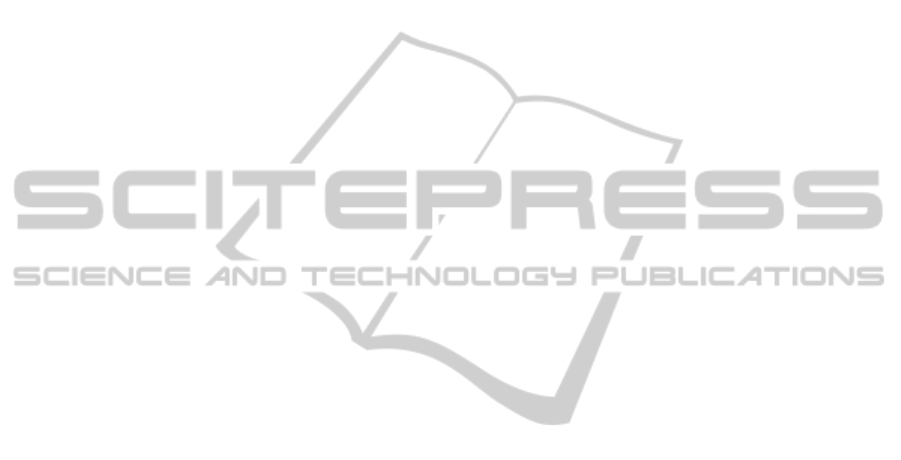
fMOVE poses technical advantages since it allows
high frequency data acquisition inside the fMRI
environment which is commonly incompatible to all
widely used motion tracking technologies, due to
the applied magnetic field. Our system is amenable
to further customization depending on the needs of
the experimental study, designed to be carried out
inside the scanner. Such customization can include
developing a multiple-marker tracking algorithm, so
as to increase the motion tracking accuracy, avoid
false marker detections and cover the motion of
multiple body parts or more complex behavioural
tasks.
Importantly, apart from its compatibility to the
fMRI environment, fMOVE constitutes an ultra-
low-cost motion tracking technology, that limits
expenses to the price of the used camera. At the
same time, the methodological platform it supports,
offers promising advantages for future studies of
motor behaviour (Wolpert, et.al., 2011, Wolpert and
Flanagan, 2010). It namely enables a tight
integration of psychophysical and functional
imaging studies and can thereby guide
investigations of the still unknown neural
foundation of cortical action selection and motor
learning rules.
REFERENCES
Abbott, W. W.; Faisal, A. A. (2012) Ultra-low-cost 3D
gaze estimation: an intuitive high information
throughput compliment to direct brain–machine
interfaces, Journal of Neural Engineering, 9
(4),046016
Abbott, W. W.; Zucconi, A.; Faisal, A. A.; Large-field
study of ultra low-cost, non-invasive task level bmi
(2013) IEEE/EMBS Conf. Proc. on Neural Eng.
(NER),723-726
Brayanov, J. B., Press, D. Z., and Smith, M. A. (2012).
Motor Memory Is Encoded as a Gain-Field
Combination of Intrinsic and Extrinsic Action
Representations. Journal of Neuroscience.
Faisal, A. A. , Selen, L. P. J. and Wolpert, D. M. (2008)
Noise in the nervous system, Nature Reviews
Neuroscience, 9 (4),292-303
Faisal, A. A. , Stout, D., Apel, J. and Bradley, B. (2010)
The manipulative complexity of Lower Paleolithic
stone toolmaking,PloS one, 5(11): e13718,2010
Fara, S.; Vikram, C. S. , Gavriel, C.; Faisal, A. A. Robust,
ultra low-cost mmg system with brain-machine-
interface applications (2013) IEEE/EMBS Conf. Proc.
on Neural Eng. (NER),723-726
Gavriel, C.; Faisal, A. A.; ",Wireless kinematic body
sensor network for low-cost neurotechnology
applications “in-the-wild” (2013) IEEE/EMBS Conf.
Proc. on Neural Eng. (NER), 1279-1282
Thomik, A. A. C.; Haber, D. ,Faisal, A. A. (2013); Real-
time movement prediction for improved control of
neuroprosthetic devices, IEEE/EMBS Conf. Proc. on
Neural Eng. (NER), 625-628
Hecht, E. E.; Gutman, D. A.; Khreisheh, N.; Taylor, S.
V.; Kilner, J.; Faisal, A. A.; Bradley, B .A.;
Chaminade, T.; Stout, D.; (2014) Acquisition of
Paleolithic toolmaking abilities involves structural
remodeling to inferior frontoparietal regions, Brain
Structure and Function 1863-2653, 1-17
Haber, D.; Thomik, A. A. C.; Faisal, A. A. (2014).
Unsupervised time series segmentation for high-
dimensional body sensor network data streams, IEEE
Conf Wearable and Implant. Body Sensor Networks
(BSN),121-126,
Friston, K. (2010). The free-energy principle: a unified
brain theory? Nature Reviews. Neuroscience, 11(2),
127–138.
Friston, K. J., Daunizeau, J., and Kiebel, S. J. (2009).
Reinforcement learning or active inference? PLoS
ONE, 4. doi:10.1371/journal.pone.0006421
Ingram, J., Körding, K. P. , Howard, I. S. and Wolpert, D.
M. (2008) The statistics of natural hand movements,
Experimental Brain Research 188 (2), 223-236
Kato, H. and Billinghurst, M., 1999. Marker Tracking and
HMD Calibration for a Video-based Augmented
Reality Conferencing System. In Augmented Reality,
1999.(IWAR'99) Proceedings. 2nd IEEE and ACM
International Workshop on, pp. 85-94.
Shadmehr, R., and Moussavi, Z. M. (2000). Spatial
generalization from learning dynamics of reaching
movements. The Journal of neuroscience : the official
journal of the Society for Neuroscience (Vol. 20, pp.
7807–7815). doi:20/20/7807 (pii)
Shadmehr, R., and Mussa-Ivaldi, F. A. (1994). Adaptive
representation of dynamics during learning of a motor
task. The Journal of Neuroscience : The Official
Journal of the Society for Neuroscience, 14, 3208–
3224. doi:8182467
Syladi A., Faisal A.A. (2012). What is the hierarchical
representation of tasks involving objects with
complex internal dynamics? Frontiers in
Computational Neuroscience.doi:10.3389/conf.fncom
.2012.55.00129
Todorov, E. (2004). Optimality principles in sensorimotor
control. Nature Neuroscience, 7, 907–915. doi:10.
1038/nn1309
Todorov, E., and Jordan, M. I. (2002). Optimal feedback
control as a theory of motor coordination. Nature
Neuroscience, 5, 1226–1235. doi:10.1038/nn963
Wolpert, D. M., Diedrichsen, J., and Flanagan, J. R.
(2011). Principles of sensorimotor learning. Nature
Reviews. Neuroscience, 12(12), 739–51. doi:10.1038/
nrn3112
Wolpert, D. M. and Flanagan, J. R., 2010. Motor
learning. Curr Biol, pp. 467-472.
NEUROTECHNIX2014-InternationalCongressonNeurotechnology,ElectronicsandInformatics
30