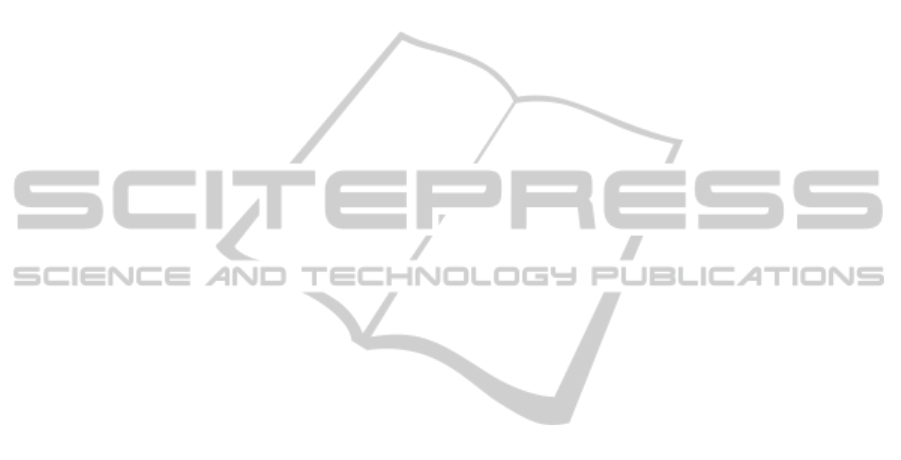
enhanced by explicit feedbacks, are definitely valu-
able and should be taken into account for ranking
opinions. For pragmatic reasons, our experiments in-
cluded news datasets having similar structures. How-
ever, exploring other datasets of different types of en-
tities, of users, and kinds of opinions is worthwhile in
order to show the wide applicability of our model. To
this end, we are planning to assess the effectiveness of
our approach using a dataset crawled from Youtube,
which is more subject to noise. We are currently in-
vestigating these points for further improvements.
ACKNOWLEDGEMENTS
This work was supported by RARE project.
REFERENCES
Akram Al-Kouz, Ernesto William De Luca, S. A. (2011).
Latent semantic social graph model for expert discov-
ery in facebook. In 11th International Conference on
Innovative Internet Community Systems, page 269. GI
Edition.
Amiri, H. and Chua, T.-S. (2012). Mining sentiment termi-
nology through time. In Proceedings of the 21st ACM
international conference on Information and knowl-
edge management, CIKM ’12, pages 2060–2064, New
York, NY, USA. ACM.
Bespalov, D., Bai, B., Qi, Y., and Shokoufandeh, A. (2011).
Sentiment classification based on supervised latent n-
gram analysis. In Proceedings of the 20th ACM in-
ternational conference on Information and knowledge
management, CIKM ’11, pages 375–382, New York,
NY, USA. ACM.
Danescu-Niculescu-Mizil, C., Kossinets, G., Kleinberg, J.,
and Lee, L. (2009). How opinions are received by on-
line communities: a case study on amazon.com help-
fulness votes. In Proceedings of the 18th international
conference on World wide web, WWW ’09, pages
141–150, New York, NY, USA. ACM.
Dave, K., Lawrence, S., and Pennock, D. M. (2003). Min-
ing the peanut gallery: opinion extraction and seman-
tic classification of product reviews. In WWW, pages
519–528.
Ding, X., Liu, B., and Yu, P. S. (2008). A holistic lexicon-
based approach to opinion mining. In WSDM, pages
231–240.
Dragut, E. C., Yu, C., Sistla, P., and Meng, W. (2010). Con-
struction of a sentimental word dictionary. In Pro-
ceedings of the 19th ACM international conference on
Information and knowledge management, CIKM ’10,
pages 1761–1764, New York, NY, USA. ACM.
Gao, S. and Li, H. (2011). A cross-domain adaptation
method for sentiment classification using probabilis-
tic latent analysis. In Proceedings of the 20th ACM
international conference on Information and knowl-
edge management, CIKM ’11, pages 1047–1052, New
York, NY, USA. ACM.
Gerani, S., Carman, M. J., and Crestani, F. (2010).
Proximity-based opinion retrieval. In Proceedings of
the 33rd international ACM SIGIR conference on Re-
search and development in information retrieval, SI-
GIR ’10, pages 403–410, New York, NY, USA. ACM.
He, Y. (2010). Learning sentiment classification model
from labeled features. In Proceedings of the 19th ACM
international conference on Information and knowl-
edge management, CIKM ’10, pages 1685–1688, New
York, NY, USA. ACM.
Hong, Y., Lu, J., Yao, J., Zhu, Q., and Zhou, G. (2012).
What reviews are satisfactory: novel features for au-
tomatic helpfulness voting. In Proceedings of the 35th
international ACM SIGIR conference on Research and
development in information retrieval, pages 495–504.
ACM.
Jia, L., Yu, C., and Meng, W. (2009). The effect of negation
on sentiment analysis and retrieval effectiveness. In
Proceedings of the 18th ACM conference on Informa-
tion and knowledge management, CIKM ’09, pages
1827–1830, New York, NY, USA. ACM.
Kim, S., Pantel, P., Chklovski, T., and Pennacchiotti, M.
(2006). Automatically assessing review helpfulness.
In Proceedings of the 2006 Conference on Empirical
Methods in Natural Language Processing, pages 423–
430.
Lin, C. and He, Y. (2009). Joint sentiment/topic model
for sentiment analysis. In Proceedings of the 18th
ACM conference on Information and knowledge man-
agement, CIKM ’09, pages 375–384, New York, NY,
USA. ACM.
Lin, Z., Tan, S., and Cheng, X. (2011). Language-
independent sentiment classification using three com-
mon words. In Proceedings of the 20th ACM interna-
tional conference on Information and knowledge man-
agement, CIKM ’11, pages 1041–1046, New York,
NY, USA. ACM.
Liu, J., Cao, Y., Lin, C.-Y., Huang, Y., and Zhou, M. (2007).
Low-quality product review detection in opinion sum-
marization. In EMNLP-CoNLL, pages 334–342.
Liu, Y., Huang, X., An, A., and Yu, X. (2008). Modeling
and predicting the helpfulness of online reviews. In
Data Mining, 2008. ICDM’08. Eighth IEEE Interna-
tional Conference on, pages 443–452. IEEE.
Santos, R. L. T., He, B., Macdonald, C., and Ounis, I.
(2009). Integrating proximity to subjective sentences
for blog opinion retrieval. In Proceedings of the 31th
European Conference on IR Research on Advances
in Information Retrieval, ECIR ’09, pages 325–336,
Berlin, Heidelberg. Springer-Verlag.
Tsur, O. and Rappoport, A. (2009). Revrank: A fully unsu-
pervised algorithm for selecting the most helpful book
reviews. In International AAAI Conference on We-
blogs and Social Media.
Turney, P. D. (2002). Thumbs up or thumbs down? seman-
tic orientation applied to unsupervised classification
of reviews. In ACL, pages 417–424.
ExploitingSocialDebatesforOpinionRanking
259