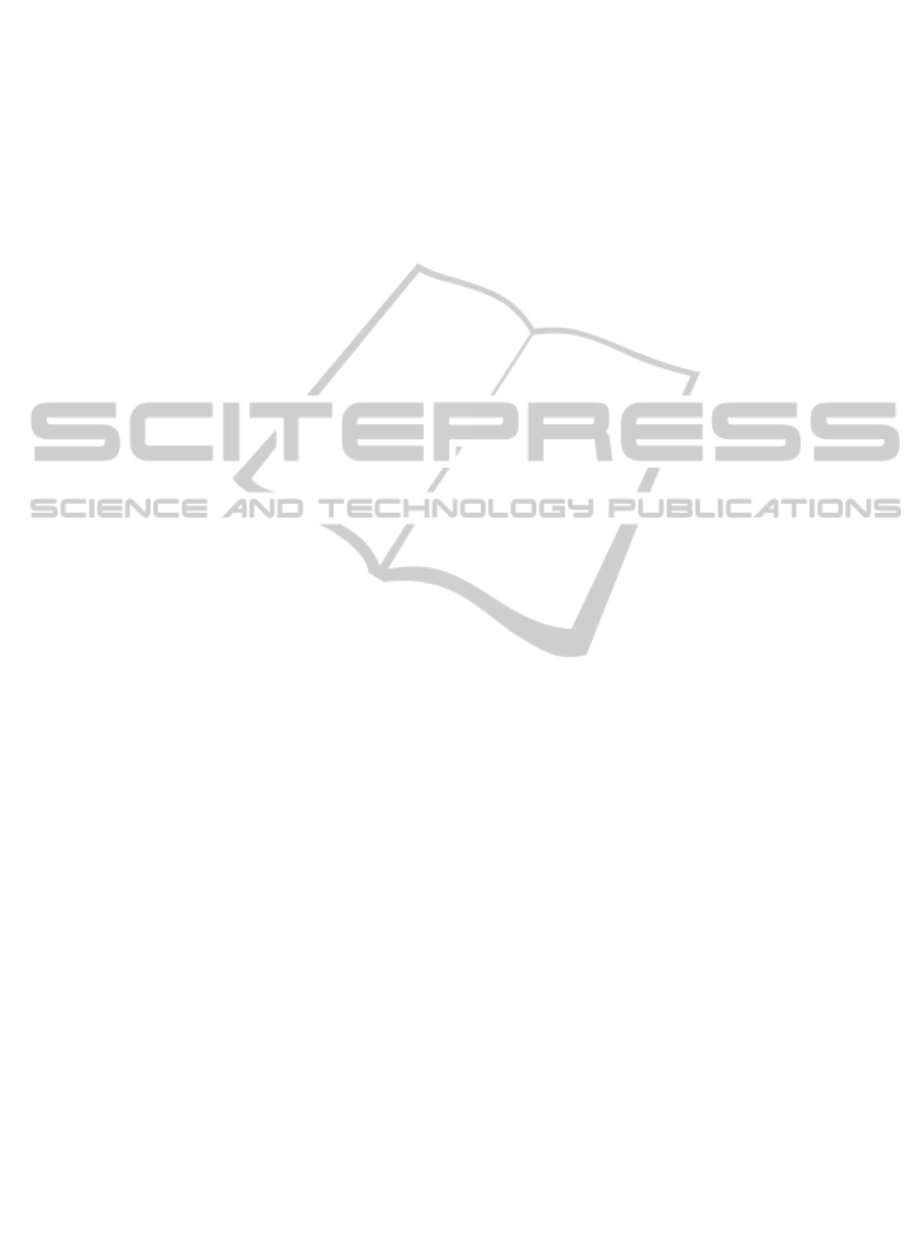
other critical units where the patients are in
continuous monitoring. In the other cases, solutions
presented in the literature can achieve this goal.
In the future additional variables will be
considered to understand how they can affect the
LOS of inpatients.
ACKNOWLEDGEMENTS
This work has been supported by FCT – Fundação
para a Ciência e Tecnologia in the scope of the
project: Pest-OE/EEI/UI0319/2014.
The authors would like to thank FCT
(Foundation of Science and Technology, Portugal)
for the financial support through the contract
PTDC/EEI-SII/1302/2012 (INTCare II).
REFERENCES
Azari, A., Janeja, V.P. & Mohseni, A., 2012. Predicting
Hospital Length of Stay (PHLOS): A Multi-tiered
Data Mining Approach. In 2012 IEEE 12th ICDB
Workshops. IEEE, pp. 17–24.
