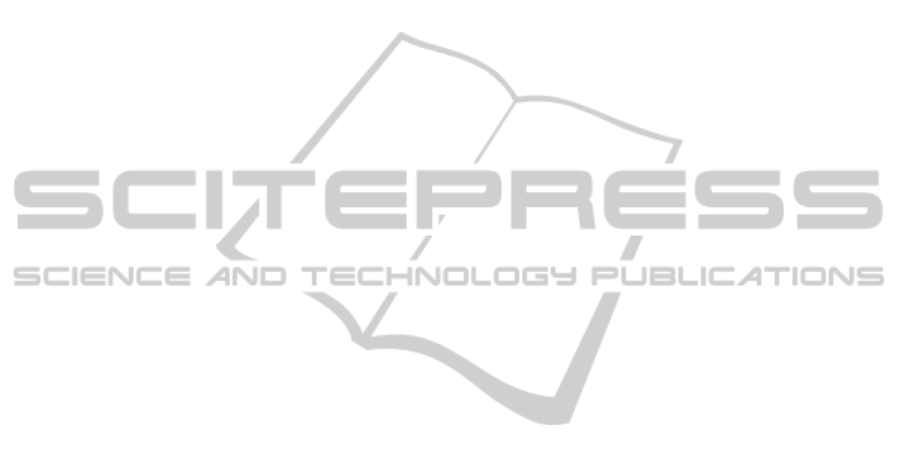
Considering the time required for an evasive ma-
neuver,it is even more important to detect the obstacle
at an early stage. The standard environmental vision
system could recognize the vehicle when it overshot
the threshold by 55 mm. In Figure 7, this threshold
is indicated with a white line drawn 800 mm from the
obstacle. In contrast, the proposed system could rec-
ognize the vehicle just after it reached the line.
When a collision occurred in the case of the stan-
dard environmental vision system, the vehicle had a
velocity of 7.2 kilometers per hour (km/h) (=1.8 mil-
limeters per millisecond (mm/ms)). Therefore, the
delay for recognition in the standard vision system
can be estimated to be 55/1.8 ≃ 30.5 ms. For this
reason, collision avoidance fails with the standard vi-
sion system, whereas it succeeds with the proposed
system.
In this experiment, the vehicle started the evasive
maneuver when the distance from the vehicle to the
obstacle fell below 800 mm. This distance is equiva-
lent to 8 m at actual scale. It is known that vehicles
must maintain at least 58 m intervals between them
for safe driving, which is around 7 times longer than
8 m.
6 CONCLUSION AND FUTURE
WORK
In this research, we aim to construct a driving safety
support system based on networked high-speed vision
cameras. We constructed a system employing two
high-speed environmental cameras attached to work-
stations, which were connected via a network and
synchronized to sub-millisecond order, and a com-
munication station. We also conducted comparative
experiments of collision avoidance. One experiment
using standard cameras (30 fps) failed to avoid a col-
lision with an obstacle. In contrast, the other experi-
ment using high-speed cameras (600 fps) succeeded
in avoiding a collision with the obstacle. Through
fundamental experiments, we demonstrated the effec-
tiveness of the proposed system when applied to a
driving safety support system and showed that such
a system can overcome the low responsiveness that is
common in standard environmental vision systems.
To further reinforce the effectiveness of the pro-
posed system, we are planning to carry out additional
experiments to compare it with an onboard vision sys-
tem for collision avoidance. We also aim to introduce
the proposed system in other situations. For instance,
it should be possible to apply it to intersections in ur-
ban areas. Although vehicles generally drive at lower
speeds in urban areas, the speed relative to vehicles
driving in the opposite direction is twice as fast as
that of the vehicle in question. We expect that our
proposed system will be effective even in such situa-
tions where the driving speed is not high.
ACKNOWLEDGEMENTS
This work was supported in part by the Strategic In-
formation and Communications R&D Promotion Pro-
gramme (SCOPE) 121803013.
REFERENCES
Cherng, S., Fang, C. Y., Chen, C. P., and Chen, S. W.
(2009). Critical motion detection of nearby moving
vehicles in a vision-based driver-assistance system. In
IEEE Transactions on Intelligent Transportation Sys-
tems, volume 10, pages 70–82.
Ishii, I. and Ishikawa, M. (1999). Self windowing for high-
speed vision. In IEEE International Conference on
Robotics and Automation, pages 1916–1921.
Keller, C. G., Dang, T., Fritz, H., Joos, A., Rabe, C., and
Gavrila, D. M. (2011). Active pedestrian safety by au-
tomatic braking and evasive steering. In IEEE Trans-
actions on Intelligent Transportation Systems, pages
1292–1304.
Kim, J. and Kim, J. (2009). Intersection collision avoid-
ance using wireless sensor network. In IEEE In-
ternational Conference on Vehicular Electronics and
Safety, pages 68–73.
Noda, A., Hirano, M., Yamakawa, Y., and Ishikawa, M.
(2014). A networked high-speed vision system for
vehicle tracking. In Sensors Applications Symposium,
pages 343–348.
Noda, A., Yamakawa, Y., and Ishikawa, M. (2013). High-
speed object tracking across multiple networked cam-
eras. In IEEE/SICE International Symposium on Sys-
tem Integration, pages 913–918.
Papadimitratos, P. and Evenssen, K. (2009). Vehicular com-
munication systems: Enabling technologies, applica-
tions, and future outlook on intelligent transportation.
In IEEE Communication Magazine, volume 47, pages
84–95.
Vceraraghavan, H., Masoud, O., and Papanikolopoulos, N.
(2002). Vision-based monitoring of intersections. In
IEEE International Conference on Intelligent Trans-
portation Systems, pages 7–12.
ZMP (2014). 1/10 scale robocar 1/10. http://www.zmp.
co.jp/wp/products/robocar-110?lang=en.
ICINCO2014-11thInternationalConferenceonInformaticsinControl,AutomationandRobotics
544