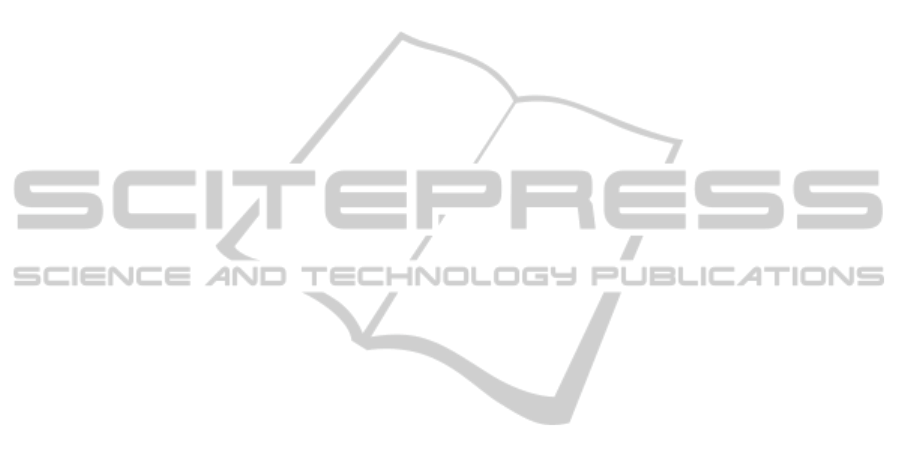
5 CONCLUSIONS
In this paper, we propose an alternative approach to
the challenging problem of human emotion
recognition based on brain data. In contrast to most
of the recognition systems where the best spatial-
temporal features are searched, we consider
separately the selection of spatial features (the
channels) and the selection of temporal features
(amplitudes/latencies) in order to distinguish the
processing of stimuli with positive and negative
emotion valence based on ERPs observations. The
core of the present study is to explore the feasibility
of training cross-subject classifiers to make
predictions across multiple human subjects. The
choice of the occipital/parietal channels (more
particularly channel Oz and P7) or the choice of the
temporal features related with the latencies of the
amplitude peaks over all channel (L
max2
,L
min3
,L
max3
)
has the potential to reduce the inter-subject
variability and improve the learning of
representative models valid across multiple subjects.
However, before making stronger conclusions on
the capacity of i) single channel or ii) single feature
over all channels classification models to decode
emotions, further research is required to answer
more challenging questions such as discrimination
of more than two emotions. In fact this is a valid
question for all reported works on affective
neuroscience (Calvo, 2010), (Hidalgo-Muñoz,
2013), (Hidalgo-Muñoz, 2014). The discrimination
is usually limited to two, three, and maximum four
valence-arousal emotional classes. Interesting
problem is also the human personality classification
based on EEG, for example high versus low neurotic
type of personality.
Also, the number of the participants in the
experiments is important for revealing stable cross
subject features. In the reviewed references the
average number of participants is about 10-15, the
maximum is 32. We need publicly available datasets
to compare different techniques and thus speed up
the progress of affective computing.
ACKNOWLEDGEMENTS
We would like to express thanks to the PsyLab from
Departamento de Educação da UA, and particularly
to Dr. Isabel Santos, for providing the data sets.
REFERENCES
Calvo, R. A. & D’Mello, S. K. (2010). Affect Detection:
An Interdisciplinary Review of Models, Methods, and
their Applications. IEEE Transactions on Affective
Computing, 1(1), 18-37.
T. Dalgleish, B. Dunn and D. Mobbs "Affective
Neuroscience: Past, Present, and Future", Emotion
Rev., vol. 1, pp.355 -368 2009.
J. K. Olofsson, S. Nordin , H. Sequeira and J. Polich
"Affective Picture Processing: An Integrative Review
of ERP Findings", Biological Psychology, vol. 77,
pp.247 -265 2008.
O. AlZoubi, R. A. Calvo and R. H. Stevens
"Classification of EEG for Emotion Recognition: An
Adaptive Approach", Proc. 22nd Australasian Joint
Conf. Artificial Intelligence, pp.52 -61 2009.
P. C. Petrantonakis and L. J. Hadjileontiadis "Emotion
Recognition from EEC Using Higher Order
Crossings", IEEE Trans. Information Technology in
Biomedicine, vol. 14, no. 2, pp.186 -194 2010.
N. Jatupaiboon, S. Panngum, P. Israsena, Real-Time EEG-
Based Happiness Detection System, The
ScientificWorld Journal, Vol. 2013, Article ID 18649,
12 pages.
Santos, I. M., Iglesias, J., Olivares, E. I. & Young, A. W.
(2008). Differential effects of object-based attention
on evoked potentials to fearful and disgusted faces.
Neuropsychologia, 46, 1468-1479.
Pourtois, G., Grandjean, D., Sander, D., & Vuilleumier, P.
(2004). Electrophysiological correlates of rapid spatial
orienting towards fearful faces. Cerebral Cortex,
14(6), 619–633.
Christopher M. Bishop (2006). Pattern Recognition and
Machine Learning. Springer.
Fisher, R. A. The Use of Multiple Measurements in
Taxonomic Problems. Annals of Eugenics, Vol. 7, pp.
179–188, 1936.
O. Georgieva, S. Milanov, P. Georgieva, I.M. Santos,
A.T.Pereira, C. F. da Silva (2014), Learning to decode
human emotions from ERPs, Neural Computing and
Applications, Springer (in press).
Bozhkov L., P. Georgieva, R. Trifonov, Brain Neural Data
Analysis Using Machine Learning Feature Selection
and Classification Methods. 15th Int. Conf. on
Engineering Applications of Neural Networks
(EANN'14) 5-7 Sept. 2014, Sofia, Bulgaria.
(accepted).
A. R. Hidalgo-Muñoz, M. L. Pérez, A. Galvao-Carmona,
A. T. Pereira, I. M. Santos, M. Vázquez-Marrufo, Ana
Maria Tomé. EEG study on affective valence elicited
by novel and familiar pictures using ERD/ERS and
SVM-RFE. Medical & Biological Engineering &
Computing, 52(2), 149-158, 2014.
A. R. Hidalgo-Muñoz, M. L. Pérez, I.M. Santos, A.T.
Pereira, M. Vázquez-Marrufo, A. Galvao-Carmona,
Ana Maria Tomé. Application of SVM-RFE on EEG
signals for detecting the most relevant scalp regions
linked to affective valence processing. Expert Systems
with Applications, 40 (6) , 2102-2108, 2013.
SIMULTECH2014-4thInternationalConferenceonSimulationandModelingMethodologies,Technologiesand
Applications
606