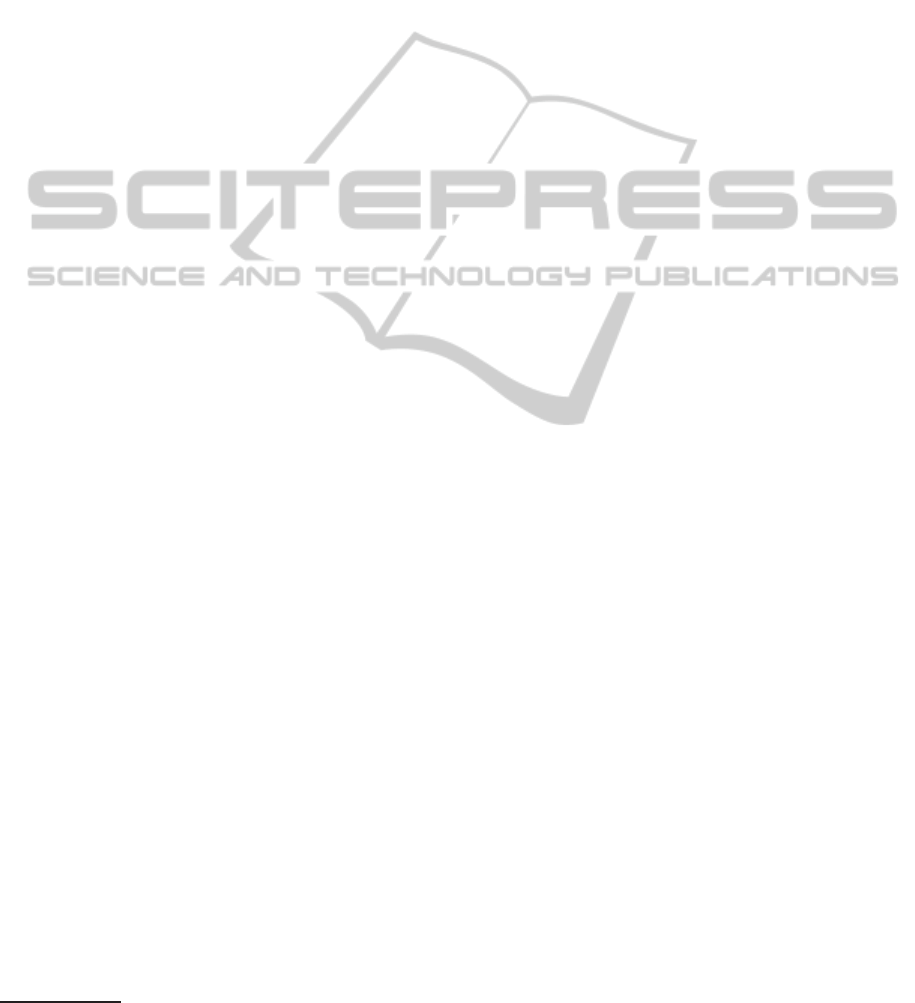
Free Data. We also investigate the case, where the
data provider offers processed information for free –
this is similar to the current practice where the real-
time traffic information is offered at no cost. Inter-
estingly, in that case, the private firm has less incen-
tive to increase his quality. In other words, the pri-
vate firm provides lower quality compare to the case
that the data provider prices his data. This, in turn,
decreases social welfare. We conjecture that with
free processed information, the data provider needs
to ignore his profit to maximize social welfare. Pre-
cisely, when the data provider does not price his data,
welfare-maximizing β is exactly one.
4 FUTURE DIRECTIONS
In addition to completing our analysis for the afore-
mentioned monopoly and duopoly, we would like to
study other pricing strategies in data markets. A
natural future step is to compare subscription and
consumption-based pricing schemes similar to those
currently used in cloud computing, for instance by
Amazon’s EC2 platform.
