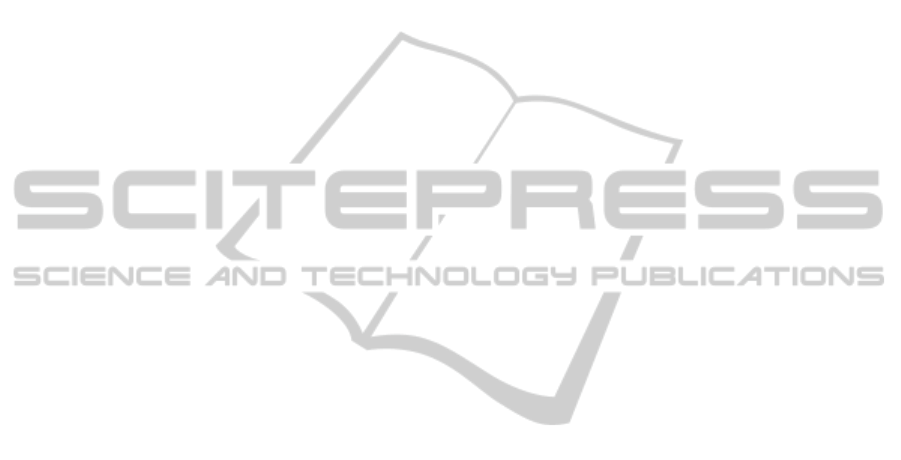
ACKNOWLEDGEMENTS
Authors thank the Slovenian Ministry of Higher
Education, Science and Technology (grant P1-017)
and 3ZEN d.o.o. for experimental work.
REFERENCES
Changyu, S., Lixia, W., Qian, L., 2007. Optimization of
injection molding process parameters using
combination of artificial neural network and genetic
algorithm method, J. Mater. Process. Technol.,
vol.183 (2), pp. 412–418.
Cook, D. F., Ragsdale, C. T., Major, R. L., 2000.
Combining a neural network with a genetic algorithm
for process parameter optimization, Eng. Appl. Artif.
Intell., vol. 13, issue 4, pp.391-396.
Daszykowski, M., Walczak, B., Massart, D. L., 2003. A
journey into low-dimensional spaces with
Autoassociative Neural Networks, Talanta, vol. 59 no.
6, pp. 1095-1105.
Daszykowski, M., Walczak, B., Massart, D. L., 2003.
Projection methods in chemistry, Chemom. Intell. Lab.
Syst., vol. 65, pp. 97-112.
Davies, O. L., 1956. Design and Analysis of Industrial
Experiments, Imperial Chemical Industries. London.
Douglas, C. Montgomery, 2012. "Design and Analysis of
Experiments, JOHN WILLEY & SONS, INC.New
York (NY), 8th Edition.
Eriksson, L., Johansson, E., Kettaneh-Wold N., et al.,
2008. Design of Experiments: Principles and
Applications, UMETRICS AB. Sweden, 3-d ed.
Hamdy, M., Hasan, A., Siren, K., 2011. Applying a multi-
objective optimization approach for Design of low-
emission cost-effective dwellings. Build. Environ.,
vol. 46, issue 1, pp.109-123.
Jin, Y., 2011. Surrogate-assisted evolutionary
computation, Recent advances and future challenges.
Swarm and Evolutionary Computation, vol.1(2), pp.
61–70.
Kramer, M. A., 1991. Nonlinear principal component
analysis using autoassociative neural networks, AIChE
J, vol. 37, no. 2, pp. 233–243.
Livingstone, D. J., Hesketh, G., Clayworth, D., 1991.
Novel methods for the display of.
multivariate data using neural networks, J. Mol. Graphics,
vol. 9, pp. 115–118.
Loshchilov, I., Schoenauer, M., Sebag, M., 2010.
Comparison-Based Optimizers Need Comparison-
Based Surrogates. Parallel Problem Solving from
Nature (PPSN XI). Springer, vol. 6238, pp. 364–373.
Natrella, M. G., 1963. Experimental Statistics. National
Bureau of Standards Handbook 91, John Wiley and
Sons. Inc.Washington.
Ozcelik, B., Erzurumlu, T., 2006. Comparison of the
warpage optimization in the plastic injection molding
using ANOVA, neural network model and genetic
algorithm, J. Mater. Process. Technol., vol. 171, issue
3, pp. 437–445.
Park, Y. W., Rhee, S., 2008. Process modeling and
parameter optimization using neural network and
genetic algorithms for aluminum laser welding
automation, The Int. J. Adv. Manuf. Technol., vvol. 37,
issue 9-10, pp. 1014-1021.
Pishvaee, M., Rabbani, M., Torabi, S., 2011. A robust
optimization approach to closed-loop supply chain
network design under uncertainty. Appl. Math.
Modell., vol. 35, issue 2, pp. 637-649.
Sette, S., Boullart, L., et al., 1997. Optimizing the Fiber-
to-Yarn Production Process with a Combined Neural
Network/Genetic Algorithm Approach, Text. Res. J.,
vol. 67 (2), pp. 84-92.
Wu, A., Zhang, J., Chung, H., 2011. Decoupled optimal
design for power electronic circuits with adaptive
migration in coevolutionary environment. Appl. Soft
Comput. J., vol. 11, issue 1, pp. 23-31.
Wang, J., Zhai, Z. J., Jing,Y., et al., 2010. Particle swarm
optimization for redundant building cooling heating
and power system. Appl. Energy, vol. 87, issue 12, pp.
3668-3679.
Zheng, J., Wang, Q., Zhao, P., et al., 2009. Optimization
of high-pressure die-casting process parameters using
artificial neural network, International journal,
advanced manufacturing technology, vol. 44(7-8), pp.
667-674.
SIMULTECH2014-4thInternationalConferenceonSimulationandModelingMethodologies,Technologiesand
Applications
766