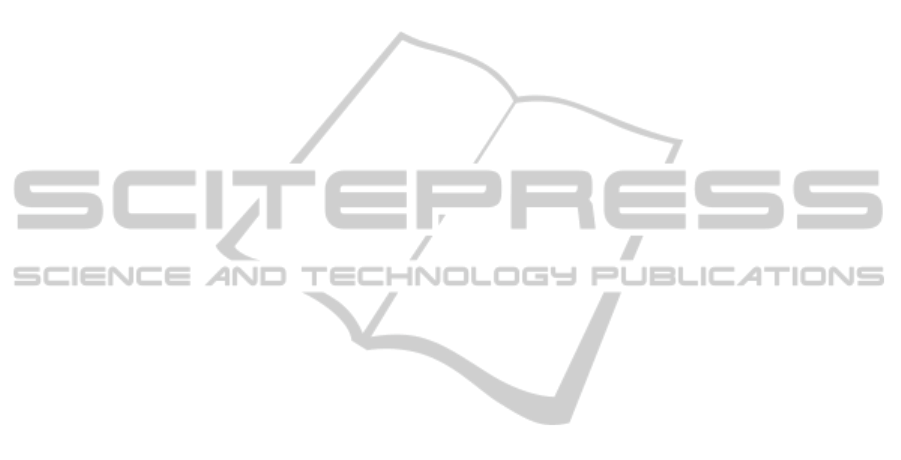
Using a segment length of 5000 is indeed a
good choice, since it leads to enough time resolution
for the time analysis of the coefficient changes.
Smaller segment sizes should be used carefully,
since the DFA method needs large number of signal
samples for the accurate estimation of the alpha
coefficients.
It should be pointed out that the practical clinical
framework of our article involves the application of
neuroprosthesis or continuous monitoring of critical
neurological patients. In both applications the
neurologist will not access the whole EEG data for
visually detecting HFOs, just because in the first
case recordings are not available to the external
environment, and in the second case the huge
amount of data prevents continuous visual analysis.
Consequently, data for the HFO detector was not
visually reviewed by a neurologist. In this context,
we can only assure that the detections pointed by the
HFO method depicts local increase in high
frequency activity of the brain, and may not be
directly related to the definition of pathological
HFO, used in most recent studies. Muscular artifacts
may also have been included as false positives for
high frequency brain activity, since they were not
treated in any specific way.
4 CONCLUSIONS
All classification groups were statistically
distinguished from one another. Notice that the
ability to separate the BS group from the D group is
really important as it suggests that it may be possible
to detect changes in HFO rate and long-term power
correlation of the EEG signal before the occurrence
of the epileptic seizure. The results also reinforce
previous researches, showing that the detection of
local increases on high frequency activity is related
to the occurrence of epileptic seizures (Engel, 2012).
Consequently, methods based on the Detrended
Fluctuation Analysis and HFO detection may
contribute to the development of seizure detection
algorithms. DFA methods may be even helpful to
locate the epileptogenic zone, through coefficient
variation maps generated along with MRI images.
Although there are previous studies
characterizing DFA analysis in identifying epileptic
seizures, this studies is novel in the scene of
analyzed frequency band and considered time
length. Therefore, target phenomenon are other than
in previous studies.
It is also worth noting that the methods are fairly
quick to run on current computers. Notice that the
HFO detection is slightly demanding in terms of
computational complexity than the DFA technique.
So algorithms based on these methods may be
applied in real time, enhancing the practical
capabilities provided by a seizure detector.
REFERENCES
Andrade-Valenca, L. P. et al., 2011. Interictal scalp fast
oscillations as a marker of the seizure onset zone.
Neurology, v. 77, n. 6, p. 524–3.
Andrade-Valenca, L. et al., 2012. Interictal high frequency
oscillations (HFOs) in patients with focal epilepsy and
normal MRI. Clinical neurophysiology : official
journal of the International Federation of Clinical
Neurophysiology, v. 123, n. 1,p. 100–5.
Blesic, S. et al., 2005. Detecting long-range correlations in
time series of dorsal horn neuron discharges. Annals of
the New York Academy of Sciences, v. 1048, p. 385–
91.
Bragin, a. et al., 1999. Hippocampal and entorhinal cortex
high-frequency oscillations (100–500 Hz) in human
epileptic brain and in kainic acid–treated rats with
chronic seizures. Epilepsia, v. 40, n. 2, p. 127–37.
Brodie, M. J., 2005. Diagnosing and predicting refractory
epilepsy. Acta neurologica Scandinavica.
Supplementum, v. 181, p. 36–9.
Buzsáki, G.; SILVA, F. L. da, 2012. High frequency
oscillations in the intact brain. Progress in
neurobiology, v. 98, n. 3, p. 241–9.
Chen, Z. et al., 2002. Effect of nonstationarities on
detrended fluctuation analysis. Physical review. E,
Statistical, nonlinear, and soft matter physics, v. 65, n.
4 Pt 1, p. 04110.
Ellenrieder, N. von et al., 2012. Automatic detection of
fast oscillations (40-200Hz) in scalp EEG recordings.
Clinical neurophysiology : official journal of the
International Federation of Clinical Neurophysiology,
v. 123, n. 4, p. 670–80.
Engel, J.; Silva, F. L. da, 2012. High-frequency
oscillations - where we are and where we need to go.
Progress in neurobiology, v. 98, n. 3, p. 316–8.
Fisher, R. S. et al., 2005. Epileptic seizures and epilepsy:
definitions proposed by the International League
Against Epilepsy (ILAE) and the International Bureau
for Epilepsy (IBE). Epilepsia, v. 46, n. 4, p. 470–2.
Goldberger, A. L. et al., 2000. PhysioBank, PhysioToolkit,
and PhysioNet: Components of a New Research
Resource for Complex Physiologic Signals.
Circulation, v. 101, n. 23, p. e215–e220.
Jacobs, J. et al., 2012. High-frequency oscillations (HFOs)
in clinical epilepsy. Progress in neurobiology, v. 98, n.
3, p. 302–15.
Lachowycz, S. M. et al., 2013. Long-range correlations
identified in time-series of volcano seismicity during
dome-forming eruptions using detrended fluctuation
analysis. Journal of Volcanology and Geothermal
Research, Elsevier B.V., v. 264, p. 197–209.
NEUROTECHNIX2014-InternationalCongressonNeurotechnology,ElectronicsandInformatics
104