Breiman, L. et al., 1984. Classification and Regression
Trees no, ed., Belmont, CA: Wadsworth International
Group.
Caetano, N. et al., 2014. A Data-Driven Approach to
Predict Hospital Length of Stay: A Portuguese Case
Study. In ICEIS 2014. (Accepted to be published)
Cios, K. et al., 2007. Supervised Learning: Statistical
Methods. In A Knowledge Discovery Approach.
Springer US, pp. 307–386.
Clarke, A., 1996. Why are we trying to reduce length of
stay? Evaluation of the costs and benefits of reducing
time in hospital must start from the objectives that
govern change. Quality in health care: QHC, 5(3),
pp.172–9.
Dahan, H. et al., 2014. Proactive Data Mining with
Decision Trees, New York, NY: Springer New York.
Guy W., Rush J, e. a. (2000). Clinical Global Impressions
(CGI) Scale. Psychiatric Measures, APA.
Hachesu, P.R. et al., 2013. Use of data mining techniques
to determine and predict length of stay of cardiac
patients. Healthcare informatics research, 19(2),
pp.121–129.
Han, J., Kamber, M. & Pei, J., 2011. Data Mining:
Concepts and Techniques 3rd ed., San Francisco, CA,
USA: Morgan Kaufmann Publishers Inc.
Hand, D.J., Smyth, P. & Mannila, H., 2001. Principles of
data mining. Available at: http://dl.acm.org/citation.
cfm?id=500820 [Accessed May 5, 2014].
Isken, M.W. & Rajagopalan, B., 2002. Data Mining to
Support Simulation Modeling of Patient Flow in
Hospitals. Journal of Medical Systems, 26(2), pp.179–
197.
Kantardzic, M., 2011. Data Mining: Concepts, Models,
Methods, and Algorithms 2nd ed., Wiley-IEEE Press.
Marshall, A., Vasilakis, C. & El-Darzi, E., 2005. Length
of Stay-Based Patient Flow Models: Recent
Developments and Future Directions. Health Care
Management Science, 8(3), pp.213–220.
Michie, D., Spiegelhalter, D.J., et al., 1994. Machine
Learning, Neural and Statistical Classification. Ellis
Horwood.
Portela, F., Santos, M.F., Silva, Á., et al., 2013. Data
Mining for Real-Time Intelligent Decision Support
System in Intensive Care Medicine. In ICAART 2013
- International Conference on Agents and Artificial
Intelligence. pp. 270–276.
Portela, F., Santos, M.F., Machado, J., et al., 2013.
Pervasive and Intelligent Decision Support in Critical
Health Care Using Ensembles. In ITBAM. pp. 1–16.
Portela, F., Pinto, F. & Santos, M.F., 2012. Data Mining
Predictive Models for Pervasive Intelligent Decision
Support in Intensive Care Medicine. In KMIS. pp. 81–
88.
Quiland, J.R., 1986. Induction of Decision Tress. Machine
Learning, 1(1), pp.81.106.
Rokach, L. & Maimon, O., 2010. Supervised Learning. In
O. Maimon & L. Rokach, eds. Data Mining and
Knowledge Discovery Handbook. Springer US, pp.
133–147.
Santos, M.F. & Azevedo, C., 2005. Data Mining
Descoberta de conhecimento em base de dados, FCA -
Editora de Informática, Lda.
Santos, M.F., Portela, F. & Vilas-Boas, M., 2011.
INTCARE : multi-agent approach for real-time
intelligent decision support in intensive medicine.
Taylor, K., 2010. Oracle Data Mining Concepts.
Vapnik, V.N., 1995. The Nature of Statistical Learning
Theory 1st ed., Springer.
Wagener, G. et al., 2011. The Surgical Procedure
Assessment (SPA) score predicts intensive care unit
length of stay after cardiac surgery. The Journal of
thoracic and cardiovascular surgery, 142(2), pp.443–
50.
Wirth, R., & Hipp, J. (2000). CRISP-DM: Towards a
standard process model for data mining. Paper
presented at the 4th ICPAKDD.
Zhang, X.-C., Zhang, Z.-D. & Huang, D.-S., 2012.
Prediction of length of ICU stay using data-mining
techniques: an example of old critically Ill
postoperative gastric cancer patients. Asian Pacific
journal of cancer prevention: APJCP, 13(1), pp.97–
101.
KMIS2014-InternationalConferenceonKnowledgeManagementandInformationSharing
254