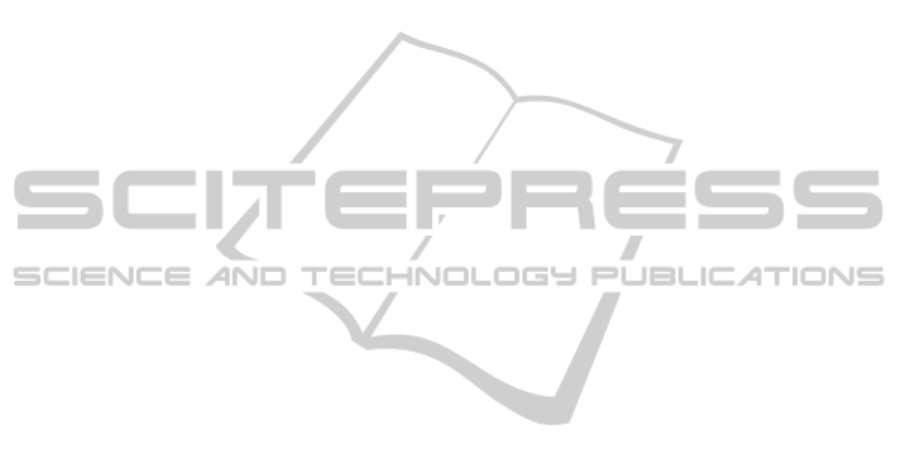
how the values may be visualised via heatmaps.
Further research would be required to fully evalu-
ate our approach and provide results including those
generated by clustering data using our fused dis-
tances. We will also need to compare our interme-
diary fusion approach with a late fusion approach us-
ing an ensemble clustering algorithm to perform the
clustering of complex objects.
REFERENCES
Berndt, D. J. and Clifford, J. (1996). Finding patterns in
time series: A dynamic programming approach. In
Fayyad, U. M., Piatetsky-Shapiro, G., Smyth, P., and
Uthurusamy, R., editors, Advances in Knowledge Dis-
covery and Data Mining, pages 229–248. American
Association for Artificial Intelligence, Menlo Park,
CA, USA.
Bettencourt-Silva, J., Iglesia, B. D. L., Donell, S., and
Rayward-Smith, V. (2011). On creating a patient-
centric database from multiple hospital information
systems in a national health service secondary care
setting. Methods of Information in Medicine, pages
6730–6737.
Bostr
¨
om, H., Andler, S. F., Brohede, M., Johansson, R.,
Karlsson, A., van Laere, J., Niklasson, L., Nilsson,
M., Persson, A., and Ziemke, T. (2007). On the defini-
tion of information fusion as a field of research. Tech-
nical report, Institutionen f
¨
or kommunikation och in-
formation.
Cormode, G. and McGregor, A. (2008). Approximation al-
gorithms for clustering uncertain data. In Proceed-
ings of the Twenty-seventh ACM SIGMOD-SIGACT-
SIGART Symposium on Principles of Database Sys-
tems, PODS ’08, pages 191–200, New York, NY,
USA. ACM.
Dimitriadou, E., Weingessel, A., and Hornik, K. (2002). A
combination scheme for fuzzy clustering. In Pal, N.
and Sugeno, M., editors, Advances in Soft Computing
AFSS 2002, volume 2275 of Lecture Notes in Com-
puter Science, pages 332–338. Springer Berlin Hei-
delberg.
Evrim, Rasmussen, M. A., Savorani, F., Ns, T., and Bro,
R. (2013). Understanding data fusion within the
framework of coupled matrix and tensor factoriza-
tions. Chemometrics and Intelligent Laboratory Sys-
tems, 129(9):53–63.
Goh, C. (1996). Representing and reasoning about semantic
conflicts. In In Heterogeneous Information System,
PhD Thesis, MIT.
Greene, D. and Cunningham, P. (2009). A matrix factoriza-
tion approach for integrating multiple data views. In
Buntine, W., Grobelnik, M., Mladeni, D., and Shawe-
Taylor, J., editors, Machine Learning and Knowl-
edge Discovery in Databases, volume 5781 of Lecture
Notes in Computer Science, pages 423–438. Springer
Berlin Heidelberg.
Henniger, O. and Muller, S. (2007). Effects of time normal-
ization on the accuracy of dynamic time warping. In
Biometrics: Theory, Applications, and Systems, 2007.
BTAS 2007. First IEEE International Conference on,
pages 1–6.
Jain, A. K., Murty, M. N., and Flynn, P. J. (1999). Data
clustering: A review. ACM Comput. Surv., 31(3):264–
323.
Kim, W. and Seo, J. (1991). Classifying schematic and data
heterogeneity in multidatabase systems. Computer,
24(12):12–18.
Laney, D. (2001). 3D data management: Controlling data
volume, velocity, and variety. Technical report, META
Group.
Long, B., Zhang, Z., Wu, X., and Yu, P. S. (2006). Spec-
tral clustering for multi-type relational data. In ICML,
pages 585–592.
Ma, H., Yang, H., Lyu, M. R., and King, I. (2008).
Sorec: Social recommendation using probabilistic
matrix factorization. In Proceedings of the 17th ACM
Conference on Information and Knowledge Manage-
ment, CIKM ’08, pages 931–940, New York, NY,
USA. ACM.
Maragos, P., Gros, P., Katsamanis, A., and Papandreou,
G. (2008). Cross-modal integration for performance
improving in multimedia: A review. In Maragos,
P., Potamianos, A., and Gros, P., editors, Multimodal
Processing and Interaction, volume 33 of Multimedia
Systems and Applications, pages 1–46. Springer US.
Pavlidis, P., Cai, J., Weston, J., and Noble, W. S. (2002).
Learning gene functional classifications from multi-
ple data types. Journal of Computational Biology,
9(2):401–411.
Ratanamahatana, C. A. and Keogh, E. (2005). Three myths
about dynamic time warping data mining. Proceed-
ings of SIAM International Conference on Data Min-
ing (SDM05), pages 506–510.
Skillicorn, D. B. (2007). Understanding Complex Datasets:
Data Mining with Matrix Decompositions. Chapman
and Hall/CRC, Taylor and Francis Group.
Strehl, A. and Ghosh, J. (2003). Cluster ensembles — a
knowledge reuse framework for combining multiple
partitions. J. Mach. Learn. Res., 3:583–617.
ˇ
Zitnik, M. and Zupan, B. (2014). Matrix factorization-based
data fusion for gene function prediction in baker’s
yeast and slime mold. Systems Biomedicine, 2:1–7.
van Vliet, M. H., Horlings, H. M., van de Vijver, M. J.,
Reinders, M. J. T., and Wessels, L. F. A. (2012). In-
tegration of clinical and gene expression data has a
synergetic effect on predicting breast cancer outcome.
PLoS ONE, 7(7):e40358.
Yu, S., Falck, T., Daemen, A., Tranchevent, L.-C., Suykens,
J., De Moor, B., and Moreau, Y. (2010). L2-norm mul-
tiple kernel learning and its application to biomedical
data fusion. BMC Bioinformatics, 11(1).
Zeng, H.-J., Chen, Z., and Ma, W.-Y. (2002). A unified
framework for clustering heterogeneous web objects.
In Web Information Systems Engineering, 2002. WISE
2002. Proceedings of the Third International Confer-
ence, pages 161–170.
KDIR2014-InternationalConferenceonKnowledgeDiscoveryandInformationRetrieval
276