12
In a consumption-based
pricing model, customers pay according to the re-
sources used. The resource can be the amount of data
they acquire. However, in subscription-based pricing
models, customers commit to the service for specified
periods of time and pay a flat fee for that period.
ACKNOWLEDGEMENTS
We would like to thank Cyrus Shahabi and Ugur
Demiryurek for inspiring discussions. This work is
supported by a grant from Integrated Media System
Center at USC.
REFERENCES
ADMS (2009). Adms smart travel lab. Available at
http://cts.virginia.edu/stl-adms.htm/.
Delbono, F. (1991). Quality choice in a vertically differeiti-
ated mixed duopo1y.
Demiryurek, U., Banaei-Kashani, F., Shahabi, C., and Ran-
ganathan, A. (2011). Online computation of fastest
path in time-dependent spatial networks. In Advances
in Spatial and Temporal Databases, pages 92–111.
Springer.
Furrier, J. (2012). Big data is creating the future - it’s a $50
billion market. Forbes.
12
http://aws.amazon.com/ec2/pricing/.
Gehrke, J. D. and Wojtusiak, J. (2008). Traffic prediction
for agent route planning. In Computational Science–
ICCS 2008, pages 692–701. Springer.
Harmon, R., Demirkan, H., Hefley, B., and Auseklis, N.
(2009). Pricing strategies for information technology
services: A value-based approach. In System Sciences,
2009. HICSS’09. 42nd Hawaii International Confer-
ence on, pages 1–10. IEEE.
Ishibashi, K. and Kaneko, T. (2008). Partial privatization
in mixed duopoly with price and quality competition.
Journal of Economics, 95(3):213–231.
Klein, L. A. (2001). Sensor technologies and data require-
ments for ITS.
Knaian, A. N. (2000). A wireless sensor network for smart
roadbeds and intelligent transportation systems. PhD
thesis, Massachusetts Institute of Technology.
Levin, J. (2003). Supermodular games. Lectures Notes,
Department of Economics, Stanford University.
Lohr, S. (2011). New ways to exploit raw data may bring
surge of innovation, a study says. The New York Times.
Available at http://www.nytimes.com/2011/05/13/
technology/13data.html.
Muschalle, A., Stahl, F., L¨oser, A., and Vossen, G.
(2013). Pricing approaches for data markets. In En-
abling Real-Time Business Intelligence, pages 129–
144. Springer.
Pan, B., Demiryurek, U., and Shahabi, C. (2012). Utiliz-
ing real-world transportation data for accurate traffic
prediction. In ICDM, pages 595–604.
Park, B., Messer, C. J., and Urbanik II, T. (1998). Short-
term freeway traffic volume forecasting using radial
basis function neural network. Transportation Re-
search Record: Journal of the Transportation Re-
search Board, 1651(1):39–47.
Schomm, F., Stahl, F., and Vossen, G. (2013). Marketplaces
for data: an initial survey. ACM SIGMOD Record,
42(1):15–26.
Shapiro, C., Varian, H. R., and Becker, W. (1999). Informa-
tion rules: a strategic guide to the network economy.
Journal of Economic Education, 30:189–190.
Tubaishat, M., Zhuang, P., Qi, Q., and Shang, Y. (2009).
Wireless sensor networks in intelligent transportation
systems. Wireless communications and mobile com-
puting, 9(3):287–302.
Williams, B. M., Durvasula, P. K., and Brown, D. E. (1998).
Urban freeway traffic flow prediction: application
of seasonal autoregressive integrated moving average
and exponential smoothing models. Transportation
Research Record: Journal of the Transportation Re-
search Board, 1644(1):132–141.
Yuan, J., Zheng, Y., Xie, X., and Sun, G. (2011). Driving
with knowledge from the physical world. In Proceed-
ings of the 17th ACM SIGKDD international confer-
ence on Knowledge discovery and data mining, pages
316–324. ACM.
DATA2014-3rdInternationalConferenceonDataManagementTechnologiesandApplications
270