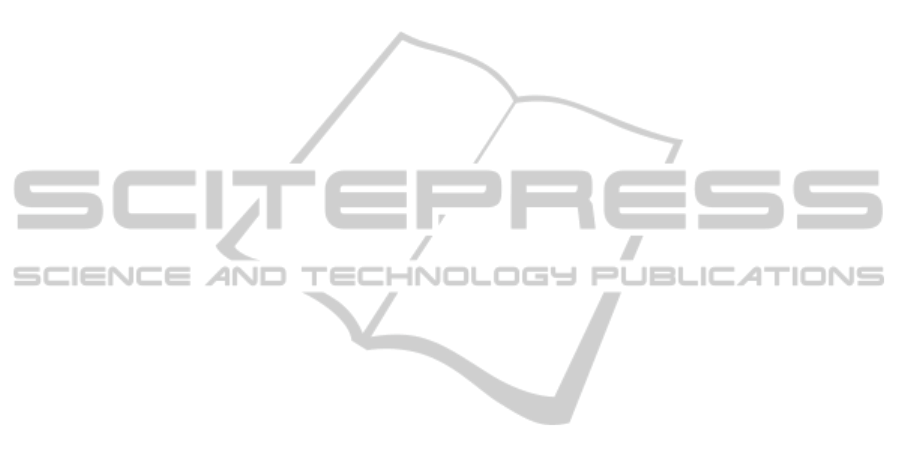
5 CONCLUSIONS
The first experiment (basic algorithm using f
1
)
shows that the sensitivity has a very high value
(0.941) for the group “hearing losses” (larger group),
and an average value equal to 0.447. The pathology
“3 - musculoskeletal disorders” was never predicted
by the algorithm. The specificity presents a value
close to 0.6 for the group “hearing loss” and an
average value greater than 0.9. These first results
show that function f
1
privileges the most frequent
pathology. In the second experiment (basic
algorithm using f
2
), the sensitivity no longer has null
values. The average sensitivity is equal to 0.461,
value slightly better than the previous case. The
specificity has values similar to the ones in the
previous experiment. The third experiment (variant
of the basic algorithm using f
1
) shows performance
values in general slightly worse compared to the
basic algorithm. However, there is an improvement
for the sensitivity of some pathologies. The fourth
experiment (variant of the basic algorithm using f
2
)
shows that the use of f
2
compared to f
1
has led to an
improvement of the average sensitivity of almost a
decimal point. Regarding the specificity, for all
pathologies the values are always greater than 0.84,
with an average value of 0.901.
The comparison of the average values of the
indicators (Table 18) shows how the second
algorithm with f
2
present the highest sensitivity.
Regarding the specificity and the predictive value of
the negative outcome of the test, we have
substantially similar behaviours for the four
experiments. As concerns positive predictive value,
the basic algorithm with f
2
has provided the best
results. The high values, close to unity, for
specificity and negative predictive value are
encouraging. However, the variant of the algorithm,
while not showing results appreciably better than the
basic algorithm, has better performance by reducing
the execution time to a third compared to the basic
version, because clustering procedures are run on
smaller sets. Thus, for the final commitment of the
system, which has to deal with a much larger
database, the second version should be preferred,
considering also that its performances are very close
to the ones obtained with the basic algorithm. In
particular, as shown by standard deviations in table
19, performances are stable over multiple runs,
assuring a good reliability to the results. Moreover,
the negative predictive value can be considered
sufficient to be used in a suited automatic screening
procedure, designed to reduce costs in performing
clinical trials on all the interested workers, since a
negative classification for a given worker is
sufficient to reliably ascertain his health status. Note
that in general for the groups “hearing loss” (the
largest group) and “tumors of the pleura and
peritoneum” (more severe disease) the results are
better than for other diseases, including the
sensitivity and the positive predictive value.
The examination of the weights of the features
(Table 16) shows different values for the different
algorithms. In all the experiments, only the
economic activity of the company seems less
important than the other features, so it might be
interesting to define a different set of features,
replacing the economic activity.
REFERENCES
A. K. Jane, R. C. Dubes, 1988. Algorithms for Clustering
Data, Prentice-Hall. Englewood Cliffs.
Alexandr A. Savinov, 1999. Mining Possibilistic Set-
Valued Rules by Generating Prime Disjunctions. In
PKDD'99, 3rd European Conference on Principles
and Practice of Knowledge Discovery in Databases.
Vol. 1704 Springer (1999), p. 536-541.
Chinmoy Mukherjee, Komal Gupta, Rajarathnam
Nallusamy, 2012. A Decision Support System for
Employee Healthcare. In Third International
Conference on Services in Emerging Markets.
Kumara Sastry, David Goldberg, Graham Kendall, 2005.
Genetic Algorithms. In Search Methodologies,
Springer US.
Razan Paul, Abu Sayed Md. Latiful Hoque, 2010.
Clustering Medical Data to Predict the Likelihood of
Diseases, IEEE - Digital Information Management
(ICDIM), Fifth International Conference.
Zhaohui Huang Daoheng Yu Jianye Zhao, 2000.
Application of Neural Networks with Linear and
Nonlinear Weights in Occupational Disease Incidence
forecast. In Circuits and systems. IEEE APCCAS
2000.
OccupationalDiseasesRiskPredictionbyClusterAnalysisandGeneticOptimization
75