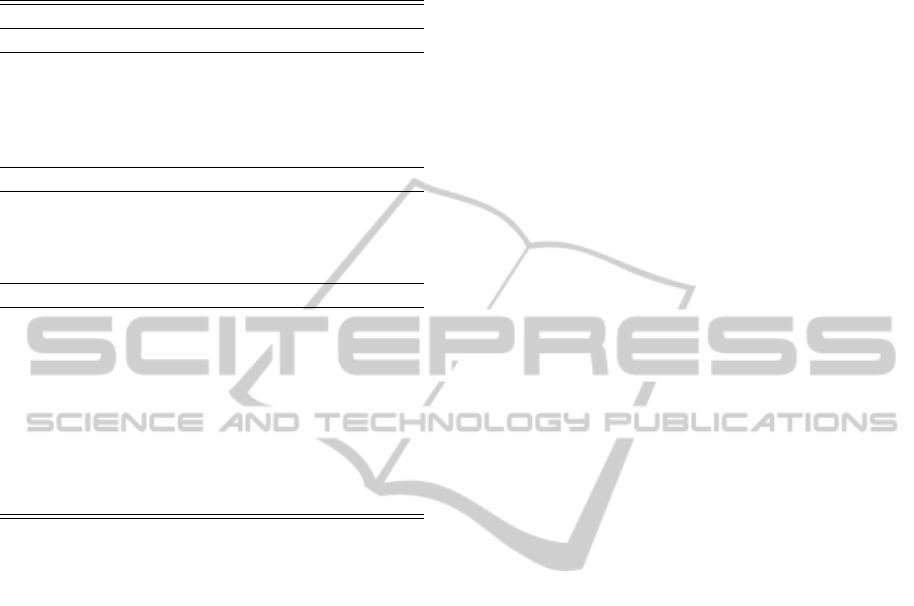
Table 5: Complexity measures computed for the differ-
ent decompositions of automobile, eucalyptus and machine
datasets.
D
i
Weights Complexity measures
automobile δ
i
F1 F3 L1 N1
D
1
0.0000 13.41 0.99 0.04 0.05
D
2
0.0142 13.04 0.46 0.29 0.15
D
3
0.2200 0.83 0.22 0.43 0.22
D
4
0.0538 2.93 0.26 0.58 0.28
D
5
0.1950 0.96 0.57 0.33 0.16
eucalyptus δ
i
F1 F3 L1 N1
D
1
0.0191 2.72 0.17 0.40 0.20
D
2
0.3745 1.48 0.21 0.51 0.24
D
3
0.1235 1.80 0.10 0.58 0.31
D
4
0.0144 2.72 0.30 0.34 0.22
machine δ
i
F1 F3 L1 N1
D
1
0.2172 0.66 0.57 0.20 0.17
D
2
0.0204 0.89 0.43 0.42 0.19
D
3
0.0075 0.82 0.39 0.61 0.23
D
4
0.0209 0.97 0.30 0.77 0.26
D
5
0.0044 1.01 0.22 0.74 0.23
D
6
0.0104 1.13 0.22 0.63 0.23
D
7
0.0024 1.45 0.37 0.50 0.12
D
8
0.0115 1.80 0.45 0.39 0.09
D
9
0.5043 2.04 0.80 0.25 0.05
the computational complexity of our method (as ker-
nel learning methods usually present a high computa-
tional cost in this sense) and try to alleviate it via the
Nymstr
¨
on method for approximating Gram matrices
(Drineas and Mahoney, 2005).
ACKNOWLEDGEMENTS
This work has been subsidized by the TIN2011-22794
project of the Spanish Ministerial Commission of Sci-
ence and Technology (MICYT), FEDER funds and
the P11-TIC-7508 project of the “Junta de Andaluc
´
ıa”
(Spain).
REFERENCES
Baccianella, S., Esuli, A., and Sebastiani, F. (2009). Evalu-
ation measures for ordinal regression. In Proceedings
of the Ninth International Conference on Intelligent
Systems Design and Applications (ISDA 09), pages
283–287, Pisa, Italy.
