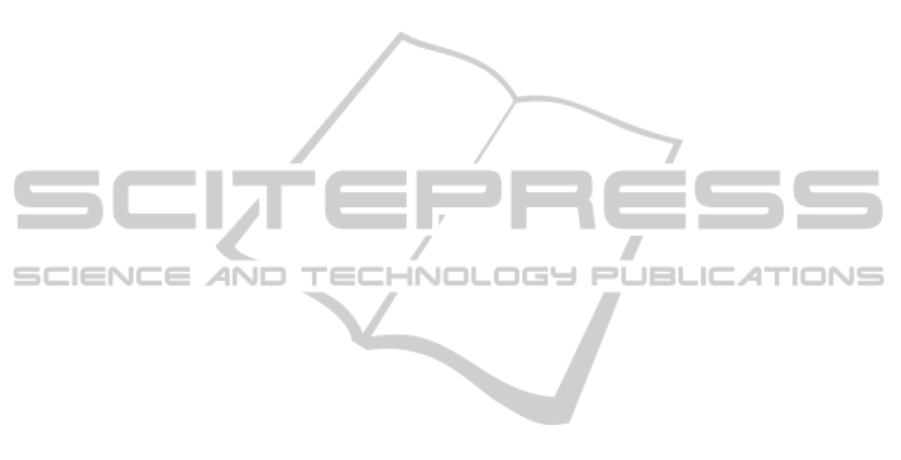
only the cluster with the best evaluation, i.e. the high-
est value, while other decision-makers prefer to select
a group of the top clusters. Within this example, the
decision-maker had selected the “top 5” clusters rep-
resenting relevant opinions from his point of view.
It is worth to mention that within this example, the
decision-maker started with 120 opinions gathered
from social-media that were grouped into 50 clusters.
Processing these clusters based on this proposal, al-
lowed the decision-maker to select the top 5 clusters
representing relevant opinions taking into account the
cohesion and the representativeness of the clusters.
One of the advantages of the presented approach
is that the flexibility in the LSP method allows the
decision-maker, changing the definition of elemen-
tary criteria, in order to select relevant opinions that
best suits his/her point of view. One remark within
this respect is that the number of noticeable opin-
ions, considered as a component of the representa-
tiveness, might be extended in order to represent dif-
ferent levels of importance (e.g., low, intermediate,
high, etc.) among experts. In this case, it is possible
to assign different weights to each expert opinion and
its normalization will become part of the criteria def-
inition. In a similar way the number of membership
functions could be replaced by the relative number of
membership functions, considering the total number
of present opinions.
In order to validate the results of this proposal, five
experts of the soft-computingarea, were asked to rank
a small selection of clusters (i.e., eight clusters from
the original group of 50) based on the cohesion and
the number of membership functions. Here, all the ex-
perts had the same selection for the top 4 and the clus-
ter with the lowest overall evaluation, but the order
of the other intermediate clusters were slightly differ-
ent. Based on the computation described in this pro-
posal, those intermediate clusters had a slightly differ-
ent value in the overall evaluation, which justifies the
small differences among the experts. However, more
elaborated experiments should be performed, and are
subject of further study.
5 CONCLUSIONS
This paper proposed to evaluate relevant opinions
within a large number of expert opinions, expressed
as membership functions, that might be gathered
through social media. A shape-similarity method is
used to cluster similar preferences in order to reduce
the number of evaluations for different points of view.
The evaluation results from selecting the best combi-
nation of cohesion and representativeness in the avail-
able clusters from the decision-maker’s point of view.
The cohesion is a measure obtained from computing
the area among the upper and lower bounds of the
cluster compared to the total available area, while its
representativeness is given by aggregating the num-
ber of membership functions and the number of no-
ticeable opinions. In order to properly reflect the
decision-maker’s point of view this proposal uses the
LSP method that buildsa precise representativemodel
of logic aggregation of preferences.
The main advantage of this proposal is that it can
handle a large group of opinions gathered through
social media, where the preferences initially given
are not modified. Furthermore, it evaluates different
points of view separately (i.e., previously clustered)
and it permits the decision-maker to select the group
of opinions that best suits his/her choice (i.e., given as
preferences in the LSP method) based on the combi-
nation of some cluster profile characteristics. Within
this paper the cluster characteristics or attributes are a
cohesion measure, the relative number of membership
functions and the number of noticeable opinions.
We consider exploring some crowdsourceapplica-
tions as opportunities for future work, and evaluating
clusters of opinions with different strategies in order
to compare them with the presented approach are sub-
ject to further study as well.
ACKNOWLEDGEMENTS
This research is supported by Escuela Superior
Polit´ecnica del Litoral (ESPOL) and it is financed by
SENESCYT under Ph.D. studies 2014.
REFERENCES
Cabrerizo, F. J., Ure˜na, R., Pedrycz, W., and Herrera-
Viedma, E. (2014). Building consensus in group deci-
sion making with an allocation of information granu-
larity. Fuzzy Sets and Systems.
Dubois, D. and Prade, H. (1997). The three semantics of
fuzzy sets. Fuzzy Sets and Systems, 90:141–150.
Dubois, D., P. H. (2000). Fundamentals of Fuzzy Sets (THE
HANDBOOKS OF FUZZY SETS Volume 7). Springer.
Dujmovi´c, J. (2007). Continuous Preference Logic for Sys-
tem Evaluation. IEEE Transactions on Fuzzy Systems,
15(6):1082–1099.
Dujmovi´c, J. (2008). Characteristic forms of generalized
conjunction/disjunction. 2008 IEEE International
Conference on Fuzzy Systems (IEEE World Congress
on Computational Intelligence), pages 1075–1080.
EvaluatingRelevantOpinionswithinaLargeGroup
85