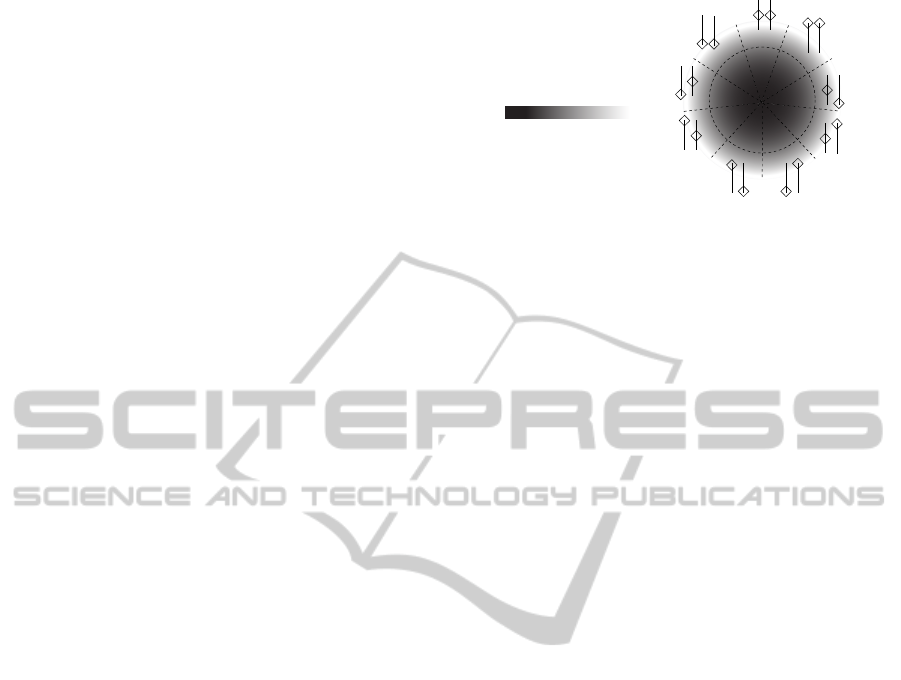
a sequence, the first CDM is related to the Chloe’s
best evaluated cookie, i.e. cookie 2 (see Table 1), and
the second to her worst’s, i.e., cookie 4. Thus, a se-
quence corresponding to Chloe-vs.-Bob comparison
(see Figure 3(c)) is “⋄|
⋄
|”, and a sequence correspond-
ing to Chloe-vs.-Alice comparison (see Figure 3(d))
is “⋄|
⋄
|”. Looking at these sequences, Chloe could hint
that neither Bob nor Alice remember a Grandma’s
cookie with no icing as she does. Due to these se-
quences allow Alice or Chloe to hint about a differ-
ence in connotations of a Grandma’s cookie, we call
any of them a connotation-differential print (CDP).
As it could be noticed above, a CDP depends on
the individual point of view of each cousin. In fact,
Alice has chosen the CDMs corresponding to cookie 4
and cookie 3, while Chloe has chosen cookie 2 and
cookie 4. This is an example of directionality and
asymmetry in comparison judgments pointed out by
Tversky in (Tversky, 1977). Furthermore, Alice could
assign a weight to each CDP in order to determine
which cousin’s referent is more similar to hers. For
example, according to her strategy to build a CDP, ⋄|⋄|
denotes a good match, thus, she assigns 1.0 to it.
⋄
|
⋄
|
and
⋄
|
⋄
| denote a not too bad match (they could become
⋄|⋄| by increasing δ) therefore, she gives 0.75 to them.
⋄
|⋄|, ⋄|
⋄
|,
⋄
|⋄| and ⋄|
⋄
| denote a big difference, so, she gives
0.25 to them. Finally,
⋄
|
⋄
| and
⋄
|
⋄
| denote a huge differ-
ence, so, she assigns 0 to them.
At this point, we could use a CDP to extend (9) to
perform a meaningful similarity comparison between
two IFSs and, therefore, to achieve better fine-tuned
and more reliable results. As an analogy, if (9) tells
us about how far is A from B, the use of a CDP tells
us in which “direction” B is in relation to A. With
this analogy, Figure 4 shows the similarity between
evaluations given by Alice and Bob, S
0
(A,B) = 0.8,
and so between Alice and Chloe’s, S
0
(A,C) = 0.8. In
Figure 4(a), using just the magnitude, there is no dif-
ference between both similarities; in contrast, in Fig-
ure 4(b), using the magnitude plus a CDP, it is note-
worthy how the “direction” of Alice vs. Bob differs
from Alice vs. Chloe’s. Hence, from Alice’s point of
view, the extended similarity between her evaluations
and Bob’s is h0.8, ⋄|⋄|i, and the corresponding one in
comparison to Chloe’s evaluations is h0.8,
⋄
|⋄|i. More-
over, using the weights that Alice proposed earlier, we
could say that Alice-vs.-Bob’s h0.8, ⋄|⋄|i is 0.8·1= 0.8,
and Alice-vs.-Chloe’s h0.8,
⋄
|⋄|i is 0.8·0.25= 0.2. This
reflects that, considering the individual connotation of
a Grandma’s cookie, the similarity between Alice and
Bob’s evaluations is better.
Using the previousanalogy, it is also possible to il-
lustrate how using a CDP as supplement could denote
in a better way an observed similarity. In the example
(a)
(b)
Figure 4: Alice vs. Bob and Alice vs. Chloe similarities.
presented by Tversky in (Tversky, 1977) it is stated
that: “considering the similarity between countries,
Jamaica is similar to Cuba (because of geographical
proximity), Cuba is similar to Russia (because of their
political affinity), but Jamaica and Russia are not sim-
ilar at all”, one may notice that both comparisons,
Jamaica vs. Cuba and Cuba vs. Russia, have simi-
lar magnitudes in their corresponding similarity mea-
sures, but different connotations: the first one focus-
ing on a “geographical proximity”-feature, and the
second one, on a “political affinity”-feature. Using
such a representation of the difference in connota-
tions, one may observe the reason why Jamaica and
Russia are not similar at all. Maybe, someone might
argue here that the same properties should be used
in both comparisons. However, as was already men-
tioned in Section 1, it is not feasible (nor practical) in
some contexts (e.g., when a crowdsourcing model is
used) clarifying all the properties that an object may
have. Indeed, this is why (fuzzy) human evaluations
are needed in those contexts.
7 AN ADVANTAGE
This section compares our meaningful similarity mea-
sure with others in order to show how it could over-
come some difficulties such as those pointed out in
(Szmidt and Kacprzyk, 2013).
7.1 Some Difficulties in Geometric
Similarity Measures for IFSs
Using a geometric interpretation of the three terms
in an IFS-element (i.e., the membership, non-
membership and hesitation margin), the similarity be-
tween two IFSs is usually assumed to be a dual notion
of a metric distance (Szmidt and Kacprzyk, 2013).
Thus, given two IFSs A,B ∈ X and a normalized met-
ric distance function l : X
2
7→ [0,1], the similarity S
between A and B is expressed as S(A, B) = 1−l(A,B),
FCTA2014-InternationalConferenceonFuzzyComputationTheoryandApplications
134