Cardoso, J. S. and da Costa, J. F. P. (2007). Learning to clas-
sify ordinal data: The data replication method. Jour-
nal of Machine Learning Research, 8:1393–1429.
Chapelle, O., Vapnik, V., Bousquet, O., and Mukherjee,
S. (2002). Choosing multiple parameters for support
vector machines. Machine Learning, 46(1-3):131–
159.
Chu, W. and Ghahramani, Z. (2005). Gaussian processes
for ordinal regression. Journal of Machine Learning
Research, 6:1019–1041.
Chu, W. and Keerthi, S. S. (2007). Support vector ordinal
regression. Neural Computation, 19(3):792–815.
Cortes, C., Mohri, M., and Rostamizadeh, A. (2012).
Algorithms for learning kernels based on centered
alignment. Journal of Machine Learning Research,
13:795–828.
Cortes, C. and Vapnik, V. (1995). Support-vector networks.
Machine Learning, 20(3):273–297.
Cristianini, N., Kandola, J., Elisseeff, A., and Shawe-
Taylor, J. (2002). On kernel-target alignment. In Ad-
vances in Neural Information Processing Systems 14,
pages 367–373. MIT Press.
Drineas, P. and Mahoney, M. W. (2005). On the
nyström method for approximating a gram ma-
trix for improved kernel-based learning. J. Mach.
Learn. Res., 6:2153–2175.
Frank, E. and Hall, M. (2001). A simple approach to ordi-
nal classification. In Proc. of the 12th Eur. Conf. on
Machine Learning, pages 145–156.
Friedrichs, F. and Igel, C. (2005). Evolutionary tuning of
multiple svm parameters. Neurocomputing, 64:107–
117.
Guti
´
errez, P. A., P
´
erez-Ortiz, M., Fernandez-Navarro,
F., S
´
anchez-Monedero, J., and Herv
´
as-Mart
´
ınez, C.
(2012). An Experimental Study of Different Ordi-
nal Regression Methods and Measures. In 7th Inter-
national Conference on Hybrid Artificial Intelligence
Systems (HAIS), volume 7209 of Lecture Notes in
Computer Science, pages 296–307.
Ho, T. K. and Basu, M. (2002). Complexity measures of su-
pervised classification problems. IEEE Trans. Pattern
Anal. Mach. Intell., 24(3):289–300.
Hsu, C.-W. and Lin, C.-J. (2002). A comparison of methods
for multi-class support vector machines. IEEE Trans-
action on Neural Networks, 13(2):415–425.
Igel, C., Glasmachers, T., Mersch, B., Pfeifer, N., and
Meinicke, P. (2007). Gradient-based optimization of
kernel-target alignment for sequence kernels applied
to bacterial gene start detection. IEEE/ACM Trans.
Comput. Biol. Bioinformatics, 4(2):216–226.
Li, L. and Lin, H.-T. (2007). Ordinal Regression by Ex-
tended Binary Classification. In Advances in Neural
Inform. Processing Syst. 19.
P
´
erez-Ortiz, M., Guti
´
errez, P., Cruz-Ram
´
ırez, M., S
´
anchez-
Monedero, J., and Herv
´
as-Mart
´
ınez, C. (2013). Ker-
nelizing the proportional odds model through the em-
pirical kernel mapping. In Rojas, I., Joya, G., and
Gabestany, J., editors, Advances in Computational In-
telligence, volume 7902 of Lecture Notes in Computer
Science, pages 270–279. Springer Berlin Heidelberg.
P
´
erez-Ortiz, M., Guti
´
errez, P. A., S
´
anchez-Monedero, J.,
and Herv
´
as-Mart
´
ınez, C. (2013). Multi-scale Sup-
port Vector Machine Optimization by Kernel Target-
Alignment. In European Symposium on Artificial
NCTA2014-InternationalConferenceonNeuralComputationTheoryandApplications
224