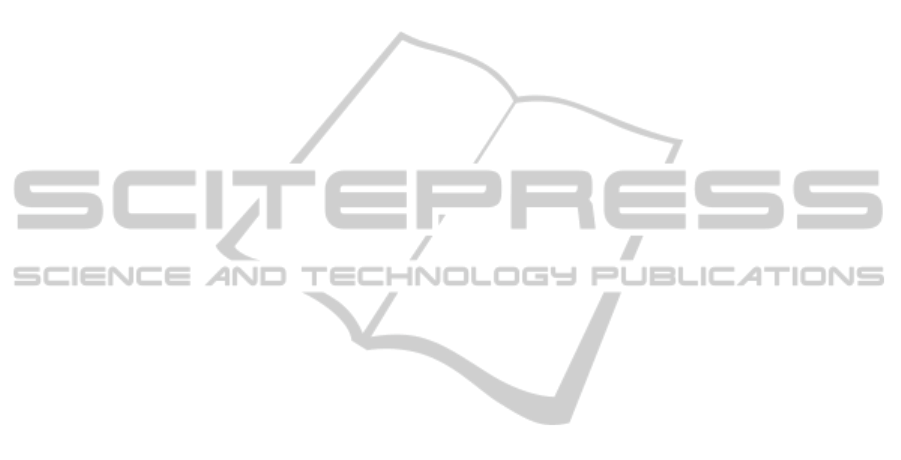
CI at a less-biased point. Sectioning also outperforms
FD in terms of coverage. Comparing FD and Exact
for p = 0.99 and small n, we see that the AHW of
FD typically is quite different from AHW for Exact,
which indicates that in these cases, FD does a poor
job estimating λ, resulting in FD’s poor coverage.
Relative to SRS, CMC reduces the AHW about
60% (resp., 70% and 80%) for p = 0.8 (resp., p =
0.95 and p = 0.99). Thus, the variance reduction from
CMC improves as we consider more extreme quan-
tiles. For each of the smaller values of n, the cov-
erage for each SRS CI (except Exact) worsens as p
increases. While CMC coverage also degrades some-
what as p approaches 1, the impact is much less pro-
nounced. Also, for large n, the slightly wider AHW
for batching and sectioning compared to Exact and
FD arises because the former two methods are based
on a Student t limit, whereas the latter two rely on a
normal limit, which has lighter tails.
5 CONCLUSIONS
We developed an estimator of a quantile ξ using con-
ditional Monte Carlo, which is guaranteed to reduce
asymptotic variance compared to simple random sam-
pling. We established that the CMC quantile estima-
tor satisfies a weak Bahadur representation, which im-
plies a CLT holds. We used these results to produce
three asymptotically valid confidence intervals for ξ
as the sample size n →∞. The CIs are based on batch-
ing, sectioning and a finite difference. Our numerical
results seem to indicate that compared to SRS, CMC
not only reduces variance, but it also leads to CIs with
better coverage. For both SRS and CMC, the sec-
tioning CI has better coverage than the batching and
finite-difference intervals for small n, especially when
p ≈ 1. Thus, of the three CIs we proposed, we recom-
mend using sectioning.
ACKNOWLEDGEMENTS
This work has been supported in part by the Na-
tional Science Foundation under Grants No. CMMI-
0926949, CMMI-1200065, and DMS-1331010. Any
opinions, findings, and conclusions or recommenda-
tions expressed in this material are those of the author
and do not necessarily reflect the views of the Na-
tional Science Foundation.
REFERENCES
Asmussen, S. and Glynn, P. (2007). Stochastic Simulation:
Algorithms and Analysis. Springer, New York.
Bahadur, R. R. (1966). A note on quantiles in large samples.
Annals of Mathematical Statistics, 37:577–580.
Billingsley, P. (1995). Probability and Measure. John Wiley
& Sons, New York, third edition.
Bloch, D. A. and Gastwirth, J. L. (1968). On a simple esti-
mate of the reciprocal of the density function. Annals
of Mathematical Statistics, 39:1083–1085.
Bofinger, E. (1975). Estimation of a density function using
order statistics. Australian Journal of Statistics, 17:1–
7.
Chu, F. and Nakayama, M. K. (2012). Confidence intervals
for quantiles when applying variance-reduction tech-
niques. ACM Transactions On Modeling and Com-
puter Simulation, 36:Article 7 (25 pages plus 12–page
online–only appendix).
Falk, M. (1986). On the estimation of the quantile density
function. Statistics & Probability Letters, 4:69–73.
Fu, M. C., Hong, L. J., and Hu, J.-Q. (2009). Conditional
Monte Carlo estimation of quantile sensitivities. Man-
agement Science, 55:2019–2027.
Ghosh, J. K. (1971). A new proof of the Bahadur repre-
sentation of quantiles and an application. Annals of
Mathematical Statistics, 42:1957–1961.
Jorion, P. (2007). Value at Risk: The New Benchmark for
Managing Financial Risk, 3rd Edition. McGraw-Hill.
Mood, A. M., Graybill, F. A., and Boes, D. C. (1974). In-
troduction to the Theory of Statistics. McGraw-Hill,
New York, 3rd edition.
Nakayama, M. K. (2014a). Confidence intervals us-
ing sectioning for quantiles when applying variance-
reduction techniques. ACM Transactions on Modeling
and Computer Simulation. To appear.
Nakayama, M. K. (2014b). Efficient quantile estimation
using conditional Monte Carlo. In preparation.
Ortega, J. M. and Rheinboldt, W. C. (1987). terative So-
lution of Nonlinear Equations in Several Variables.
SIAM.
Ross, S. M. (2006). Simulation. Academic Press, San
Diego, CA, fourth edition.
Serfling, R. J. (1980). Approximation Theorems of Mathe-
matical Statistics. John Wiley & Sons, New York.
U.S. Nuclear Regulatory Commission (1989). Best-
estimate calculations of emergency core cooling per-
formance. Nuclear Regulatory Commission Regula-
tory Guide 1.157, U.S. Nuclear Regulatory Commis-
sion, Washington, DC.
U.S. Nuclear Regulatory Commission (2011). Applying
statistics. U.S. Nuclear Regulatory Commission Re-
port NUREG-1475, Rev 1, U.S. Nuclear Regulatory
Commission, Washington, DC.
QuantileEstimationWhenApplyingConditionalMonteCarlo
285