic neural networks, In Proceedings of the 16th In-
ternational Conference on Pattern Recognition, (Kas-
turi R., Laurendeau D., Suen C. eds.). IEEE Computer
Society, Los Alamitos, pp. 136–139.
Grim, J., Somol, P., Haindl, M. and Daneˇs, J. (2009).
Computer-Aided Evaluation of Screening Mammo-
grams Based on Local Texture Models, IEEE Trans.
on Image Processing, Vol. 18, No. 4, pp. 765-773.
Hasselblad, V. (1966). Estimation of prameters for a mix-
ture of normal distributions, Technometrics, Vol. 8, pp.
431-444.
Hasselblad, V. (1969). Estimation of finite mixtures of dis-
tributions from the exponential family, Journal of
Amer. Statist. Assoc., Vol. 58, pp. 1459-1471.
Hebb, D.O. (1949). The Organization of Behavior: A Neu-
ropsychological Theory, (New York: Wiley 1949).
Hosmer Jr, D.W. (1973). A comparison of iterative maxi-
mum likelihood estimates of the parameters of a mix-
ture of two normal distributions under three different
types of sample, Biometrics, pp. 761-770.
Kirshner, S. and Smyth, P. (2007). Infinite mixtures of trees,
In Proceedings of the 24th International Conference
on Machine Learning (ICML’07), Ed. Zoubin Ghahra-
mani, ACM, New York, USA, pp. 417-423.
Kruskal, J.B. (1956). On the shortest spanning sub-tree of a
graph, Proc. Amer. Math. Soc., No. 7, pp. 48-50.
Kullback, S. and Leibler, R.A. (1951). On Information and
Sufficiency, The Annals of Mathematical Statistics,
Vol. 22, No. 1, pp. 79-86.
Lowd, D. and Domingos, P. (2005). Naive Bayes models
for probability estimation, In Proceedings of the 22nd
international conference on machine learning, ACM
2005, pp. 529-536.
Markley, S.C. and Miller, D.J. (2010). Joint parsimonious
modeling and model order selection for multivariate
Gaussian mixtures, IEEE Journal of Selected Topics
in Signal Processing, Vol. 4, No. 3, pp. 548-559.
Meila, M. and Jordan, M.I. (1998). Estimating dependency
structure as a hidden variable, In Proceedings of the
1997 Conference on advances in neural information
processing systems 10, pp. 584-590.
Meila, M. and Jaakkola T. (2000). Tractable Bayesian
Learning of Tree Belief Networks, In Proceedings of
the 16th Conference on Uncertainty in Artificial Intel-
ligence, pp. 380-388.
Meila, M. and Jordan, M.I. (2001). Learning with mixtures
of trees, Journal of Machine Learning Research, Vol.
1, No. 9, pp. 1-48.
Prim, R.C. (1957). Shortest connection networks and some
generalizations, Bell System Tech. J., Vol. 36 , pp.
1389-1401.
Schlesinger, M.I. (1968). Relation between learning and
self learning in pattern recognition, (in Russian),
Kibernetika, (Kiev), No. 2, pp. 81-88.
Vajda, I. Theory of statistical inference and information,
Kluwer Academic Publishers (Dordrecht and Boston),
1989.
Wolfe, J.H. (1970). Pattern clustering by multivariate mix-
ture analysis, Multivariate Behavioral Research, Vol.
5, pp. 329-350.
NCTA2014-InternationalConferenceonNeuralComputationTheoryandApplications
74